TL;DR:
- The HUB framework revolutionizes the integration of human feedback in Reinforcement Learning.
- It excels in Reward Learning from Human Feedback (RLHF), especially in scenarios involving multiple teachers.
- HUB streamlines teacher selection and enhances overall RLHF system performance.
- The framework actively queries teachers, providing deeper insights into utility functions.
- Operates as a Partially Observable Markov Decision Process (POMDP), optimizing both teacher selection and learning objectives.
- HUB’s practical applicability shines in real-world domains such as paper recommendations and COVID-19 vaccine testing.
Main AI News:
In the realm of Reinforcement Learning (RL), the integration of human feedback has become an increasingly pivotal challenge. This challenge is amplified when we delve into the intricate domain of Reward Learning from Human Feedback (RLHF), especially when multiple teachers are involved. The complexity of selecting the right teachers for RLHF systems has prompted the introduction of the groundbreaking HUB (Human-in-the-Loop with Unknown Beta) framework. This innovation is set to streamline teacher selection and elevate the overall performance of RLHF systems.
Existing methods in RLHF have grappled with the intricacies of managing utility functions efficiently. This limitation underscores the need for a more sophisticated and holistic approach to teacher selection. The HUB framework rises to meet this challenge by offering a structured and systematic method for appointing teachers within the RLHF paradigm. What sets it apart is its proactive approach to querying teachers, enabling a deeper exploration of utility functions and delivering refined estimations, even in the face of complex scenarios involving multiple educators.
At its core, the HUB framework functions as a Partially Observable Markov Decision Process (POMDP), seamlessly integrating teacher selection with the optimization of learning objectives. This integration not only streamlines the selection process but also fine-tunes the learning objectives themselves. The secret to its success lies in the active engagement with teachers, leading to a more nuanced comprehension of utility functions and, consequently, bolstering the precision of utility function estimation. By incorporating this POMDP-based approach, the HUB framework skillfully navigates the intricacies of learning utility functions from multiple teachers, ultimately enhancing accuracy and performance.
The true power of the HUB framework reveals itself in its real-world applicability across diverse domains. Rigorous evaluations in areas like paper recommendations and COVID-19 vaccine testing highlight its adaptability and practical significance. In the realm of paper recommendations, the framework’s ability to optimize learning outcomes demonstrates its relevance in information retrieval systems. Similarly, its successful deployment in COVID-19 vaccine testing underscores its potential to address urgent and multifaceted challenges, making substantial contributions to healthcare and public health advancements.
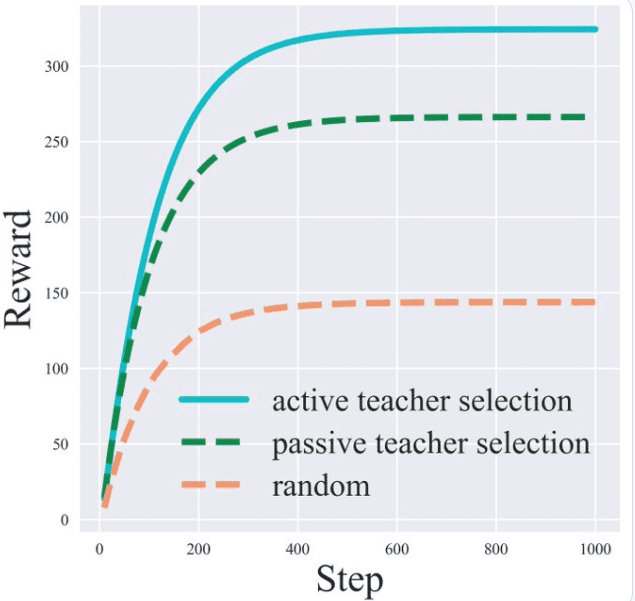
Source: Marktechpost Media Inc.
Conclusion:
The introduction of the HUB framework represents a significant leap in the field of Reinforcement Learning, particularly in the context of RLHF with multiple teachers. Its ability to streamline teacher selection and improve learning outcomes offers promising opportunities for businesses and industries that rely on AI-driven decision-making. The HUB framework’s adaptability across diverse domains positions it as a valuable asset for information retrieval systems, healthcare, and public health advancements, potentially transforming how businesses approach complex decision-making processes.