- Researchers develop machine learning-based emulator for predicting auroral current system in space weather.
- Previous physics-based models required supercomputers, hindering efficiency.
- The new emulator, SMRAI2, operates million times faster, incorporating seasonal effects.
- Solar weather forecasts are crucial for mitigating impacts on satellite communication and radiation exposure.
- Echo state network (ESN) was utilized to refine prediction accuracy.
- Integration of emulator into ensemble space weather forecast to enhance precision.
Main AI News:
The realm of space weather encompasses various levels of severity, from geomagnetic storms to solar radiation storms and radio blackouts. These phenomena unleash diverse impacts on Earth, from disrupting satellite and GPS functionalities to causing communication breakdowns and electrical grid malfunctions. Moreover, they pose health risks to astronauts and individuals aboard high-altitude flights. Among the marvels wrought by geomagnetic storms are the captivating auroras frequently witnessed in polar regions.
Recognizing the potential hazards posed by space weather events, researchers have endeavored to devise physics-based models for predicting the auroral current system, leveraging data on solar wind particles discharged from the sun. However, hitherto, executing such models has been laborious and resource-intensive, necessitating the utilization of entire supercomputers.
In a significant breakthrough, researchers have unveiled a machine learning-driven emulator capable of swiftly replicating physics-based simulations of the auroral current system, all while demanding considerably lesser computational resources. Their findings, elucidating this innovation, have been disseminated in the esteemed journal Space Weather.
“A physics-based simulation of the auroral current system is pivotal for space weather forecasting. Nevertheless, the execution of such simulations mandates dedicated supercomputing resources,” remarked Ryuho Kataoka, lead author of the study and associate professor at the National Institute of Polar Research and SOKENDAI, situated in Tachikawa, Japan.
Among the well-established models in this domain is REPPU (REProduce Plasma Universe), renowned for its reliability in reproducing the auroral current system. With the inception of the ’emulator,’ akin outcomes can now be attained by employing a conventional laptop PC.
Dubbed Surrogate Model for REPPU Auroral Ionosphere version 2 (SMRAI2), this novel emulator operates at speeds surpassing its physics-based counterparts by a staggering factor of one million, while also incorporating seasonal variations into its predictions.
While forecasts cannot mitigate the impacts of solar radiation and solar wind on Earth, they can serve to equip affected communities with preparedness measures against communication disruptions and radiation hazards for astronauts and high-altitude aircraft passengers.
Of particular concern are satellites, highly susceptible to perturbations induced by magnetic storms. A stark illustration of this vulnerability emerged in February 2022 when 38 commercial satellites succumbed to reentry into Earth’s atmosphere following a moderate magnetic storm. Such storms stem from the transfer of substantial energy from the solar wind to Earth’s magnetosphere.
To fashion the physics-based prediction model emulator, the research team deployed a time-dependent machine learning model dubbed echo state network (ESN). Notably, ESNs represent a specialized form of recurrent neural networks adept at handling sequential data.
Significantly refining an initial iteration, the team enhanced the ESN-based emulator, ver1.0, by training the new model, SMRAI2, with a magnitude more physics-based simulation outputs.
“The culmination of this endeavor, SMRAI2, stands as a pioneering instance of employing machine learning techniques to emulate the ionospheric output derived from physics-based global magnetohydrodynamic (MHD) simulations. As we amass additional MHD simulation data and leverage other cutting-edge machine learning models, we anticipate further advancements in prediction accuracy,” explained Kataoka. MHD simulations serve as a tool for elucidating the dynamics of the magnetosphere, delineating the interaction between solar wind and Earth’s magnetic field.
The ensuing objective for the research cohort is to seamlessly integrate the emulator into the ensemble space weather forecast, comprising a suite of forecasts presenting a spectrum of future space weather scenarios. Ultimately, they aspire to harness the emulator in conjunction with an array of observational datasets within a data-assimilation forecast framework, thereby synergizing model outputs and observations to bolster prediction precision.
