TL;DR:
- A team of researchers, led by experts from The University of Tokyo, has applied deep learning to accelerate supernova simulations, published in the “Monthly Notices of the Royal Astronomical Society.”
- This innovative approach aims to enhance our understanding of galaxy formation and evolution by speeding up simulations, including the chemistry leading to life.
- Deep learning, previously successful in weather forecasting, inspired the application to supernova explosions.
- Current simulations often oversimplify supernovae as perfectly spherical, whereas deep learning models seek to account for their asymmetry.
- The 3D-MIM model reduces computational steps by 99%, promising to alleviate bottlenecks in supernova research.
- The team’s success opens the door to applying this methodology in other astrophysical areas and fields requiring high-resolution simulations.
Main AI News:
In the vast cosmos, the role of supernovae, those awe-inspiring exploding stars, is nothing short of pivotal in the grand tapestry of galactic formation and evolution. Yet, these celestial phenomena have long stymied scientists with their elusive complexities, demanding an inordinate amount of computational effort and time for faithful simulations.
Breaking new ground in the realm of astrophysics, a consortium of researchers, prominently featuring scholars from The University of Tokyo, has harnessed the formidable power of deep learning to tackle the enigma of supernova simulations head-on. This groundbreaking endeavor finds its prestigious place within the pages of the esteemed journal, “Monthly Notices of the Royal Astronomical Society.”
Their innovative approach holds the promise of revolutionizing supernova simulations, thereby expediting our comprehension of galaxy formation and evolution. Crucially, these simulations encompass the intricate chemical processes that ultimately gave rise to life itself.
Deep learning, a stalwart in contemporary research, has recently ventured into the realm of meteorology, leaving a lasting impact. At a notable tech event referred to as a “hackathon,” a team demonstrated the formidable prowess of deep learning in the arena of weather forecasting. The results were nothing short of remarkable, igniting a spark of curiosity within Keiya Hirashima, a doctoral student at the University of Tokyo’s Department of Astronomy.
Hirashima elucidated, “Weather, despite its inherent complexity, fundamentally boils down to fluid dynamics calculations. This realization prompted me to ponder the feasibility of adapting deep learning models, originally designed for meteorological predictions, to another fluid system—one operating on an incomprehensibly grander scale, beyond our direct reach: the domain of supernova explosions, the focus of my research.”
Supernovae materialize when colossal celestial bodies exhaust their fuel and succumb to cataclysmic detonations. These cosmic juggernauts wield such immense influence that their fiery throes reverberate across the expanse of their host galaxies. Had a supernova erupted within a few hundred light-years of Earth several centuries ago, our very interaction might have been a missed encounter. Consequently, the deeper our understanding of supernovae, the more profound our comprehension of the intricacies that shape galaxies.
Hirashima elucidated the crux of the issue, “The bottleneck in our pursuit of understanding lies in the protracted duration required for calculating the intricacies of supernova explosions. Presently, many galaxy models spanning eons opt for a simplification: they envision supernovae as perfectly spherical eruptions, an easily calculable scenario.”
“However,” he continued, “in reality, supernovae unfold in asymmetric splendor. Distinct regions of the explosive shell possess varying complexities. By harnessing deep learning, we’ve embarked on the quest to identify which facets of the explosion warrant heightened scrutiny and which can be expedited to achieve optimal accuracy within the shortest computational timeframe. This paradigm, known as Hamiltonian splitting, has given birth to our cutting-edge model, 3D-MIM. It achieves a remarkable 99% reduction in computational steps required for simulating 100,000 years of supernova evolution. We’re confident that this breakthrough will significantly alleviate the existing computational bottleneck.”
However, the journey of deep learning is one paved with rigorous training. Hirashima and his team embarked on a marathon of simulations, consuming millions of hours of computing time. Supercomputers, thanks to their parallel architecture, enabled the efficient division of this time among thousands of processing elements. The toil was demanding, yet the outcomes were unequivocally rewarding.
Now, with their triumph over the intricacies of supernovae, these visionary researchers aspire to extend the boundaries of their methodology to other frontiers within astrophysics. Galactic evolution, sculpted by expansive star-forming regions, beckons for further exploration. 3D-MIM, with its adept modeling of stellar demises, may soon be pressed into service to unveil the very births of these cosmic giants. Beyond the realms of astrophysics, this innovation holds the potential to find applications in diverse fields that demand high spatial and temporal resolutions, from climate predictions to earthquake simulations.
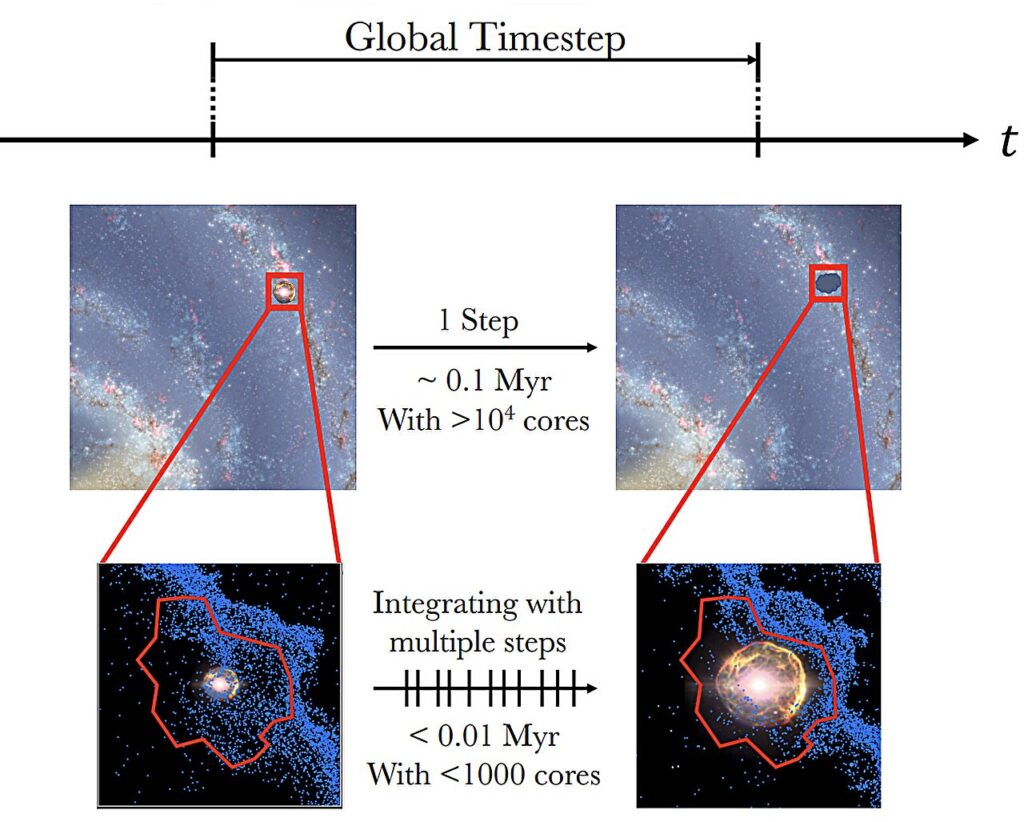
Source: Phys.org
Conclusion:
The application of deep learning to supernova simulations is a groundbreaking development in astrophysics. It promises to significantly accelerate our understanding of galaxy formation and evolution while reducing computational bottlenecks. This innovation not only enhances our grasp of the cosmos but also opens doors to high-resolution simulations in various fields, potentially impacting markets related to advanced computing and simulation technologies.