TL;DR:
- LLMs have greatly impacted AI with their language-processing capabilities.
- The challenge is efficiently utilizing external tools due to inconsistent documentation.
- “EASY TOOL” by leading institutions simplifies and standardizes tool documentation for LLMs.
- It streamlines documentation, focusing on essential tool functionalities.
- The framework also provides detailed instructions on tool usage.
- “EASY TOOL” improves LLM performance, reducing token consumption, and enabling adaptability.
Main AI News:
In the realm of artificial intelligence, Large Language Models (LLMs) have undeniably revolutionized the landscape, boasting unparalleled language-processing capabilities. They have seamlessly integrated into applications ranging from automated customer service to the creation of imaginative content. However, a significant hurdle has emerged in their quest for efficiency – the challenge of harnessing external tools effectively.
This challenge arises from the inherent inconsistencies, redundancies, and incompleteness often found in tool documentation. These limitations create a roadblock, preventing LLMs from fully capitalizing on external tools, a crucial component in broadening their functional horizons. Traditional approaches, such as fine-tuning models or using prompt-based methods, have attempted to address this issue. Yet, the quality of available documentation remains a stumbling block, leading to incorrect tool usage and suboptimal task execution.
Enter “EASY TOOL,” a pioneering framework introduced by researchers from Fudan University, Microsoft Research Asia, and Zhejiang University. This game-changing framework aims to simplify and standardize tool documentation explicitly for LLMs, paving the way for enhanced practicality across various domains. “EASY TOOL” employs a systematic approach to restructure extensive tool documentation from diverse sources, with a keen focus on distilling the core essence while eliminating extraneous details. This streamlined methodology clarifies the tools’ functionalities, making them more accessible and comprehensible for LLMs.
The inner workings of “EASY TOOL” encompass a two-fold strategy. Firstly, it meticulously reorganizes the original tool documentation by purging irrelevant information, retaining only the vital functionalities of each tool. This step is pivotal in spotlighting the fundamental purpose and utility of the tools without the encumbrance of superfluous data. Secondly, “EASY TOOL” enriches this concise documentation with structured, comprehensive instructions on tool utilization. This includes an exhaustive outline of both mandatory and optional parameters for each tool, complemented by practical examples and demonstrations. This dual-pronged approach not only ensures precise tool invocation by LLMs but also equips them with the capability to select and apply these tools adeptly in diverse scenarios.
The implementation of “EASY TOOL” has yielded remarkable dividends in the performance of LLM-based agents across real-world applications. One of its most striking achievements is the substantial reduction in token consumption, directly translating into more efficient processing and response generation by LLMs. Furthermore, this framework has elevated the overall proficiency of LLMs in tool utilization across a wide spectrum of tasks. Impressively, it has empowered these models to operate effectively even in the absence of tool documentation, highlighting the framework’s adaptability and versatility in varying contexts.
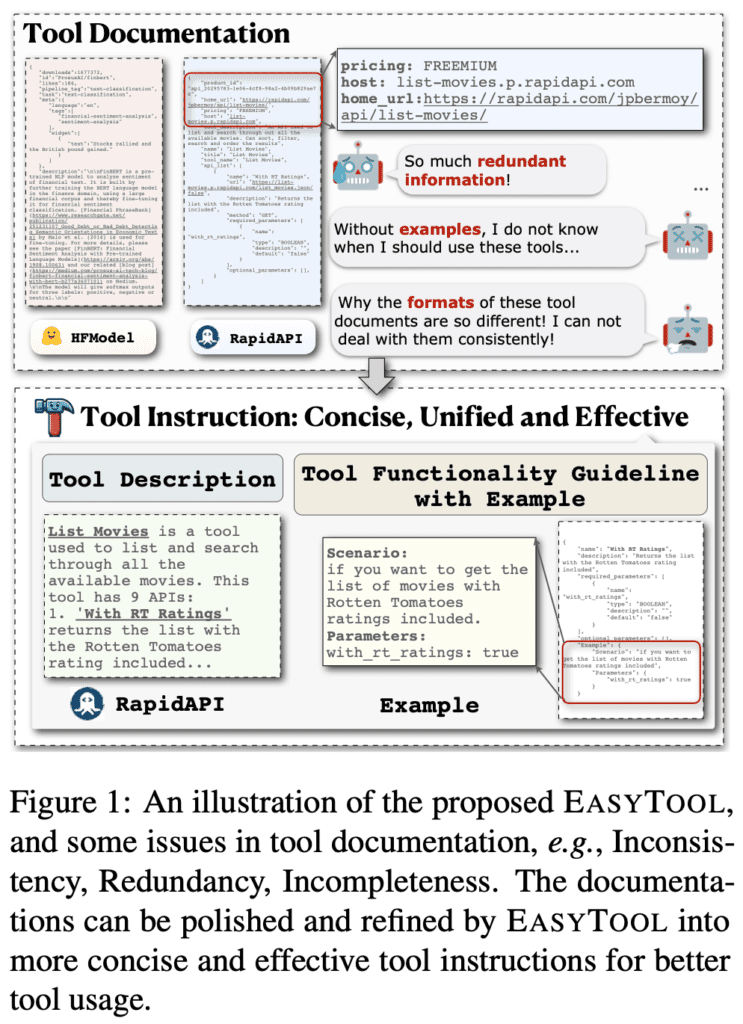
Source: Marktechpost Media Inc.
Conclusion:
The introduction of “EASY TOOL” represents a significant leap forward in the realm of Large Language Models (LLMs). This framework, developed by esteemed institutions, addresses the pressing issue of inefficient external tool utilization caused by inconsistent documentation. With its streamlined approach and comprehensive tool instructions, “EASY TOOL” is poised to enhance LLM performance across various sectors, ultimately shaping the market by improving AI efficiency and productivity.