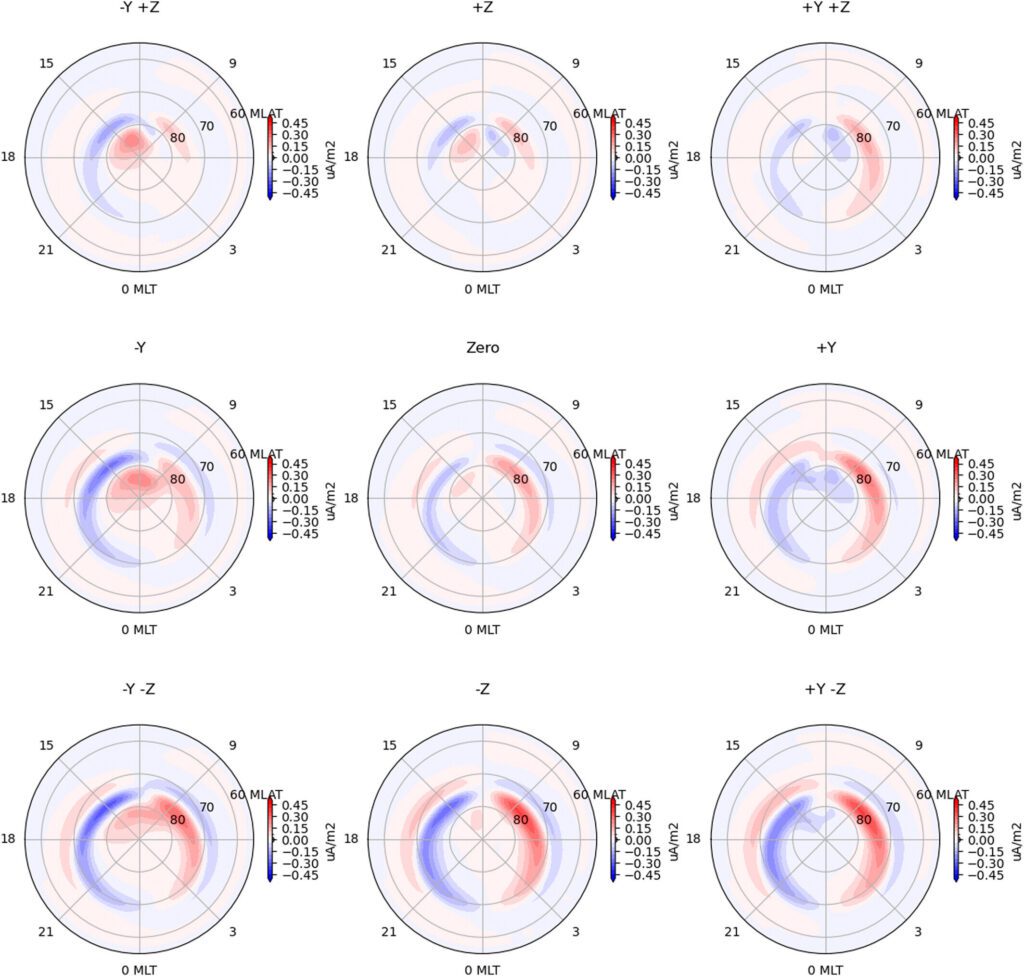
Steady state conditions of the auroral current system pattern in the Arctic region (polar view), which varies with the direction of the magnetic field of the solar wind, can be reproduced by SMRAI2 almost perfectly. Red and blue represent earthward (downward) and upward currents, respectively; Y and Z represent the direction of the solar wind magnetic field, with Z positive northward in the north-south direction and Y positive westward in the east-west direction. For example, ‘Zero’ indicates no Y and Z components of the solar wind magnetic field, ‘-Z’ indicates a complete south orientation, and ‘-Y -Z’ indicates a southeast orientation. Source: Space Weather (2024).
Conclusion:
The development of a machine learning-driven emulator for space weather forecasting marks a significant advancement in the field. This innovation not only improves prediction speed and accuracy but also enables better preparedness against potential disruptions to satellite communication and increased radiation exposure. As this technology continues to evolve, it presents lucrative opportunities for businesses involved in satellite communication, aerospace, and space exploration, allowing them to better anticipate and mitigate the effects of space weather events on their operations.