TL;DR:
- SEINE is a Short-to-Long Video Diffusion Model designed to enhance long video production.
- Existing video generation techniques primarily focus on short “shot-level” videos, limiting cinematic potential.
- SEINE bridges scenes with smooth transitions, addressing the shortcomings of traditional methods.
- It uses a random mask module and textual descriptions to generate transition frames.
- The model is trained to predict noise, ensuring realistic and visually coherent transitions.
- SEINE aims to revolutionize video production by enabling high-quality long videos with creative transitions.
Main AI News:
In the realm of video generation, a new contender has emerged, and it goes by the name of SEINE – a Short-to-Long Video Diffusion Model. This innovative model is set to redefine the landscape of extended video content by offering high-quality videos with seamless and creative transitions between scenes.
The Success of Diffusion Models
SEINE draws inspiration from the remarkable success of diffusion models in the realm of text-to-image generation. These models have paved the way for a surge of video generation techniques, opening up a world of exciting possibilities. However, there’s a catch – most existing video generation techniques are confined to the “shot-level.” In other words, they produce short videos, typically spanning just a few seconds and showcasing a single scene.
The Limitations of Shot-Level Videos
While shot-level videos have their place, they fall short when it comes to meeting the demands of cinematic and film productions. In the world of cinematic and industrial-level video production, “story-level” long videos reign supreme. These videos are characterized by a mosaic of distinct shots, each featuring different scenes. These shots, varying in length, are seamlessly interconnected through the art of transitions and editing, resulting in longer videos and more intricate visual storytelling.
The Role of Transitions
Transitions play a pivotal role in post-production, bringing together scenes or shots with finesse. Traditional transition methods like dissolves, fades, and wipes have their merits, but they rely on predefined algorithms or established interfaces. These methods often lack the flexibility and creative potential required to elevate video production to new heights.
SEINE’s Innovative Approach
Enter SEINE, a groundbreaking video diffusion model that addresses the need for seamless and smooth transitions in long videos. What sets SEINE apart is its focus on generating intermediate frames that bridge the gap between two distinct scenes. This model aims to create transition frames that are not only semantically relevant to the given scene image but also coherent, smooth, and consistent with the provided text.
The Power of SEINE
SEINE, short for Short-to-Long Video Diffusion Model, is here to revolutionize video generation. Its primary objective is clear: to produce high-quality long videos with fluid and imaginative transitions between scenes, accommodating a wide range of shot-level video lengths.
Incorporating Random Mask Modules
To achieve this, SEINE incorporates a random mask module that enables the generation of previously unseen transition and prediction frames based on conditional images or videos. Using a pre-trained variational auto-encoder, N-frames are extracted from the original videos and encoded into latent vectors. Additionally, SEINE takes textual descriptions as input, enhancing the controllability of transition videos and harnessing the power of short text-to-video generation.
The Training Process
During the training stage, the latent vector undergoes controlled corruption with noise, and a random-mask condition layer is applied to capture an intermediate representation of motion between frames. This masking mechanism selectively retains or suppresses information from the original latent code. SEINE leverages this masked latent code and the mask itself as conditional input to determine which frames are masked and which remain visible. The model is trained to predict the noise affecting the entire corrupted latent code, effectively learning the underlying distribution of noise affecting both unmasked frames and the textual description.
A Vision of Realism and Coherence
Through this intricate process of modeling and predicting noise, SEINE aspires to generate transition frames that are not only realistic but also visually coherent. These frames seamlessly blend visible frames with unmasked frames, ensuring a captivating and uninterrupted viewing experience.
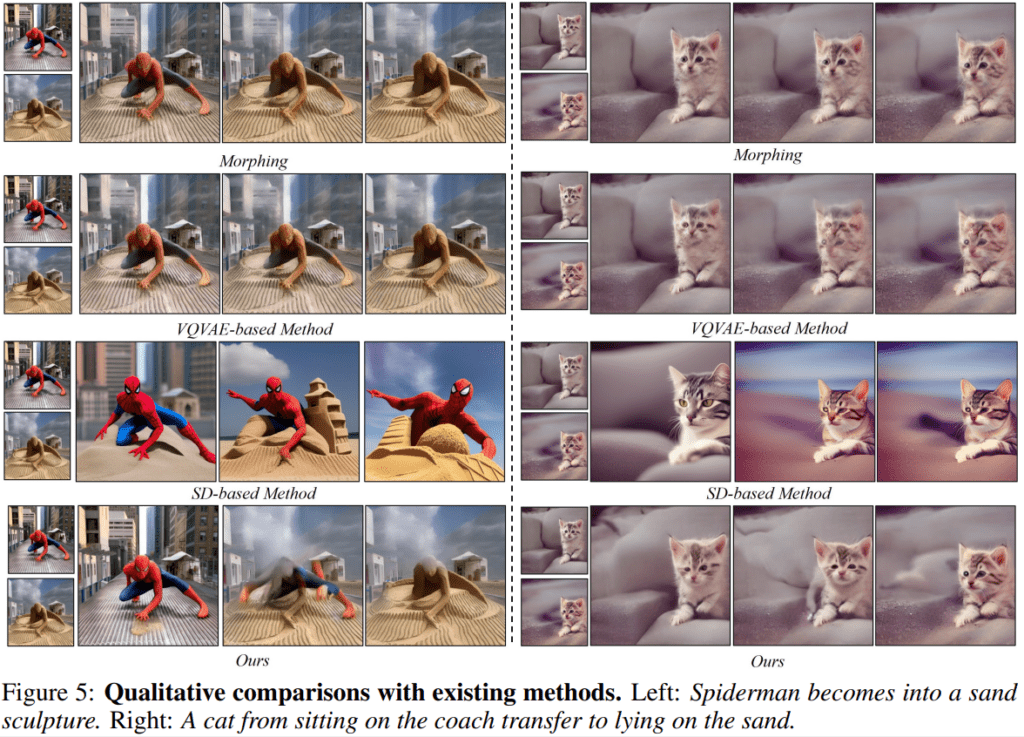
Source: Marktechpost Media Inc.
Conclusion:
SEINE’s innovative approach to long video production and seamless transitions opens up exciting opportunities in the market. With its ability to generate high-quality, cinematic videos, SEINE is set to meet the demands of film and industrial-level video productions. Its potential impact on the market is significant, offering a new level of creativity and flexibility in video content creation.