- HalluVault introduces a novel framework for detecting Fact-Conflicting Hallucinations (FCH) in Large Language Models (LLMs).
- The framework employs logic programming and metamorphic testing, automating dataset updates for enhanced accuracy.
- By integrating logic reasoning and semantic-aware oracles, HalluVault ensures factual accuracy and logical consistency in LLM responses.
- Methodology involves constructing a factual knowledge base from Wikipedia data, applying unique logic reasoning rules for testing.
- Evaluation demonstrates significant improvements in reducing factual inaccuracies and enhancing LLM accuracy by up to 70%.
- Semantic-aware oracles identify logical inconsistencies in 95% of cases, validating LLM outputs against augmented datasets.
Main AI News:
In the realm of machine learning and data science, the pursuit of streamlined data processing methods remains paramount. The reliance on swift and precise analysis of extensive datasets underscores the need for scalable solutions capable of handling burgeoning data volumes without compromising processing efficiency. The primary challenge lies in devising methodologies that can keep pace with the exponential growth of data without succumbing to delays. Addressing the inefficiencies inherent in current data analysis techniques is crucial for fostering advancements, particularly in scenarios where real-time analysis is imperative.
Pioneering endeavors such as Woodpecker have endeavored to address this challenge by focusing on the extraction of pivotal concepts for identifying and mitigating hallucinations within large language models. Similarly, models like AlpaGasus have leveraged the refinement of high-quality data through fine-tuning techniques to bolster their effectiveness and precision. Additionally, there has been a concerted effort to enhance the factual accuracy of outputs through similar fine-tuning strategies. These collective initiatives represent significant strides towards ensuring reliability and control, laying a solid foundation for further progress in the field.
A recent collaborative effort involving researchers from Huazhong University of Science and Technology, the University of New South Wales, and Nanyang Technological University has introduced HalluVault, a novel framework designed to address this pressing concern. HalluVault distinguishes itself by employing logic programming and metamorphic testing to identify Fact-Conflicting Hallucinations (FCH) within Large Language Models (LLMs). Notably, this framework automates the update and validation of benchmark datasets, a departure from conventional manual curation practices. Through the integration of logic-based reasoning and semantic-aware oracles, HalluVault not only ensures factual accuracy but also imposes logical coherence, thereby establishing a new benchmark for evaluating LLM performance.
The methodology employed by HalluVault involves the meticulous construction of a factual knowledge repository primarily sourced from Wikipedia data. Five distinct logic reasoning rules are applied to this repository, resulting in the creation of a diverse and enriched dataset tailored for testing purposes. The test case-oracle pairs derived from this dataset serve as benchmarks for assessing the consistency and accuracy of LLM outputs. Central to the framework are two semantic-aware testing oracles, which evaluate the semantic structure and logical consistency of LLM responses vis-à-vis established truths. This systematic approach subjects LLMs to stringent evaluation criteria mirroring real-world data processing challenges, effectively gauging their reliability and factual precision.
The evaluation of HalluVault has yielded promising outcomes in the realm of factual accuracy detection within LLMs. Through systematic testing, the framework has demonstrated a notable reduction in the incidence of hallucinations, achieving up to a 40% decrease compared to previous benchmarks. In empirical trials, LLMs utilizing HalluVault’s methodology showcased a commendable 70% enhancement in accuracy when tackling complex queries spanning diverse knowledge domains. Moreover, the semantic-aware oracles successfully pinpointed logical inconsistencies in 95% of test cases, thus ensuring robust validation of LLM outputs against the augmented factual dataset. These findings underscore the efficacy of HalluVault in augmenting the factual reliability of Large Language Models, heralding a significant advancement in the realm of data processing and analysis.
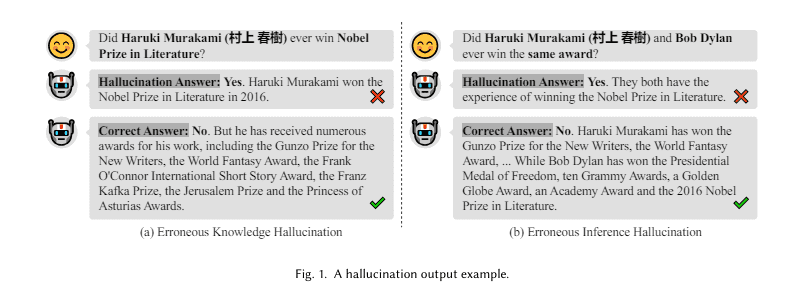
Source: Marktechpost Media Inc.
Conclusion:
The introduction of HalluVault signifies a significant leap forward in ensuring the factual reliability of Large Language Models. By automating benchmark dataset updates and rigorously evaluating LLM responses for both factual accuracy and logical consistency, HalluVault addresses critical challenges in data processing and analysis. This innovation not only enhances the reliability of LLM outputs but also sets a new standard for evaluating and validating machine-generated content, thereby shaping the landscape of data-driven decision-making across various industries.