TL;DR:
- Deep learning reconstruction (DLR) is revolutionizing MRI imaging in radiology.
- DLR improves signal-to-noise ratio and image quality in MRI scans.
- It enables denoising without extending imaging time, reducing patient discomfort and scanner costs.
- DLR integrates with accelerated acquisition techniques, reducing reconstruction time.
- DLR uses supervised learning with convolutional layers and has three categories.
- Various derivatives of DLR have shown feasibility in clinical practice.
- DLR may alter imaging features and obscure small lesions depending on network training.
- Radiologists should question if the information is lost in seemingly clean images.
Main AI News:
The realm of radiology is undergoing a groundbreaking transformation with the advent of deep learning technology. Among its various applications, deep learning reconstruction (DLR) has emerged as a game-changing tool in the image reconstruction process of magnetic resonance imaging (MRI). This cutting-edge technique brings a host of benefits to MRI operations, enhancing efficiency and paving the way for unprecedented advancements. However, it is crucial for radiologists to remain vigilant, as DLR has the potential to alter imaging features and obscure small lesions, depending on the training of the convolutional neural network.
One of the most significant contributions of deep learning reconstruction is in the denoising of MRI images. By applying this technique, the signal-to-noise ratio can be significantly improved, leading to superior image quality. This denoising capability has found its way into commercial MRI scanners, particularly in lower magnetic field-strength systems. Remarkably, denoising can be achieved without extending the imaging time while still delivering comparable results to higher-field-strength scanners. Shorter imaging times not only alleviate patient discomfort but also contribute to reduced MRI scanner running costs—a win-win situation.
DLR has seamlessly integrated with accelerated acquisition imaging techniques, such as parallel imaging or compressed sensing, further streamlining the reconstruction process. This integration has resulted in reduced reconstruction times, enabling faster and more efficient image generation. The foundation of DLR lies in supervised learning utilizing convolutional layers and encompasses three distinct categories: image domain, k-space learning, and direct mapping types. Building upon these categories, researchers have explored various derivatives of DLR, demonstrating its feasibility and immense potential in clinical practice.
While DLR excels at reducing Gaussian noise in MR images, it does introduce certain challenges. Denoising can amplify image artifacts, necessitating a comprehensive solution. Radiologists must exercise caution, recognizing that DLR has the capacity to modify the imaging characteristics of lesions and even obscure small lesions altogether. This awareness compels radiologists to cultivate a habit of questioning whether any critical information may have been compromised in seemingly pristine images.
Deep learning reconstruction has opened up unprecedented possibilities in MRI imaging, revolutionizing the field of radiology. By harnessing its power, radiologists can unlock greater efficiency, enhanced image quality, and reduced costs. Nevertheless, it is imperative to acknowledge the potential alterations in imaging features and the obscured visibility of lesions, which are contingent on the specific training of the convolutional neural network. The fusion of deep learning reconstruction and radiology holds immense promise, but the importance of maintaining a discerning eye cannot be overstated.
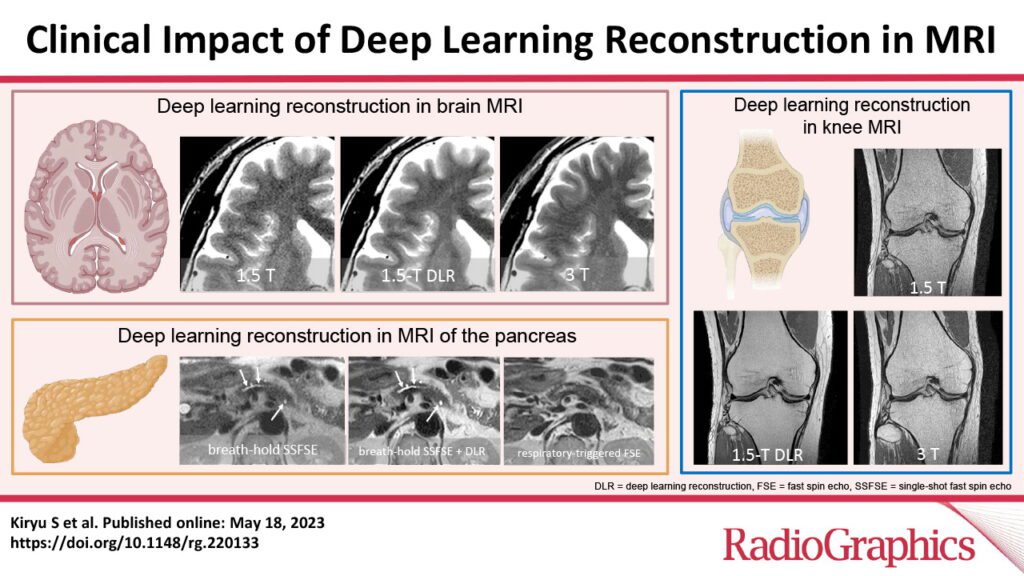
Source: Radiological Society of North America
Conlcusion:
The emergence of deep learning reconstruction (DLR) in MRI imaging represents a significant breakthrough in the field of radiology. This transformative technology offers improved image quality, reduced imaging time, and enhanced denoising capabilities. However, it is important for market participants, such as manufacturers of MRI scanners and radiology centers, to recognize the potential impact of DLR on imaging features and lesion visibility. By staying informed and adapting their strategies accordingly, market players can harness the benefits of DLR while addressing the associated challenges, thus positioning themselves at the forefront of this rapidly evolving market.