TL;DR:
- The challenge of teaching robots parkour skills for navigating challenging environments is being addressed through an innovative two-stage RL method.
- This approach integrates “soft dynamics constraints” during training, expediting skill acquisition.
- Specialized skill policies, combining recurrent neural networks and multilayer perceptrons, enable informed decision-making based on sensory inputs.
- Simulated environments with increasing obstacle complexity are employed for training.
- Precise reward structures incentivize desired behaviors in reinforcement learning.
- Domain adaptation techniques bridge the gap between simulation and real-world application.
- Vision sensors, including depth cameras, play a crucial role in enabling agile robotic parkour performance.
- The proposed method outperforms baseline approaches, particularly in tasks requiring exploration, climbing, leaping, crawling, and tilting.
Main AI News:
In the realm of robotics, the pursuit of excellence in executing intricate physical maneuvers, particularly those demanded by challenging environments, has remained an enduring challenge. Among the most formidable tests in this sphere lies the domain of parkour—a discipline that demands nothing short of swiftness and dexterity in conquering obstacles. Parkour necessitates a fusion of competencies, including climbing, leaping, crawling, and tilting, which poses a formidable hurdle for robots due to the intricate demands of coordination, perception, and decision-making. The core question that this paper and article endeavor to tackle revolves around the efficient imparting of these nimble parkour skills to robots, empowering them to navigate through a spectrum of real-world scenarios.
Before embarking on a deep dive into the proposed resolution, it is imperative to gain insight into the current landscape of robotic locomotion. Traditional methodologies often entail the manual crafting of control strategies, a process that can be immensely labor-intensive and relatively inflexible when confronted with diverse scenarios. The promise of Reinforcement Learning (RL) in instructing robots in complex tasks is evident; however, RL methods encounter stumbling blocks related to exploration and the seamless transfer of acquired skills from the simulated environment to the tangible world.
Now, let’s delve into the groundbreaking approach set forth by a pioneering research team, addressing these very challenges head-on. These researchers have meticulously engineered a two-stage RL methodology, precisely tailored to instill parkour skills effectively into robots. The crux of their methodology lies in the integration of “soft dynamics constraints” during the initial training phase, a critical factor for expeditious skill acquisition.
The researchers’ approach comprises several pivotal components that contribute to its efficacy.
- Specialized Skill Policies: At its core, the method revolves around the formulation of specialized skill policies that are indispensable for mastering parkour. These policies are meticulously crafted using a blend of Recurrent Neural Networks (GRU) and Multilayer Perceptrons (MLP) to produce joint positions. They take into account a myriad of sensory inputs, including depth images, proprioception (the awareness of the body’s position), prior actions, and more. This amalgamation of inputs empowers robots to make discerning decisions grounded in their surroundings.
- Soft Dynamics Constraints: The ingenious aspect of this approach lies in the incorporation of “soft dynamics constraints” during the initial training phase. These constraints serve as guiding beacons in the learning process, providing robots with indispensable information about their environment. By introducing these soft dynamics constraints, the researchers ensure that robots can explore and master parkour skills with remarkable efficiency. The result? Expedited learning curves and heightened performance.
- Simulated Environments: To cultivate the specialized skill policies, the researchers employ simulated environments, meticulously constructed with IsaacGym. These virtual realms feature 40 distinct tracks, each housing 20 obstacles of varying complexities. The properties of these obstacles, spanning height, width, and depth, progressively escalate in intricacy across the tracks. This setup equips robots with the ability to progressively hone their parkour skills, conquering increasingly challenging terrains.
- Reward Structures: Within the realm of reinforcement learning, the role of reward structures cannot be overstated. The researchers painstakingly define reward parameters tailored to each specialized skill policy. These reward metrics align with specific objectives, encompassing velocity, energy conservation, penetration depth, and penetration volume. The precision of these reward structures is paramount, incentivizing desired behaviors while discouraging undesirable ones.
- Domain Adaptation: The translation of skills acquired in a simulated environment to the tangible world presents a formidable hurdle in robotics. Here, the researchers employ domain adaptation techniques to bridge this chasm. This strategic move empowers robots to apply their parkour prowess in practical settings by adapting skills honed in simulated environments to real-world scenarios.
- Vision as a Keystone Component: In the pursuit of agile parkour performances, vision emerges as an indispensable element. Vision sensors, including depth cameras, furnish robots with crucial insights into their surroundings. This visual acumen equips robots to gauge obstacle attributes, prepare for nimble maneuvers, and make judicious decisions when confronting hurdles.
- Performance: The proposed methodology transcends several baseline approaches and experimental variations. Notably, the two-stage RL approach fortified with soft dynamics constraints propels learning to unprecedented speeds. Robots trained using this methodology achieve markedly higher success rates in tasks demanding exploration, encompassing climbing, leaping, crawling, and tilting. Furthermore, the integration of recurrent neural networks proves indispensable for tasks requiring memory, such as climbing and jumping.
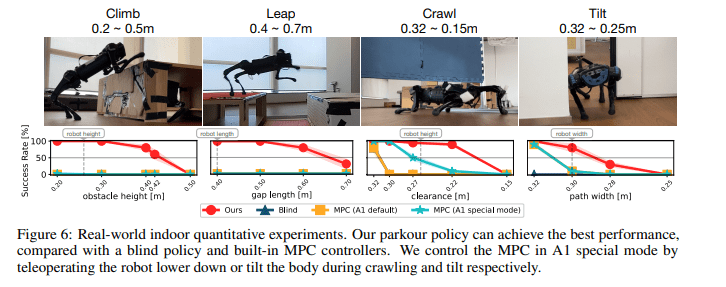
Source: Marktechpost Media Inc.
Conclusion:
This breakthrough in teaching robots agile parkour skills has significant implications for the robotics market. It opens up new possibilities for cost-effective, versatile, and agile robotic solutions that can excel in diverse real-world scenarios. This technology can find applications in industries such as search and rescue, logistics, and entertainment, offering a competitive edge to companies that embrace these advancements.