TL;DR:
- An innovative AI approach, Senseiver, transforms edge computing by extracting extensive data from limited sensors.
- A compact neural network reduces computational resources, which is ideal for drones and sensor arrays.
- Researchers validate Senseiver’s efficiency using real-world sparse data.
- Practical applications include self-driving cars, oil and gas modeling, patient monitoring, and more.
- Los Alamos Laboratory bridges AI advantages to field-based sensors and remote applications.
Main AI News:
In the realm of cutting-edge technology, a groundbreaking approach to artificial intelligence (AI) is revolutionizing the way we harness data. This innovative technique empowers us to extract vast amounts of information, such as comprehensive ocean temperature data, from a limited number of field-deployable sensors using low-powered “edge” computing. With its far-reaching applications spanning across industry, science, and medicine, this novel AI approach is changing the game.
Javier Santos, a dedicated researcher at Los Alamos National Laboratory, has spearheaded the development of a neural network that encapsulates large systems in an exceptionally compact manner. Santos explains, “This compactness significantly reduces the computational resources required compared to state-of-the-art convolutional neural network architectures. As a result, it’s ideally suited for deployment in the field on drones, sensor arrays, and other edge-computing applications, effectively bringing computation closer to its end-users.“
A Boost in Computing Efficiency
In a recent breakthrough, Santos and a team of Los Alamos researchers introduced their pioneering AI technique, aptly named “Senseiver,” in a paper published in Nature Machine Intelligence. Building on Google’s Perceiver IO AI model, they applied the principles of natural-language models, including ChatGPT, to the challenge of reconstructing extensive information about various environments, such as the ocean, from a limited dataset.
What makes Senseiver truly remarkable is its exceptional efficiency. Dan O’Malley, a co-author of the paper and a Los Alamos researcher specializing in machine learning for geoscience, points out, “By utilizing fewer parameters and consuming less memory, the model demands fewer central processing unit cycles on the computer. This results in faster performance on smaller computing devices.”
Real-world Validation
In a pioneering move, Santos and his colleagues at Los Alamos successfully validated the model’s effectiveness using real-world datasets consisting of sparse information. These datasets contained measurements taken from sensors covering only a minute fraction of the target area and complex three-dimensional fluid data.
Practical Applications Unleashed
To showcase the real-world utility of Senseiver, the team applied the model to a sea-surface-temperature dataset provided by the National Oceanic and Atmospheric Administration. This dataset comprised measurements collected over decades from satellites and sensors on ships. From these sparse data points, the model skillfully forecasted temperatures across the entire expanse of the ocean, offering valuable insights for global climate models.
Senseiver’s Potential Unleashed
Senseiver proves to be highly versatile, offering numerous applications and research opportunities at Los Alamos. Hari Viswanathan, a Los Alamos Laboratory Fellow and environmental scientist, notes, “Los Alamos possesses an array of remote sensing capabilities. However, AI models have historically been too large to operate in the field. This limitation has driven us towards edge computing.” Viswanathan adds, “Our work bridges the gap, bringing the advantages of AI to drones, networks of field-based sensors, and other applications that were previously beyond the reach of cutting-edge AI technology.”
One particular area where the AI model shines is in the Lab’s endeavor to identify and characterize orphaned wells. Los Alamos leads the Consortium Advancing Technology for Assessment of Lost Oil & Gas Wells (CATALOG), a federal program focused on locating and characterizing undocumented orphaned wells while measuring their methane emissions. Viswanathan serves as the lead scientist of CATALOG.
Senseiver’s impact extends to various large-scale practical applications, including self-driving cars, remote asset modeling in the oil and gas industry, patient health monitoring, cloud gaming, content delivery, and contaminant tracing. With its compact efficiency, Senseiver unlocks the potential of edge computing, ushering in a new era of AI-driven innovations across diverse domains.
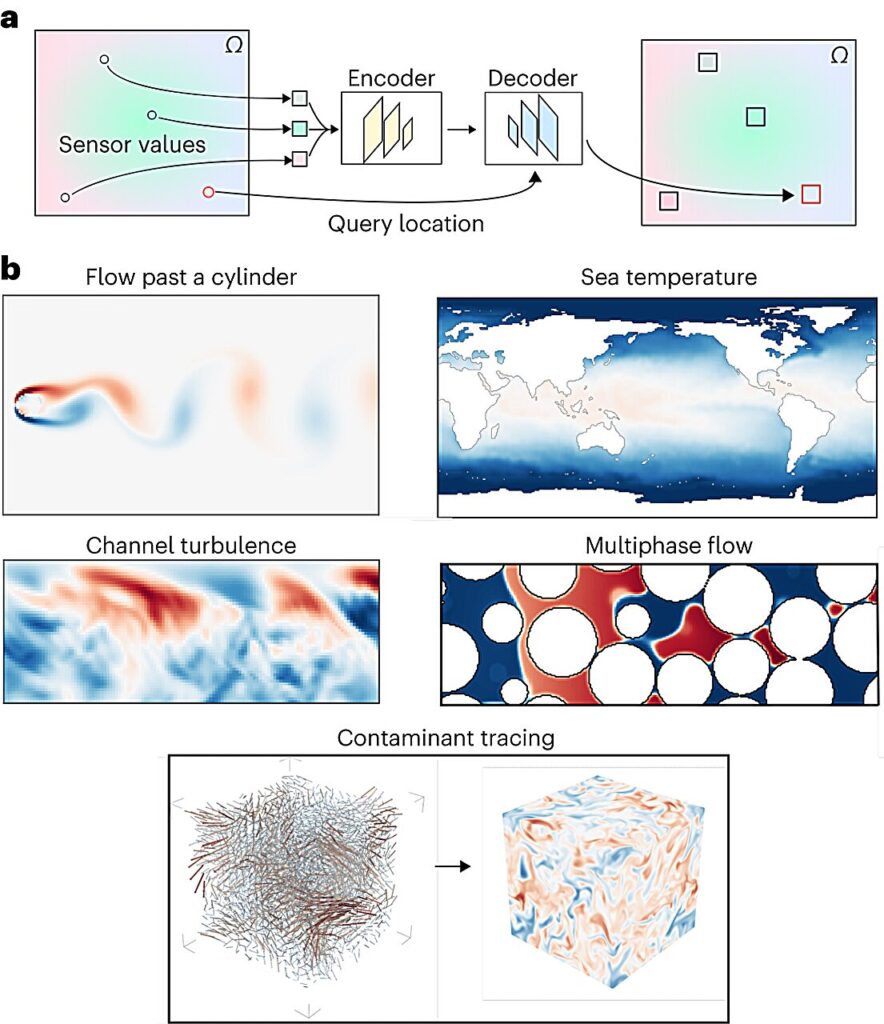
Source: Tech Xplore
Conclusion:
Senseiver’s breakthrough in AI and edge computing opens new possibilities for efficiency and practical applications. Its compact design reduces computational demands, paving the way for widespread use in various industries. The market can expect increased productivity, cost-effectiveness, and innovation as Senseiver propels edge computing to the forefront of technological advancements.