- Stanford University introduces Octopus v2, a groundbreaking on-device language model aimed at addressing latency, accuracy, and privacy concerns associated with existing models.
- Octopus v2 significantly reduces latency and enhances accuracy for on-device applications, surpassing GPT-4 in efficiency and speed while slashing context length by 95%.
- The methodology involves fine-tuning a 2 billion parameter model derived from Gemma 2B on a tailored dataset focusing on Android API calls, incorporating functional tokens for precise function calling.
- Octopus v2 achieves a remarkable 99.524% accuracy rate in function-calling tasks, with latency minimized to 0.38 seconds per call and requiring only 5% of the context length for processing.
Main AI News:
In the realm of Artificial Intelligence (AI), particularly in the domain of large language models (LLMs), the quest for equilibrium between model efficacy and real-world constraints like privacy, cost, and device compatibility has been a pressing concern. While cloud-based models boast remarkable accuracy, their dependency on constant internet connectivity, potential privacy vulnerabilities, and exorbitant costs present formidable challenges. Moreover, deploying these models on edge devices introduces complexities in maintaining optimal latency and accuracy due to hardware limitations.
Numerous endeavors, such as Gemma-2B, Gemma-7B, and Llama-7B, along with frameworks like Llama cpp and MLC LLM, have been undertaken to bolster AI efficiency and accessibility. Initiatives like NexusRaven, Toolformer, and ToolAlpaca have pushed the boundaries of function-calling in AI, striving to emulate the effectiveness of GPT-4. Techniques like LoRA have eased fine-tuning under GPU constraints. However, these efforts grapple with a critical bottleneck: achieving a harmonious blend of model size and operational efficiency, especially for low-latency, high-accuracy applications on resource-constrained devices.
Enter Stanford University’s latest innovation, Octopus v2, an advanced on-device language model poised to tackle the prevailing challenges of latency, accuracy, and privacy associated with current LLM applications. Unlike its predecessors, Octopus v2 boasts significant latency reduction and accuracy enhancement for on-device applications. Its groundbreaking approach revolves around fine-tuning with functional tokens, enabling precise function calling and surpassing GPT-4 in efficiency and speed, all while slashing the context length by a staggering 95%.
The methodology behind Octopus v2 entails fine-tuning a 2 billion parameter model derived from Google DeepMind’s Gemma 2B on a meticulously curated dataset focusing on Android API calls. This dataset incorporates both positive and negative examples to refine function calling precision. The training regimen incorporates full model and Low-Rank Adaptation (LoRA) techniques to optimize performance for on-device execution. The crux of the innovation lies in the introduction of functional tokens during fine-tuning, which drastically curtails latency and context length requirements. This breakthrough enables Octopus v2 to achieve remarkable accuracy and efficiency in function calling on edge devices without the need for extensive computational resources.
In benchmark evaluations, Octopus v2 astoundingly achieved a 99.524% accuracy rate in function-calling tasks, vastly outperforming GPT-4. Moreover, the model exhibited a remarkable reduction in response time, with latency minimized to a mere 0.38 seconds per call, marking a 35-fold improvement compared to prior models. Furthermore, Octopus v2 necessitated a mere 5% of the context length for processing, underscoring its prowess in handling on-device operations with unparalleled efficiency. These metrics underscore Octopus v2’s transformative strides in mitigating operational demands while upholding exceptional performance levels, solidifying its position as a monumental advancement in on-device language model technology.
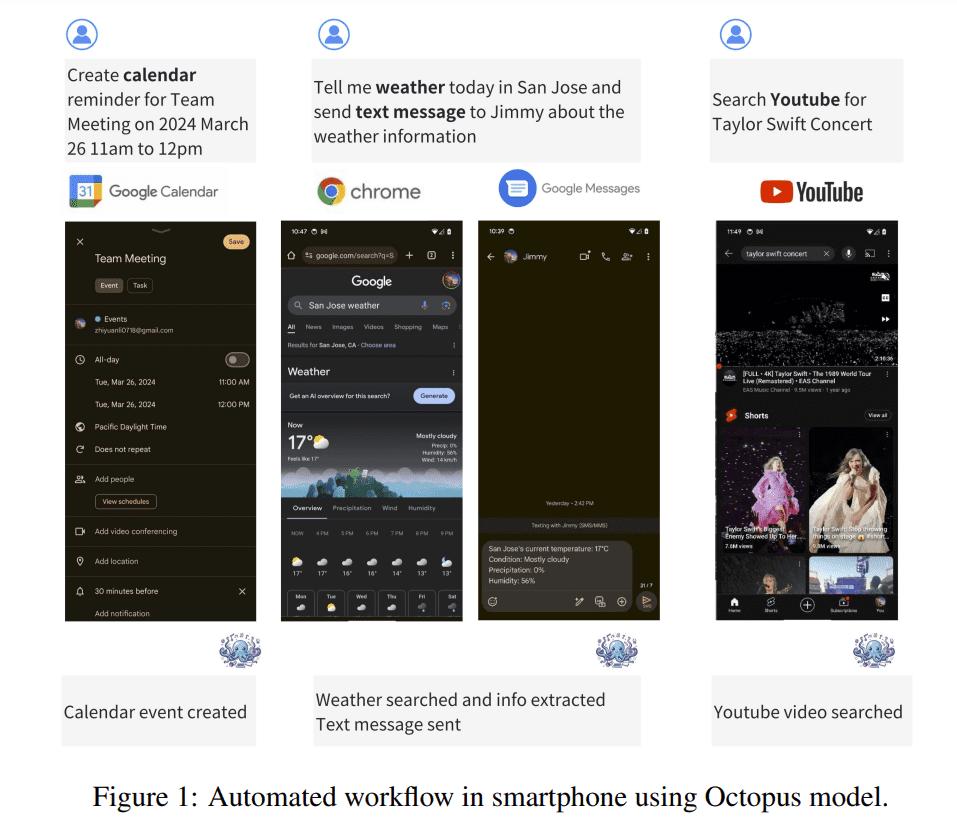
Source: Marktechpost Media Inc.
Conclusion:
Stanford’s Octopus v2 represents a significant leap forward in on-device language model technology. Its ability to drastically reduce latency and context length while enhancing accuracy has profound implications for various markets, particularly those reliant on AI applications with stringent privacy and efficiency requirements. Octopus v2’s advancements are poised to revolutionize the landscape of on-device AI, offering unparalleled performance and paving the way for innovative solutions across industries.