TL;DR:
- ComCLIP introduces a groundbreaking approach to aligning images and text in the realm of vision-language research.
- It dissects images into subjects, objects, and action sub-images, departing from the conventional monolithic treatment.
- A dynamic evaluation method helps assess the importance of different components for precise alignment.
- ComCLIP creates predicate sub-images that visually represent actions or relationships, enriching model comprehension.
- CLIP text and vision encoders capture the essence of input, and ComCLIP computes similarity scores to weigh component significance.
- The result is an ultimate image embedding that encapsulates the essence of the entire input.
Main AI News:
In the ever-evolving landscape of vision-language research, the harmonious alignment of images and textual descriptions has long been a formidable challenge. This intricate task demands precision in aligning subject, predicate/verb, and object concepts within both visual and textual realms. Its implications ripple across diverse applications, encompassing image retrieval, content comprehension, and beyond. Even with the remarkable strides made by pretrained vision-language models, exemplified by the transformative CLIP, a persistent need for enhanced compositional performance persists. This need arises from the insidious influence of biases and spurious correlations that often infiltrate these models during their intensive training phases. Enter ComCLIP, a groundbreaking solution poised to redefine the paradigm.
In today’s domain of image-text matching, where CLIP has undeniably excelled, the conventional wisdom treats images and text as monolithic entities. While this approach thrives in many scenarios, it falters in tasks demanding intricate compositional nuances. Herein lies the audacious departure of ComCLIP from the established norm. Instead of treating visual and textual inputs as indivisible wholes, ComCLIP dissects images into their elemental constituents: subjects, objects, and action sub-images. This dissection adheres to meticulous encoding rules governing the segmentation process. By embracing this dissection strategy, ComCLIP unveils a profound comprehension of the distinct roles enacted by these diverse components. Furthermore, ComCLIP employs a dynamic evaluation method that gauges the significance of these components in achieving precise compositional alignment. This ingenious approach holds the potential to mitigate the repercussions of biases and spurious correlations endemic to pretrained models, promising superior compositional generalization without necessitating additional training or fine-tuning.
ComCLIP’s methodology orchestrates several pivotal components, converging to surmount the challenges of compositional image and text alignment. It initiates with the utilization of a dense caption module to process the original image, generating dense image captions that zero in on the salient objects within the scene. Simultaneously, the input text sentence undergoes parsing, meticulously extracting entity words and organizing them into a subject-predicate-object format mirroring the structural hierarchy inherent in visual content. The magic transpires when ComCLIP forges a robust alignment between these dense image captions and the extracted entity words. This alignment serves as a bridge, proficiently mapping entity words to their corresponding regions within the image, leveraging the richness of dense captions.
A notable innovation within ComCLIP lies in the creation of predicate sub-images. These sub-images are artfully crafted by amalgamating pertinent object and subject sub-images, mirroring the actions or relationships elucidated in the textual input. The resultant predicate sub-images visually encapsulate the actions or relationships, elevating the model’s comprehension to new heights. Armed with the original sentence, image, parsed words, and sub-images, ComCLIP proceeds to harness the power of CLIP text and vision encoders. These encoders metamorphose textual and visual inputs into embeddings, capturing the essence of each constituent. ComCLIP calculates cosine similarity scores between each image embedding and the corresponding word embeddings, gauging the pertinence and relevance of these embeddings. Subsequently, these scores undergo scrutiny through a softmax layer, empowering the model to judiciously weigh the significance of diverse components. Ultimately, ComCLIP amalgamates these weighted embeddings to unveil the ultimate image embedding—a representation that encapsulates the very essence of the entire input, heralding a new era in compositional image and text alignment.
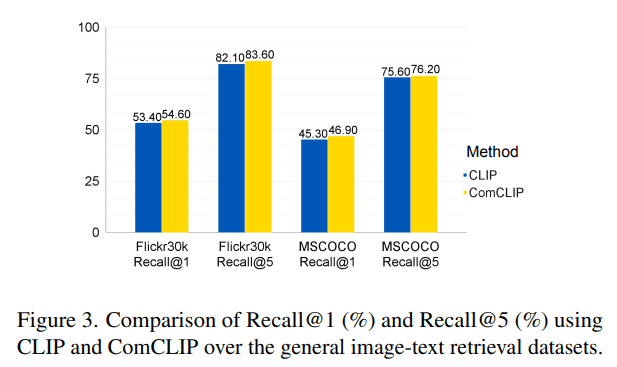
Source: Marktechpost Media Inc.
Conclusion:
ComCLIP’s innovative approach to compositional image and text alignment marks a significant leap forward in the vision-language domain. By dissecting images, creating predicate sub-images, and employing dynamic evaluation, it promises superior compositional alignment, mitigating biases inherent in pretrained models. This technology holds the potential to revolutionize applications like image retrieval and content understanding, creating new opportunities and efficiencies in the market.