- Integration of large language models (LLMs) and simulations revolutionizes scientific inquiry.
- Challenges in simulating observational feedback and theoretical models in physical sciences addressed.
- Scientific Generative Agent (SGA) introduced, merging LLMs and simulations for unified scientific exploration.
- SGA employs a two-level process: LLMs for hypothesis generation, simulations for parameter optimization.
- SGA outperforms traditional methods in constitutive law discovery and molecular design tasks.
- Significant reduction in loss values achieved by SGA across various scientific tasks.
Main AI News:
The convergence of advanced computational techniques and scientific inquiry has birthed a new frontier in accelerating the pace of discovery. In this landscape, the fusion of large language models (LLMs) with simulations stands as a beacon, illuminating pathways to enhanced hypothesis generation, experimental design, and data analysis. This synergy not only promises to democratize access to cutting-edge research tools but also holds the potential to push the boundaries of scientific knowledge across diverse domains.
Challenges abound for researchers endeavoring to bridge the realms of observational feedback and theoretical modeling in physical sciences. Conventional methods, constrained by their lack of universality, have often hindered the quest for innovative breakthroughs. The clarion call for a more comprehensive and adaptable framework resounds loudly, urging the scientific community to forge ahead in pursuit of more effective methodologies.
Pioneering endeavors have seen the integration of LLMs with domain-specific data, yielding promising avenues for scientific exploration. Techniques such as Chain-of-Thoughts prompting, FunSearch, and Eureka have leveraged the prowess of LLMs in problem-solving realms. Meanwhile, Neural Architecture Search (NAS) has honed in on optimizing neural network structures, while symbolic regression and population-based molecule design have contributed to advancing scientific frontiers. These approaches, coupled with differentiable simulations, have not only augmented hypothesis generation but have also widened the scope of automated scientific inquiry.
Enter the Scientific Generative Agent (SGA), a groundbreaking bilevel optimization framework introduced by researchers from esteemed institutions including MIT CSAIL, CMU LTI, UMass Amherst, and the MIT-IBM Watson AI Lab. SGA heralds a new dawn in scientific discovery, seamlessly integrating LLMs and simulations to transcend disciplinary boundaries and offer a unified approach to physical science exploration. By amalgamating the abstract reasoning capabilities of LLMs with the computational prowess of simulations, SGA presents a holistic methodology for scientific inquiry.
At the heart of SGA lies a two-level process: LLMs spearhead hypothesis generation at the outer level, while simulations fine-tune continuous parameters at the inner level. Drawing upon QM9 datasets for molecular design and employing differentiable Material Point Method (MPM) simulators for constitutive law discovery, the framework iteratively refines hypotheses by amalgamating discrete symbolic variables with continuous parameters. The outcome? Enhanced material properties, precise molecular structures, and a paradigm shift in scientific problem-solving.
The empirical evidence speaks volumes: SGA outshines its counterparts with remarkable finesse. In constitutive law discovery, SGA boasts a staggering 50% reduction in loss compared to conventional baselines. Moreover, its prowess in optimizing molecules with specific quantum properties is unparalleled, achieving a minuscule loss value of 0.0001 in the HOMO-LUMO gap task, a feat unmatched by traditional methods. With a consistent track record of delivering lower loss values across diverse tasks, SGA emerges as the vanguard of scientific innovation, heralding a future where novel solutions are not just envisioned but accurately identified.
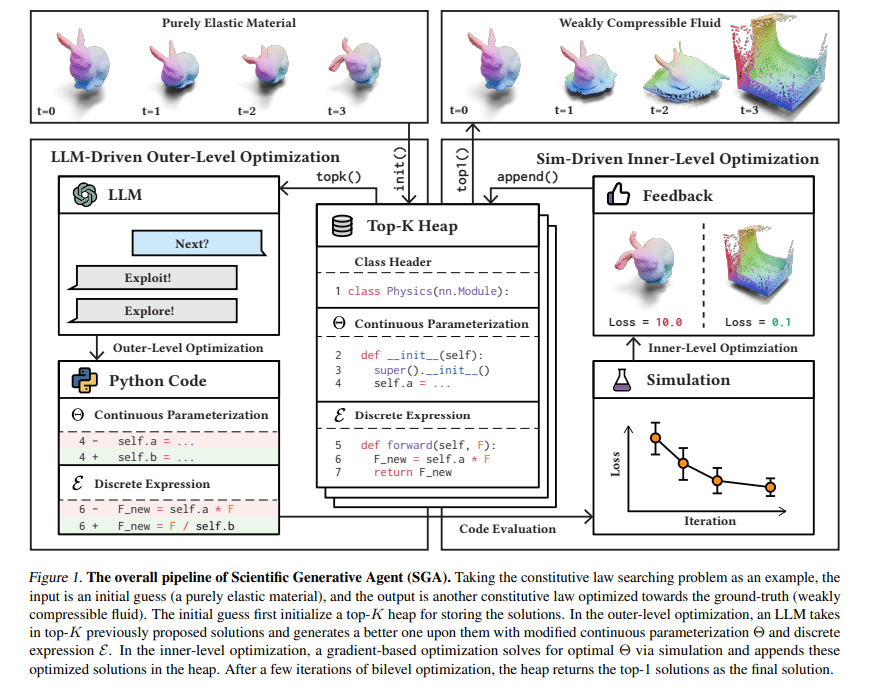
Source: Marktechpost Media Inc.
Conclusion:
The emergence of the Scientific Generative Agent marks a pivotal moment in the scientific landscape, offering a unified framework that transcends disciplinary boundaries. Its ability to seamlessly integrate large language models with simulations not only enhances efficiency but also propels scientific discovery to unprecedented heights. For businesses operating in scientific research and development, embracing such innovative methodologies could lead to accelerated breakthroughs, heightened efficiency, and a competitive edge in an increasingly dynamic market.