TL;DR:
- Cross-Attention Masked Autoencoders (CrossMAE) introduced by UC Berkeley and UCSF researchers for efficient visual data processing.
- Traditional methods face challenges in interpreting complex visual information.
- CrossMAE focuses exclusively on cross-attention for decoding masked patches, simplifying the process.
- The method reduces computational demands while maintaining image quality and task performance.
- Benchmark tests demonstrate that CrossMAE outperforms conventional MAE models in various tasks.
Main AI News:
In the ever-evolving landscape of computer vision, the quest for efficient visual data processing techniques continues to gain momentum. From automated image analysis to the development of intelligent systems, the demand for interpreting complex visual information is more pressing than ever before. While traditional methods have made commendable progress, the pursuit of even more efficient and effective approaches remains a paramount objective.
Within the realm of visual data processing, self-supervised learning and generative modeling techniques have taken center stage. Yet, as groundbreaking as they are, these methods encounter challenges when it comes to efficiently handling complex visual tasks, especially in the context of masked autoencoders (MAE). MAEs are designed to reconstruct images from a limited set of visible patches, a concept that has yielded valuable insights. However, their reliance on self-attention mechanisms places significant demands on computational resources.
Enter Cross-Attention Masked Autoencoders (CrossMAE), a groundbreaking innovation developed by researchers at UC Berkeley and UCSF. This novel framework diverges from the traditional MAE approach by exclusively leveraging cross-attention for decoding masked patches. Unlike conventional MAEs, which utilize a blend of self-attention and cross-attention, CrossMAE simplifies and accelerates the decoding process by concentrating solely on cross-attention between visible and masked tokens.
At the heart of CrossMAE’s efficiency lies its unique decoding mechanism, which relies exclusively on cross-attention between masked and visible tokens. This strategic departure from the need for self-attention within mask tokens marks a significant shift in the MAE paradigm. The CrossMAE decoder is meticulously engineered to focus on decoding a subset of mask tokens, resulting in swifter processing and training. Remarkably, this modification preserves the integrity and quality of reconstructed images and maintains performance in downstream tasks, underscoring CrossMAE’s potential as an efficient alternative to conventional methodologies.
Benchmark tests, including ImageNet classification and COCO instance segmentation, have demonstrated that CrossMAE either matches or surpasses the performance of conventional MAE models, all while significantly reducing decoding computation requirements. Notably, the quality of image reconstruction and the effectiveness in carrying out complex tasks remain unaltered. These findings highlight CrossMAE’s ability to handle intricate visual tasks with enhanced efficiency.
Cross-Attention Masked Autoencoders redefine the approach to masked autoencoders within the realm of computer vision. By prioritizing cross-attention and adopting a partial reconstruction strategy, CrossMAE paves the way for a more efficient method of processing visual data. This research signifies that even small yet innovative changes in approach can yield substantial enhancements in computational efficiency and performance in complex tasks, echoing the evolving landscape of modern computer vision.
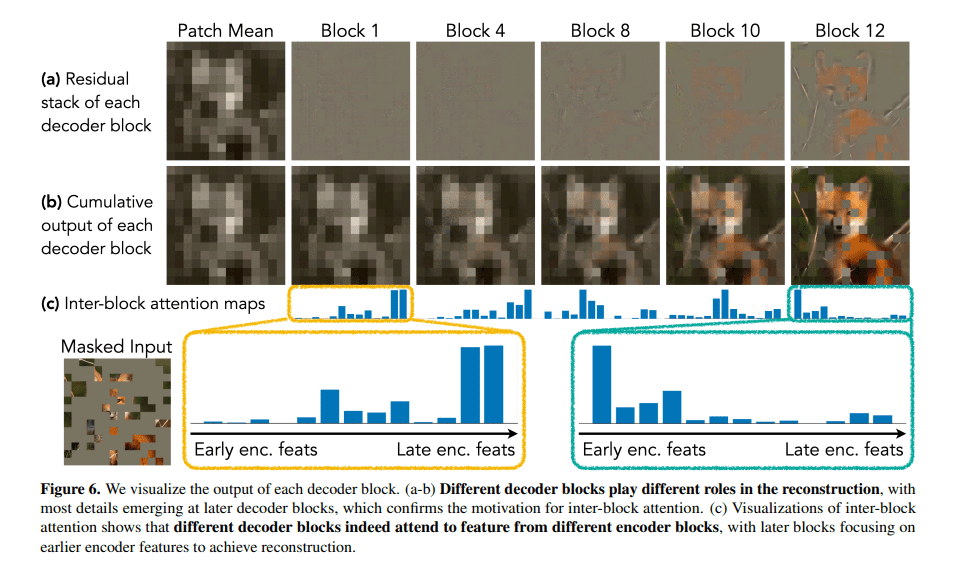
Source: Marktechpost Media Inc.
Conclusion:
The introduction of Cross-Attention Masked Autoencoders (CrossMAE) signifies a significant leap in the efficient processing of visual data. With its ability to outperform traditional models in benchmark tests while reducing computational requirements, CrossMAE has the potential to reshape the market for computer vision solutions. This innovation underscores the value of adopting novel approaches to address complex visual tasks efficiently, which can lead to improved performance and cost-effectiveness in various applications.