TL;DR:
- Large vision language models (LVLMs) are a focal point in AI research.
- Current LVLMs show promise but have room for improvement in image perception.
- Challenges: deficiencies in vision vocabulary networks and high computational costs.
- LVLMs excel in CV and NLP tasks thanks to visionary vocabulary networks.
- Vary-toy, a refined LVLM, optimizes the vision vocabulary creation process.
- It incorporates object detection into the vocabulary network, enhancing adaptability.
- Vary-toy delivers impressive results on challenging benchmarks.
- Its compact size makes it accessible to researchers with limited resources.
- Public code release promotes collaboration and innovation in LVLM research.
Main AI News:
In the realm of artificial intelligence research, the spotlight has firmly shifted toward large vision language models (LVLMs) in the past year. These remarkable models have showcased exceptional performance across a multitude of tasks when approached from different angles. However, the realm of image perception within LVLMs still holds untapped potential, calling for enhancements in their perceptual abilities.
This quest for enhanced visual comprehension within LVLMs encounters two formidable challenges. First, the current vision vocabulary networks exhibit deficiencies that need to be addressed. Second, the computational demands associated with optimizing numerous parameters are undeniably high.
Notably, LVLMs have proven their prowess in the convergence of Computer Vision (CV) and Natural Language Processing (NLP), excelling in tasks like image captioning, Visual Question Answering (VQA), meme interpretation, and scene Optical Character Recognition (OCR). A significant contributor to their success lies in the visionary vocabulary networks, with CLIP being a prominent example. LVLMs predominantly employ two architectural paradigms: utilizing image tokens as prefixes or employing cross-attention mechanisms for feature fusion. Regardless of architectural choices, the upper limits of these models hinge on the efficiency of their vision vocabulary networks in encoding visual cues.
In response to these challenges, researchers have devised a pragmatic and efficient strategy to amplify the vision vocabulary of LVLMs. This entails training a novel visual vocabulary network with the assistance of a smaller auto-regressive model like OPT-125M. The resulting vocabulary is then merged with the existing one, culminating in the creation of a refined LVLM. However, this approach, while promising, has its limitations, including underutilized network capacity and substantial iteration costs when employing Vary-base with 7B LLM.
Enter Vary-toy, the brainchild of researchers at MEGVII Technology, aimed at mitigating these limitations. Vary-toy adheres to the same conceptual framework as its predecessor, Vary, but introduces refinements in the vision vocabulary creation process. Instead of treating natural images solely as negative samples, it incorporates object detection tasks into the vocabulary network. This ingenious fusion combines rich textual data in PDF format with precise natural object location data, elevating Vary-toy’s adaptability and universality. After constructing and fortifying this novel vocabulary, it seamlessly integrates with CLIP and harmoniously amalgamates into a 1.8B language model.
The proof of Vary-toy’s mettle lies in the experimental results, where it shines brightly on challenging benchmarks such as DocVQA, ChartQA, MMvet, and RefCOCO. With impressive performances, including a remarkable 65.6% ANLS on DocVQA, 59.1% accuracy on ChartQA, 88.1% accuracy on RefCOCO, and a respectable 29% on MMVet, Vary-toy stands as a compact yet potent LVLM.
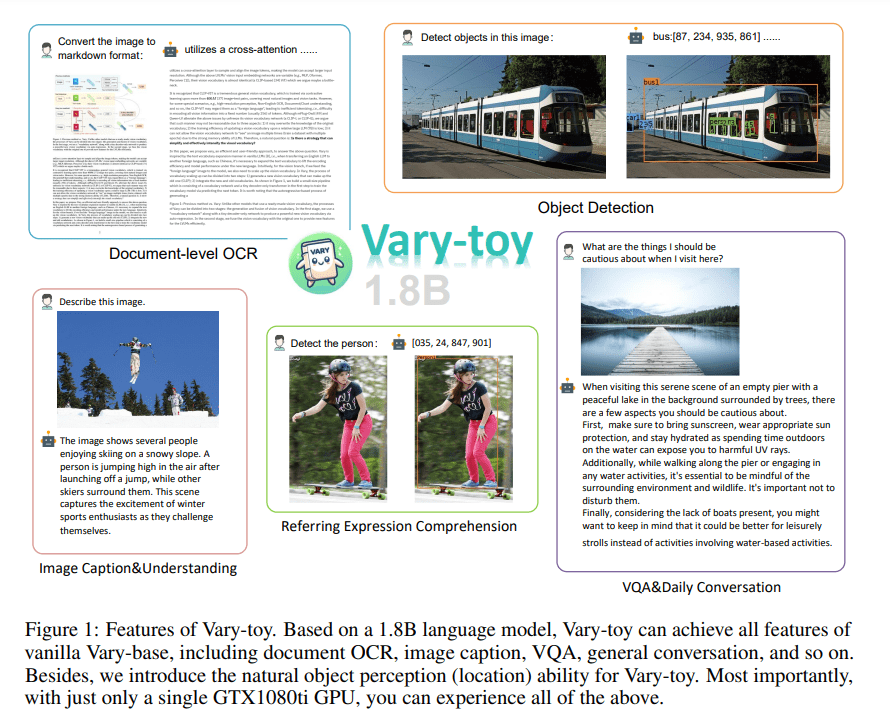
Source: Marktechpost Media Inc.
Conclusion:
The introduction of Vary-toy represents a significant advancement in the realm of large vision language models. Addressing key challenges in vision vocabulary networks and computational costs, enhances the adaptability and universality of LVLMs. With impressive performance on benchmarks and its compact size, Vary-toy is poised to empower researchers with limited resources and drive further innovation in the AI market. Its public code release will foster collaboration and propel the development of practical AI applications.