TL;DR:
- Northwestern Medicine unveils a pioneering deep learning algorithm for genomic research.
- The algorithm identifies polyadenylation sites on the human genome, essential for gene expression.
- Researchers gain insights into factors controlling cleavage sites and site strength.
- Multiple signals near polyadenylation sites influence their precise location.
- The algorithm provides a roadmap for efficient polyadenylation site prediction.
- Expansion plans include applying the model to various species for evolutionary insights.
- The breakthrough has significant implications for understanding genetic mutations and diseases.
Main AI News:
Northwestern Medicine researchers have unveiled a groundbreaking deep learning algorithm, showcased in Nature Communications, which promises to revolutionize our understanding of the human genome. This innovative technology has the potential to reshape the landscape of medical research by accelerating the study of diseases and disorders linked to DNA transcription irregularities.
Polyadenylation, a pivotal process in gene expression, involves adding nucleotides to RNA molecules, thereby stabilizing them for the eventual translation into proteins. Equally important, it cues RNA to halt transcription, preventing erroneous gene expression. While polyadenylation’s implications for conditions like cancer, neonatal diabetes, and genetic disorders are well-established, the precise location of polyadenylation sites on the genome and the factors influencing them have remained elusive.
Dr. Zhe Ji, an assistant professor of Pharmacology at the McCormick School of Engineering and the study’s senior author, emphasizes the focus on understanding the control of transcription termination. He explains, “This polyadenylation process is essential for defining the three-prime ends of the RNA and also for transcription termination. In my field, there’s this challenging question: ‘Where are these polyadenylation sites localized in the genome sequence?’ We want to understand where and how these signals occur so we can optimize them across the human genome.”
Emily Stroup, a Ph.D. candidate and the first author of the study, developed sophisticated deep-learning models to analyze and predict polyadenylation sites on the human genome. These models not only pinpoint the sites but also shed light on the factors governing their precise cleavage and strength relative to others.
Stroup highlights the model’s advantages, stating, “The major advantage of having a series of models like this is that we can learn from what the model learned during training to better understand how polyA site usage is regulated and the factors that determine where the exact cleavage sites are chosen.”
One key revelation from this research is the multitude of signals near polyadenylation sites influencing their location. Furthermore, the model identifies human genome sequences where polyadenylation occurs efficiently, providing a roadmap for future research endeavors.
Stroup explains, “We were able to look at the factors that control how precisely cleavage sites are defined and then what controls the usage of those sites and how we can modulate that.”
Dr. Ji is excited about the prospects this breakthrough brings, saying, “It’s a breakthrough, basically. With this model, for the first time, we can achieve this single-nucleotide resolution prediction of these polyA sites across the human genome because the site is determined by multiple signals.”
Moving forward, Stroup and Dr. Ji are committed to extending their models to other species, including zebrafish, fruit flies, and yeast, to gain insights into polyadenylation site variations across different genomes. This research promises to deepen our understanding of genetic evolution and its implications for various diseases.
Dr. Ji concludes, “We’re hoping that will allow us to understand how signal mutations can contribute to population genetics or different kinds of human diseases, such as muscular dystrophy, neuronal disorders, and cancers.”
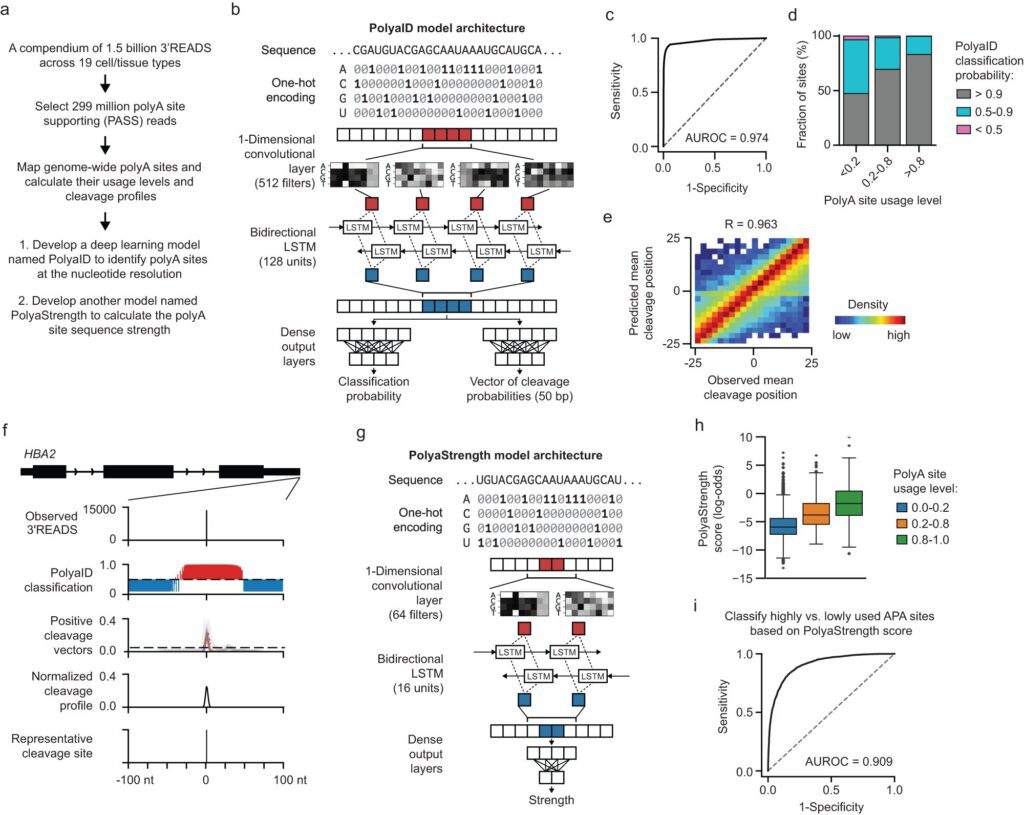
Source: Medical Xpress
Conclusion:
Northwestern Medicine’s groundbreaking deep learning algorithm is poised to transform the genomics research landscape. By unraveling the complexities of polyadenylation sites and their regulation, this innovation opens doors to a deeper understanding of genetic mutations and their role in diseases. The potential applications span various species, promising valuable insights into genetic evolution. This advancement has the potential to catalyze significant breakthroughs in healthcare and pharmaceutical industries, driving innovation and precision medicine.