TL;DR:
- Groundbreaking deep learning model for AMD classification using OCT scans.
- Two-stage convolutional neural network for precise diagnosis.
- Extensive dataset leads to accurate staging of AMD.
- Significance of OCT scans in detailed AMD assessment.
- Comprehensive performance metrics showcase the model’s reliability.
- Promising results with an average ROC-AUC of 0.94 in real-world testing.
- Potential for future adaptation to various OCT devices and broader applications.
- Uncertainty estimates enhance reliability in clinical settings.
- This is a monumental step toward revolutionizing AMD diagnosis and treatment.
Main AI News:
In the realm of medical innovation, a groundbreaking research paper unveils a cutting-edge deep learning model designed to classify stages of Age-Related Macular Degeneration (AMD) using real-world Retinal Optical Coherence Tomography (OCT) scans. This transformative development holds the promise of revolutionizing AMD diagnosis and treatment protocols, ushering in a new era of precision medicine.
The research paper introduces a sophisticated two-stage convolutional neural network, meticulously crafted for the classification of macula-centered 3D volumes extracted from Topcon OCT images. This neural network, a technological marvel in itself, is poised to redefine how we perceive and manage AMD. The first stage of the model relies on a 2D ResNet50 for the critical task of B-scan classification. Subsequently, the second stage deploys smaller ResNets to perform volume classification with surgical precision.
Underpinned by an extensive dataset, this neural network emerges as a formidable force in categorizing macula-centered 3D volumes, distinguishing between Normal, early/intermediate AMD (iAMD), atrophic (GA), and neovascular (nAMD) stages with remarkable accuracy. Such accuracy is indispensable, as the study emphasizes, for initiating timely treatment – a pivotal factor in preserving precious vision.
The performance metrics employed in this groundbreaking research encompass a comprehensive array of evaluation criteria: ROC-AUC, balanced accuracy, accuracy, F1-Score, sensitivity, specificity, and Matthews correlation coefficient. These metrics collectively underline the model’s prowess and reliability.
This pioneering research endeavor also underscores the pivotal role played by Retinal Optical Coherence Tomography (OCT) scans, a non-invasive imaging technique. Unlike conventional methods, OCT scans provide an unparalleled level of detail in AMD staging, ushering in a new era of diagnostic precision.
To delve deeper into the model’s inner workings, the research elucidates a two-stage deep learning architecture. The first stage, leveraging the power of ImageNet-pretrained ResNet50, localizes disease categories within the volume. Subsequently, the second stage, employing four separate ResNets, performs volume-level classification. This meticulous approach ensures the highest degree of accuracy, laying the foundation for a transformative shift in AMD diagnosis and staging.
The real-world testing phase yielded immensely promising results, with an average ROC-AUC of 0.94 – a testament to the model’s robustness and reliability. Incorporating Monte-Carlo dropout at inference time further enhanced the model’s ability to provide trustworthy classification uncertainty estimates. The study meticulously evaluated its performance using a curated dataset of 3995 OCT volumes from 2079 eyes, employing an array of metrics including AUC, BACC, ACC, F1-Score, sensitivity, specificity, and MCC.
This research paper signifies a remarkable achievement in the field of AMD detection and staging. The two-stage convolutional neural network not only accurately classifies macula-centered 3D volumes but also outperforms baseline approaches, offering the added advantage of B-scan-level disease localization – a feat previously thought unattainable.
Looking ahead, the study points to exciting avenues for further exploration. Adapting the deep learning model to various OCT devices, including Cirrus and Spectralis, could significantly broaden its applicability. Addressing challenges related to dataset-specific training through domain shift adaptation methods is crucial for ensuring consistent performance across diverse signal-to-noise ratios. Additionally, the model’s potential for retrospective AMD onset detection holds promise for the automatic labeling of longitudinal datasets. Furthermore, leveraging uncertainty estimates in real-world screening settings and exploring the model’s capabilities for detecting other disease biomarkers beyond AMD are avenues ripe for future investigation. These endeavors are poised to transform disease screening across a broader population, promising a brighter future for healthcare.
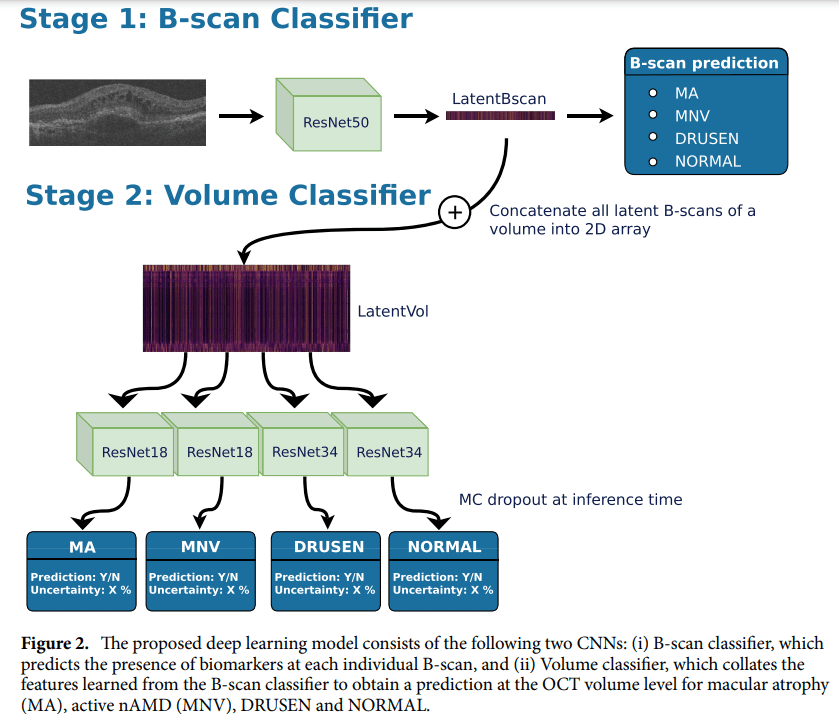
Source: Springer Nature Limited
Conclusion:
The introduction of this AI-powered AMD classification model represents a significant leap forward in retinal health diagnostics. Its potential for precise and reliable diagnosis, coupled with adaptability to various OCT devices, positions it as a game-changer in the market. Healthcare providers can expect improved accuracy in AMD staging, leading to more effective treatments and enhanced patient care. This innovation paves the way for a brighter future in the field of retinal health, promising significant opportunities for growth and advancement in the market.