TL;DR:
- Machine learning and optimization techniques hold promise for enhancing energy efficiency in complex systems like data centers.
- Dr. Stephen Whitelam conducted computer simulations to train nanosystems, achieving greater energy efficiency.
- The simulations demonstrated that learned protocols could extract heat from systems by continuously measuring and identifying energy-efficient operations.
- Whitelam’s work draws inspiration from Maxwell’s Demon, a thought experiment in physics, and uses machine learning algorithms similar to those in video games to optimize nanoscale systems.
- The simulations offer insights into energy optimization possibilities and the balance between information and energy.
- An analogy to refrigerators highlights the concept of a heat engine, where cooling is achieved despite generating heat.
- Whitelam’s simulations involved optimizing the motion of nanoscale beads through water using an optical trap and flipping the state of a nanomagnetic bit for information processing.
- These simulations serve as a testbed for exploring concepts and offer potential for real-life experiments to minimize energy expenditure and improve energy efficiency.
- The findings present opportunities to revolutionize energy-efficient technologies and address challenges in energy consumption and heat dissipation.
Main AI News:
Discovering innovative ways to enhance energy efficiency is a never-ending pursuit in the realm of physics. However, recent advancements in the field of machine learning, coupled with strategic thinking and the assistance of an unlikely ally—a demon—have unveiled a promising avenue for achieving improved energy efficiency in complex systems, such as data centers.
Dr. Stephen Whitelam, a distinguished researcher at the Department of Energy’s Lawrence Berkeley National Laboratory, conducted a series of computer simulations to explore the potential of neural networks, a type of machine-learning model that mimics the intricate processes of the human brain. These simulations focused on training nanosystems, minute machines comparable in size to molecules, to operate with greater energy efficiency.
Remarkably, the findings revealed that the learned protocols enabled the nanosystems to extract heat from the surroundings by continuously monitoring and identifying the most energy-efficient operations. In other words, not only could energy be harnessed from the system, but work could also be effectively stored within it. This revelation carries immense significance, especially in the context of operating massive systems like computer data centers, where the heat generated by banks of computers must be dissipated to safeguard delicate electronics from harm.
Dr. Whitelam conducted his groundbreaking research at the esteemed Molecular Foundry, a Department of Energy Office of Science user facility nestled within Berkeley Lab. The fruits of his labor are documented in a comprehensive paper published in the esteemed journal Physical Review X, shedding light on the exciting possibilities of energy optimization in complex systems.
Curiously, Dr. Whitelam drew inspiration from unlikely sources, including the classic video game Pac-Man and a thought experiment in physics known as Maxwell’s Demon. He explained that techniques utilized in the realm of machine learning to master Atari video games seemed naturally well-suited to the field of materials science. Analogous to Pac-Man, the objective in this context is to determine the optimal timing for specific actions—be it moving up, down, left, or right—to maximize performance.
Over time, the machine learning algorithms acquire the ability to discern the most effective moves and precisely when to execute them, resulting in remarkable scores. Similarly, these algorithms can be effectively employed to fine-tune nanoscale systems, thereby enhancing their energy efficiency.
Additionally, Dr. Whitelam’s simulations offer a compelling response to Maxwell’s Demon, a thought experiment postulated by the eminent physicist James Clerk Maxwell in 1867. Maxwell envisioned a box filled with gas, with a weightless “demon” stationed in the center, regulating a trap door.
The demon’s task was to selectively open the door, allowing swifter gas molecules to migrate to one side of the box while impeding the movement of slower molecules. As a consequence, the segregated molecules would eventually lead to a stark temperature contrast within the box, with the “slow” side turning cold and the “fast” side becoming hot, resulting in a precise balance of molecular energy.
In the pursuit of unlocking new frontiers in energy efficiency, Dr. Stephen Whitelam, an esteemed researcher at the Department of Energy’s Lawrence Berkeley National Laboratory, has harnessed the concept of optimization, drawing inspiration from Maxwell’s Demon—a groundbreaking thought experiment in physics. By leveraging the potential of machine learning protocols, Whitelam has opened up exciting possibilities for manipulating nanoscale systems, paving the way for improved energy utilization and conservation.
