TL;DR:
- Microsoft’s Neural Graphical Models (NGMs) redefine probabilistic graphical models (PGMs).
- NGMs break free from traditional PGM constraints, accommodating diverse data types.
- They leverage deep neural networks to parametrize probability functions efficiently.
- Experimental validations on real and synthetic data showcase NGMs’ prowess.
- NGMs excel in predicting rare events, such as infant mortality causes.
- NGMs offer unparalleled versatility and precision in probabilistic modeling.
Main AI News:
In the realm of uncertainty-driven decision-making, probabilistic graphical models (PGMs) have stood as stalwart companions for data analysts. These models provide a meticulously structured framework to represent intricate relationships within datasets, unraveling underlying probability distributions that encapsulate the intricate interplay of dataset features. Be it data learning, inference generation, or sampling, graphical models unfold a treasure trove of capabilities for navigating complex domains. Nonetheless, these models also grapple with their own set of constraints, dictated by variable types and the intricacies of operations.
Traditional PGMs have demonstrated their prowess across diverse domains, but their flexibility is tethered. A plethora of graphical models confine themselves to continuous or categorical variables, curtailing their usefulness across data landscapes of diverse types. Further, limitations like the prohibition of continuous variables as parents of categorical variables in directed acyclic graphs (DAGs) encumber their adaptability. Moreover, conventional graphical models exhibit limitations in representing probability distributions, often showing a predilection for multivariate Gaussian distributions.
In a monumental stride towards addressing these challenges, Microsoft researchers have presented their groundbreaking “Neural Graphical Models” (NGMs) in a paper unveiled at the 17th European Conference on Symbolic and Quantitative Approaches to Reasoning with Uncertainty (ECSQARU 2023). NGMs introduce a novel breed of PGMs that harness the might of deep neural networks to glean and effectively encapsulate probability functions across a domain. What distinguishes NGMs is their unparalleled capability to transcend the familiar constraints of traditional PGMs.
NGMs unfurl an expansive canvas for modeling probability distributions, unshackling themselves from the straitjacket of variable types or predefined distributions. This empowerment enables them to gracefully handle an eclectic assortment of data inputs, ranging from categorical and continuous variables to images and embeddings. Moreover, NGMs deliver swift solutions for inference and sampling, elevating them to a commanding status in the realm of probabilistic modeling.
At the heart of NGMs lies the ingenious idea of employing deep neural networks to parameterize probability functions within a given domain. This neural network efficiently undergoes training, driven by the optimization of a loss function that concurrently enforces adherence to a specified dependency structure (presented as an input graph, either directed or undirected) and seamlessly fits the data. Unlike their conventional counterparts, NGMs shatter the boundaries of common constraints, adroitly navigating the intricate landscape of diverse data types.
Diving deeper into the world of NGMs, let us explore their performance through rigorous experimental validations conducted on both real-world and synthetic datasets:
- Infant Mortality Data: The researchers harnessed data sourced from the Centers for Disease Control and Prevention (CDC), with a specific focus on pregnancy and birth variables related to live births in the United States. This dataset also encompassed vital information on infant mortality—a domain notorious for its rarity. Astonishingly, NGMs exhibited an enviable level of inference accuracy, surpassing conventional methods like logistic regression and Bayesian networks. They held their ground alongside Explainable Boosting Machines (EBM) for categorical and ordinal variables.
- Synthetic Gaussian Graphical Model Data: In addition to real-world datasets, NGMs were subjected to a rigorous evaluation using synthetic data derived from Gaussian Graphical Models. Here, NGMs proved their mettle, showcasing an innate capacity to adapt to intricate data structures and deliver commendable performance in this synthetic terrain.
- Lung Cancer Data: The NGMs were further validated using a dataset culled from Kaggle, centered around lung cancer—a field of immense significance. While specific results pertaining to this dataset were not delved into extensively, it unequivocally underlines the versatility of NGMs across an array of domains.
A standout facet of NGMs is their remarkable ability to tackle scenarios where conventional models falter, especially in the prediction of low-probability events. For instance, NGMs shine when it comes to predicting the cause of infant mortality, even in cases where such events are exceedingly rare. This underscores the robustness of NGMs and underscores their immense potential in domains where precision in predicting infrequent outcomes assumes paramount importance.
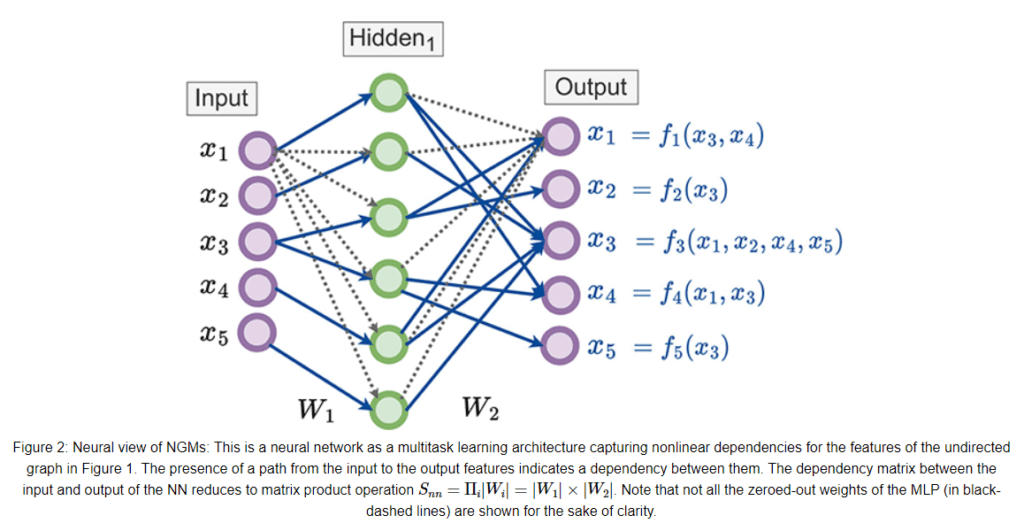
Source: Marktechpost Media Inc.
Conclusion:
Microsoft’s Neural Graphical Models (NGMs) represent a seismic shift in the world of probabilistic graphical models. Their ability to handle diverse data types, break free from constraints, and excel in predicting rare events opens up new horizons for businesses across various domains. NGMs empower organizations to make data-driven decisions with unparalleled precision and flexibility, positioning them as a valuable asset in an increasingly data-centric market.