- Google introduces ScreenAI, a vision-language model for UI and infographic understanding.
- ScreenAI improves upon PaLI architecture with a flexible patching strategy from pix2struct.
- Training involves two stages: pre-training with self-supervised learning and fine-tuning with human-labeled data.
- Data generation includes compiling extensive screenshots dataset and annotating UI elements and textual content.
- ScreenAI excels in question answering, screen navigation, and summarization tasks.
- Achieves state-of-the-art performance on various UI and infographic-based tasks.
- Novel benchmark datasets such as Screen Annotation and Complex ScreenQA set a baseline for future research.
Main AI News:
In today’s digital age, user interfaces (UIs) and infographics serve as vital conduits for human communication and interaction with machines. From intricate charts to interactive diagrams, these visual elements enrich user experiences, but also pose significant challenges for understanding and interaction due to their complexity and diverse formats. Recognizing this, Google unveils “ScreenAI: A Vision-Language Model for UI and Infographics Understanding,” a groundbreaking solution poised to transform the landscape of UI and visually-situated language understanding.
Understanding the Challenge
UIs and infographics share a common visual language, encompassing icons, layouts, and design principles. Yet, deciphering these elements accurately requires a model capable of comprehending, reasoning, and interacting with diverse interfaces effectively. The complexity and variability inherent in UIs and infographics necessitate innovative approaches to modeling and understanding.
Introducing ScreenAI
ScreenAI builds upon the PaLI architecture, integrating the flexible patching strategy from pix2struct to enhance its capabilities. This multimodal model leverages a vision transformer (ViT) for image embeddings and a multimodal encoder for text and image embeddings, enabling it to tackle a myriad of vision tasks framed as text+image-to-text problems.
Training and Data Generation
ScreenAI undergoes two-stage training: pre-training and fine-tuning. The pre-training phase employs self-supervised learning to generate data labels, while the fine-tuning stage incorporates human-labeled data to refine the model’s performance. Data generation involves compiling an extensive dataset of screenshots across various devices, annotated with UI elements, spatial relationships, and textual content using advanced techniques, including layout annotation and optical character recognition (OCR).
Enhanced Capabilities
Through pre-training data diversity and leveraging large language models (LLMs) with structured schemas, ScreenAI simulates diverse user interactions and scenarios. It excels in tasks such as question answering, screen navigation, and summarization, enabling seamless interaction with UIs and infographics.
Experiments and Results
Fine-tuned on public QA, summarization, and navigation datasets, ScreenAI achieves state-of-the-art performance on various UI and infographic-based tasks, including Chart QA, DocVQA, and InfographicVQA. It demonstrates competitive performance on screen summarization and OCR-VQA tasks. Additionally, novel benchmark datasets such as Screen Annotation and Complex ScreenQA provide a baseline for future research in this domain.
Implications and Future Directions
The advent of ScreenAI marks a significant leap forward in UI and infographic understanding, promising enhanced user experiences and streamlined human-machine interaction. As Google continues to innovate in this space, ScreenAI lays the foundation for further advancements, driving progress in visual language understanding and multimodal interaction.
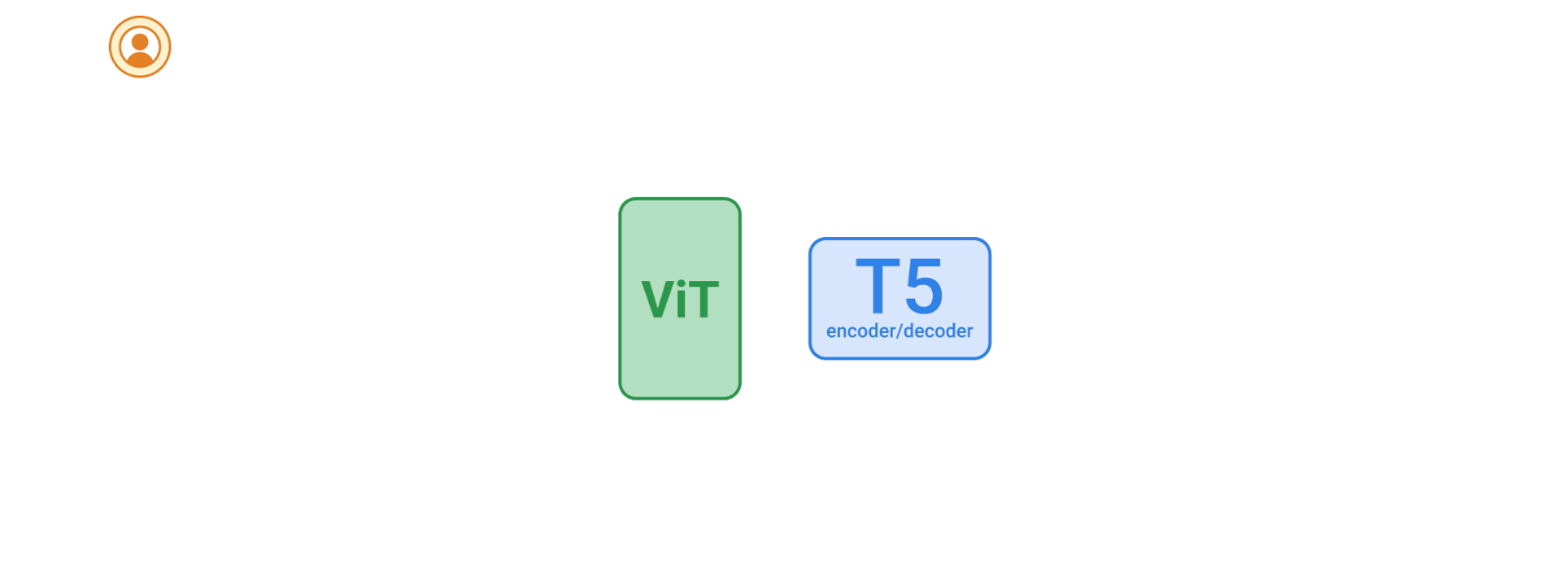
Source: Google
Conclusion:
Google’s introduction of ScreenAI marks a significant advancement in the market for visual language understanding. By leveraging innovative architecture and training methodologies, ScreenAI not only enhances user experiences but also sets new benchmarks for performance in tasks related to UI and infographic understanding. This signals a shift towards more sophisticated and efficient human-machine interaction, with implications for diverse industries relying on visual information processing. As ScreenAI continues to evolve, it is poised to reshape the landscape of visual language understanding, driving innovation and efficiency across various sectors.