It is important to note that Whitelam’s approach adheres steadfastly to the laws of thermodynamics, discrediting any notion of achieving something for nothing. The key lies in understanding that information is intrinsically linked to energy. While the demon—symbolic of the machine learning protocol—seems to extract energy effortlessly, the underlying process of measuring the position and speed of molecules within the system incurs an energy cost exceeding that obtained from the resulting heat engine.
To illustrate the significance of this paradigm, Whitelam draws a compelling analogy to refrigerators. Despite the apparent contradiction of a cooling mechanism generating heat, refrigerators exemplify the concept of a heat engine. As the system operates, the desired outcome of keeping the food inside cold is achieved, even though the back of the fridge becomes hot due to work executed by the refrigerator’s motor. In this context, the machine learning protocol serves as the demon, effectively converting information derived from the modeled system into heat energy through the process of optimization.
In one of Whitelam’s simulations, he focused on optimizing the motion of a nanoscale bead through water—a task akin to dragging the bead with minimal energy expenditure. By employing an optical trap that employs laser beams as light-based tweezers to manipulate the bead, Whitelam demonstrated that by measuring the inherent fluctuations known as Brownian motion, the optimal moment for moving the bead with the utmost energy efficiency could be determined. This simulation echoes Maxwell’s thought experiment, albeit on a nanoscale with the aid of an optical trap, showcasing the capabilities of the neural-network demon.
Expanding the realm of possibilities, Whitelam further applied this concept to the realm of microelectronics and computation. He simulated the state-flipping of a nanomagnetic bit between 0 and 1—an essential information erasure and information-copying operation in computing. Through repeated iterations, the demon “learns” to flip the bit in a manner that absorbs heat from the surroundings, drawing parallels to the functioning of a refrigerator. Remarkably, this implies the potential to develop a computer that cools down while in operation, with the heat efficiently redirected elsewhere within the data center.
Whitelam emphasizes that these simulations serve as a testbed for exploring and comprehending various concepts and ideas. They validate the notion that these protocols can be executed with minimal energy expenditure or even by absorbing energy from alternative sources, presenting opportunities for real-life experiments. As industries strive to tackle the challenges of energy consumption and heat dissipation, the innovative techniques developed by Dr. Whitelam offer a glimmer of hope for a future where optimization reigns supreme, revolutionizing the landscape of energy-efficient technologies.
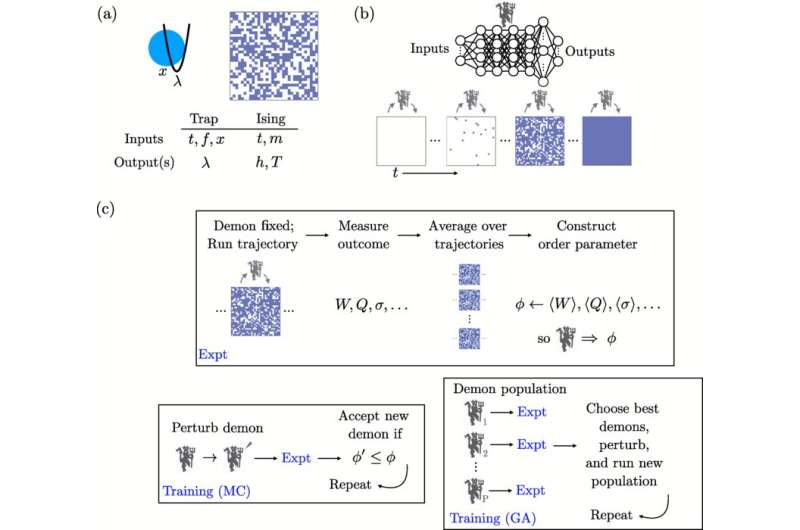
Source: Phys.org
Conlcusion:
The groundbreaking research conducted by Dr. Stephen Whitelam, leveraging machine learning and optimization techniques to enhance energy efficiency in complex systems, carries profound implications for the market. The ability to extract energy from systems while achieving optimal performance and minimizing energy expenditure opens up new possibilities for industries reliant on data centers, microelectronics, and computation.
This breakthrough paves the way for more energy-efficient technologies, which in turn can lead to cost savings, reduced environmental impact, and enhanced competitiveness in the market. As businesses strive to meet the growing demand for energy efficiency, embracing these innovative approaches holds the potential to reshape the landscape and drive transformative changes across industries.