TL;DR:
- DreamSync is an innovative AI framework developed by leading researchers to enhance T2I models.
- It improves alignment and aesthetics in text-to-image synthesis without human annotations or complex model changes.
- The framework leverages Visual Question Answering (VQA) models to evaluate and fine-tune candidate images.
- DreamSync is model-agnostic, bridging the gap between text and image by utilizing vision-language models (VLMs).
- It introduces iterative bootstrapping to label unlabeled data, refine alignment, and enhance model capabilities.
- DreamSync significantly improves faithfulness and aesthetics in T2I models, outperforming baseline methods.
- Its impact extends to various model architectures and diverse settings.
Main AI News:
In the ever-evolving landscape of artificial intelligence, a groundbreaking innovation has emerged, promising to reshape the way we perceive and interact with visual content. Meet DreamSync, the brainchild of visionary researchers from the University of Southern California, the University of Washington, Bar-Ilan University, and Google Research. DreamSync offers a transformative solution to enhance alignment and aesthetic appeal in diffusion-based text-to-image (T2I) models, all without the need for human annotation, complex model alterations, or arduous reinforcement learning processes.
The Core Principle: Bridging Text and Image Seamlessly
DreamSync operates on a simple yet powerful principle: it generates candidate images, evaluates them using cutting-edge Visual Question Answering (VQA) models, and meticulously fine-tunes the text-to-image model. This innovative approach eliminates the traditional hurdles faced by T2I models, marking a pivotal moment in the evolution of AI-driven image synthesis.
Previous studies had introduced VQA models, such as TIFA, to assess T2I generation, but DreamSync takes it several steps further. With an impressive arsenal of 4K prompts and 25K questions, TIFA facilitates evaluation across 12 distinct categories. Complementary techniques like SeeTrue and training-involved methods, including RLHF and training adapters, have addressed T2I alignment, but DreamSync goes beyond these boundaries. It introduces training-free techniques, exemplified by SynGen and StructuralDiffusion, to fine-tune inference for alignment.
A Model-Agnostic Approach: Bridging the Gap
DreamSync’s true innovation lies in its model-agnostic framework, which excels at aligning T2I generation with user intentions and aesthetic aspirations, all without relying on specific architectural choices or labeled data. This visionary framework harnesses the power of vision-language models (VLMs) to identify discrepancies between generated images and input text, effectively closing the gap between text and image.
The DreamSync Process Unveiled
DreamSync’s workflow involves the generation of multiple candidate images from a given prompt, followed by their evaluation for text faithfulness and image aesthetics using two dedicated VLMs. The image that best aligns with the intended output, as determined by VLM feedback, is then used to fine-tune the T2I model. This iterative process continues until convergence is achieved, resulting in images that seamlessly match the user’s vision.
Iterative Bootstrapping: A Novel Approach
Additionally, DreamSync introduces the concept of iterative bootstrapping, utilizing VLMs as teacher models to label unlabeled data for T2I model training. This innovative approach not only refines the alignment but also leverages untapped data resources to further enhance the model’s capabilities.
Elevating T2I Models: Real-World Results
The impact of DreamSync on T2I models is undeniable. When applied to SDXL and SD v1.4 T2I models, DreamSync demonstrates its prowess with three SDXL iterations resulting in an impressive 1.7 and 3.7-point improvement in faithfulness on TIFA. Visual aesthetics also received a substantial boost, improving by 3.4 points. The application of DreamSync to SD v1.4 yields a 1.0-point faithfulness improvement and a 1.7-point absolute score increase on TIFA, with aesthetics improving by 0.3 points. In a head-to-head comparison, DreamSync outperforms SDXL in alignment, producing images with more relevant components and 3.4 more correct answers. It achieves superior textual faithfulness without compromising visual appearance on TIFA and DSG benchmarks, showcasing consistent progress through iterations.
A Bright Future Ahead
In conclusion, DreamSync emerges as a versatile framework that has been rigorously evaluated on challenging T2I benchmarks, showcasing substantial improvements in alignment and visual appeal across diverse settings. With the incorporation of dual feedback from vision-language models and validation through human ratings and preference prediction models, DreamSync sets a new standard for T2I synthesis.
As we look to the future, DreamSync is poised for further enhancements, including the integration of detailed annotations like bounding boxes to identify misalignments. Tailoring prompts at each iteration aim to target specific improvements in text-to-image synthesis, while the exploration of linguistic structure and attention maps strives to enhance attribute-object binding. The training of reward models with human feedback promises to align generated images even more closely with user intent. Furthermore, DreamSync’s application to a wide array of model architectures and ongoing studies in diverse settings promise an exciting journey ahead. DreamSync is not just a framework; it’s a catalyst for innovation in the realm of AI-driven image synthesis.
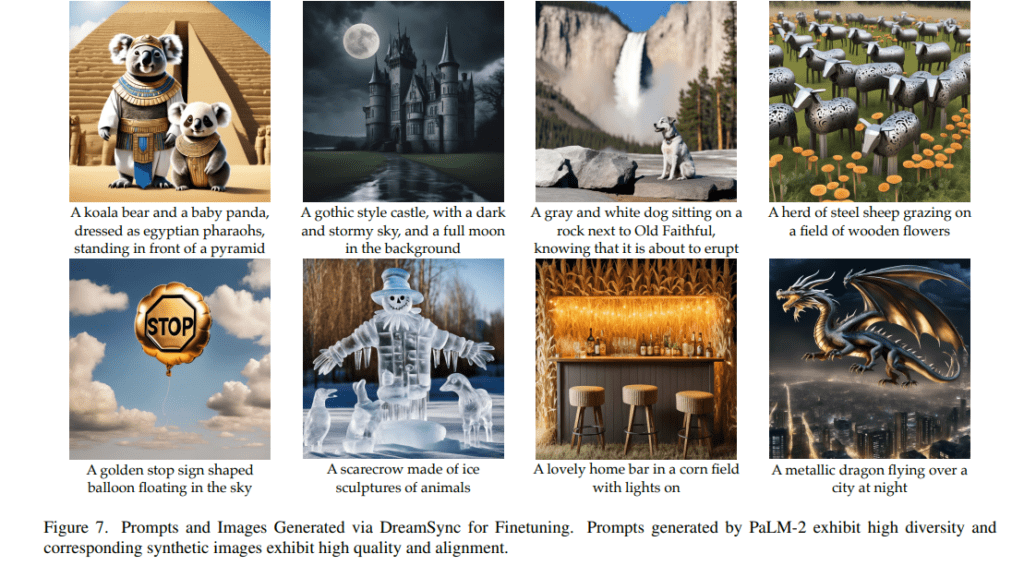
Source: Marktechpost Media Inc.
Conclusion:
DreamSync’s emergence represents a game-changing development in the T2I synthesis market. This innovative framework’s ability to enhance alignment and aesthetics while remaining model-agnostic positions it as a valuable asset for businesses looking to leverage AI-driven image synthesis. With potential applications across various industries, DreamSync sets a new standard for T2I models, offering significant improvements and unlocking new possibilities for visual content generation.