TL;DR:
- DeepMind introduces GraphCast, an advanced machine-learning model for weather forecasting.
- GraphCast outperforms supercomputer-dependent NWP models in accuracy and efficiency.
- It uses historical and current weather data to predict future conditions.
- The innovation is vital in the face of climate change and extreme weather events.
- GraphCast excels in forecasting up to 10 days in advance and even severe weather events.
- It surpasses both traditional models and other AI-driven approaches.
Main AI News:
In a remarkable stride forward in the realm of weather forecasting, Google DeepMind proudly presents GraphCast, an innovative machine-learning marvel. This AI powerhouse signifies a momentous leap, promising superior precision and swifter prognostications than the prevailing methods, challenging the reign of conventional numerical weather prediction (NWP) models.
Transforming the Landscape of Weather Prediction
GraphCast operates seamlessly on a standard desktop computer, a stark departure from the supercomputer-dependent NWP models, notorious for their voracious appetite for energy and resources. This AI juggernaut, as showcased in Science on the 14th of November, harnesses historical and current weather data to swiftly forecast forthcoming atmospheric conditions.
This momentous development arrives precisely when precise weather forecasting holds paramount importance, grappling with the global repercussions of climate change and the surge in extreme weather occurrences. Traditional NWP models, while commendable in accuracy, impose an exorbitant demand for computational prowess to decipher the intricate interplay of heat, air, and water vapor within our atmosphere.
GraphCast’s Ascendancy Over Conventional Models
Engineered within DeepMind’s London-based laboratory, GraphCast stands as a product of rigorous training, employing historical global weather records spanning from 1979 to 2017. It harnesses this extensive dataset to unravel the intricate web of correlations between diverse meteorological elements such as temperature, humidity, air pressure, and wind. Its predictive prowess extends up to a remarkable ten days in advance, furnishing forecasts in less than a minute—a task that consumes several hours with the RESolution forecasting system (HRES), an integral component of the ECMWF’s NWP.
Notably, within the troposphere—the atmospheric layer closest to the Earth’s surface—GraphCast outshines the HRES in over 99% of the evaluated 12,000 measurements. It masterfully forecasts five critical weather variables near the Earth’s surface and six atmospheric variables at elevated altitudes. This proficiency extends to predicting severe weather phenomena, encompassing tropical cyclones and radical temperature fluctuations.
A Comparative Edge
GraphCast’s supremacy transcends the confines of traditional models, standing tall even among other AI-driven alternatives. When juxtaposed with Huawei’s Pangu-weather model, GraphCast displayed superior performance in 99% of weather forecasts, as corroborated by a comprehensive Huawei study. However, it’s prudent to acknowledge that future evaluations employing diverse metrics may yield varying results.
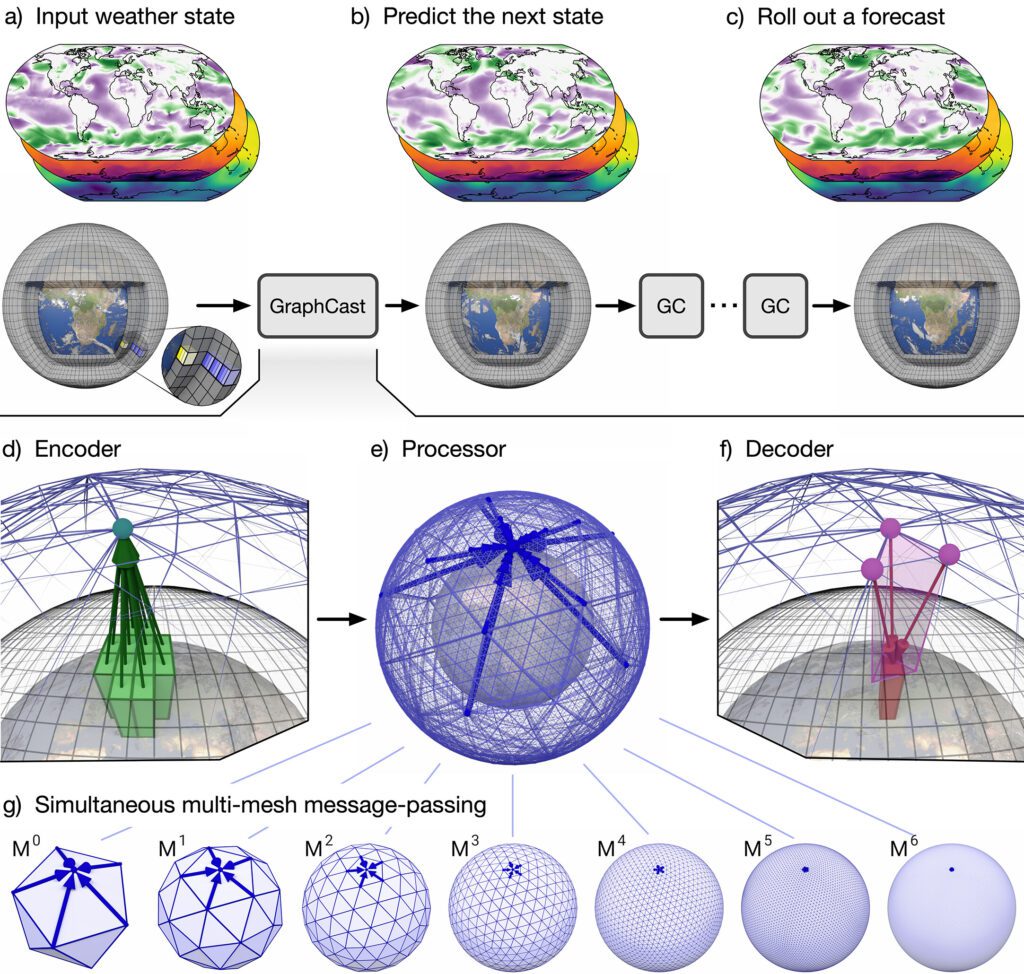
Source: American Association for the Advancement of Science
Conclusion:
DeepMind’s GraphCast presents a game-changing innovation in the field of weather forecasting. Its ability to provide highly accurate and rapid predictions while operating on standard hardware holds the potential to disrupt the market dominated by resource-intensive traditional models. With its demonstrated superiority over both conventional and AI-driven competitors, GraphCast is poised to reshape the landscape of weather prediction, offering new opportunities and efficiencies for various industries reliant on accurate weather forecasts.