TL;DR:
- DenseDiffusion: A novel AI technique for text-to-image generation.
- Challenges with intricate captions and layout manipulation.
- Existing approaches involve training or fine-tuning, requiring resources.
- DenseDiffusion revolutionizes by being training-free.
- Attention modulation process and layout correlation key to DenseDiffusion.
- Demonstrated superiority over compositional diffusion models.
- Business implications: Streamlined image creation, precise translation of textual directives.
Main AI News:
In the fast-evolving realm of artificial intelligence, the fusion of text and image generation has taken tremendous strides, yielding remarkable systems capable of crafting lifelike images from succinct textual descriptions. Despite these breakthroughs, a critical challenge persists—adeptly rendering intricate captions while preserving the distinct attributes of diverse objects within the imagery. This challenge has sparked the emergence of “dense” captioning, a paradigm that employs discrete phrases to detail specific image regions, forming the core of DenseDiffusion’s innovation.
Navigating this intricacy is essential, as users increasingly demand precise manipulation of element arrangements within AI-generated visuals. As businesses harness the power of AI-generated images for marketing, design, and storytelling, the need to faithfully translate textual directives into coherent and compelling visuals has become paramount.
Recent strides in the field have seen the emergence of techniques aimed at endowing users with spatial dominion, achieved through the training or refinement of text-to-image models in congruence with layout conditions. Pioneering endeavors like “Make-aScene” and “Latent Diffusion Models” have pioneered systems built from the ground up, seamlessly blending textual and layout parameters. Concurrent approaches, represented by “SpaText” and “ControlNet,” have ushered in supplementary spatial controls, enhancing existing text-to-image models through meticulous fine-tuning.
However, these techniques often entail computationally intensive training or fine-tuning endeavors. Akin to meticulously sculpting a masterpiece, this iterative process demands time, resources, and expert oversight. Furthermore, the exigency to recalibrate models for every unique user context, domain, or foundational text-to-image framework introduces a substantial impediment.
Responding to these challenges, an ingenious breakthrough emerges—DenseDiffusion. As the moniker suggests, this pioneering methodology dispenses with the shackles of traditional training paradigms and bestows unprecedented prowess upon text-to-image generation. Rather than relying on arduous training iterations, DenseDiffusion capitalizes on the inherent power of its architecture, seamlessly accommodating dense captions and offering unparalleled layout manipulation.
Before delving into the heart of this innovative technique, let’s briefly demystify the inner workings of diffusion models. Picture a canvas gradually coming to life through a sequence of denoising steps, akin to a symphony building to a crescendo. Each step serves as a stroke of brilliance, led by networks that predict and rectify noise, culminating in a resplendent image. Recent refinements in this methodology have optimized the denoising trajectory, preserving efficiency without compromising visual excellence.
Crucially, the cornerstone of cutting-edge diffusion models lies in their self-attention and cross-attention layers. These twin pillars orchestrate the intricate dance between visual elements and textual semantics. Within the self-attention layer, intermediate features not only enhance local details but also sculpt a global coherence by interlinking image tokens that span diverse territories. Simultaneously, the cross-attention layer harmonizes these elements, leveraging the prowess of a CLIP text encoder to infuse textual insights into the visual narrative.
Zooming in on the nucleus of DenseDiffusion’s innovation, we encounter a radical reinterpretation of attention modulation. This paradigm shift is encapsulated in the illustrative diagram below, portraying the synthesis of image layout and attention mechanisms.
The crux of the matter unfolds as the intermediary features of a pre-trained text-to-image diffusion model are dissected. This dissection unveils an intimate nexus between image layout and the orchestration of self-attention and cross-attention maps. Drawing from this profound revelation, a dynamic recalibration of intermediate attention maps transpires, meticulously attuned to layout conditions. This methodology further entails a nuanced consideration of the original attention score spectrum, coupled with precision fine-tuning in alignment with each segment’s spatial domain.
The experimental landscape is illuminated by the authors’ comprehensive demonstrations, spotlighting DenseDiffusion’s prowess to elevate the “Stable Diffusion” model’s performance. Surpassing benchmarks set by a cadre of compositional diffusion models, DenseDiffusion emerges triumphant across various dimensions—be it the intricacies of dense captions, the interplay of text and layout, or the very quality of the generated imagery.
Evidentiary glimpses culled from the study materialize in the imagery below, painting a vivid comparison between DenseDiffusion and the forefront contenders in the AI arena.
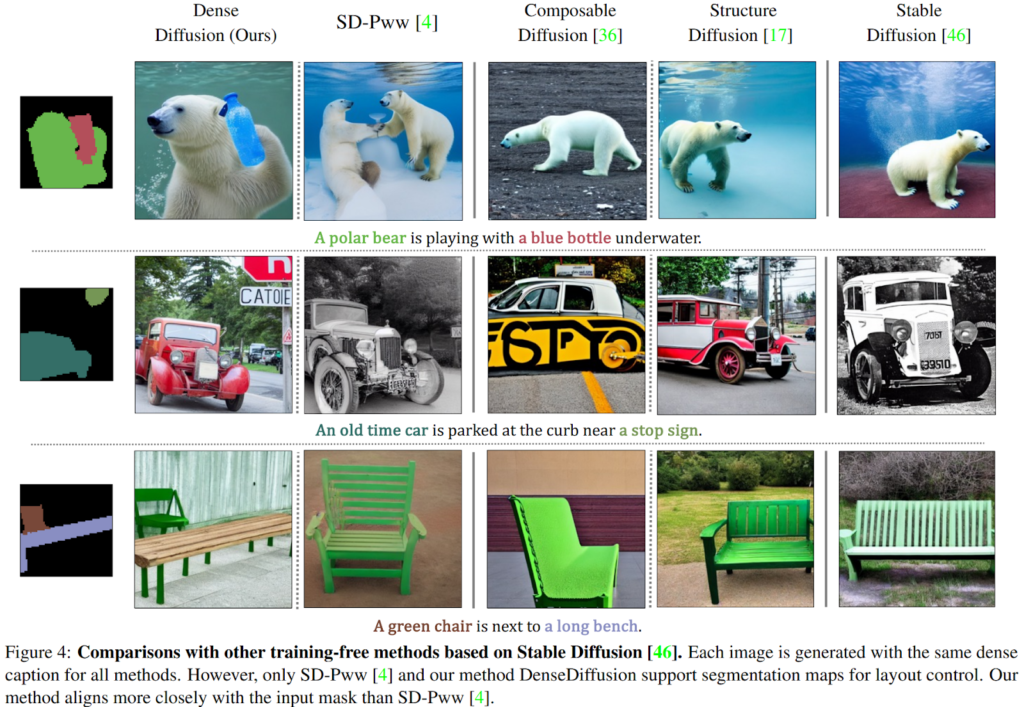
Source: Marktechpost Media Inc.
Conclusion:
The emergence of DenseDiffusion in the field of text-to-image generation marks a pivotal turning point. Its training-free approach addresses challenges with intricate captions and layout manipulation, streamlining the image creation process. This not only enhances efficiency but also ensures a more faithful translation of textual instructions into compelling visuals. For the market, this signifies a leap towards more accessible and accurate AI-generated imagery, opening doors to innovative applications in marketing, design, and communication.