TL;DR:
- Probabilistic diffusion models are reshaping computer vision research.
- They introduce innovative generative capabilities compared to VAEs, GANs, and other models.
- Diffusion models employ a unique process involving both diffusion and denoising phases.
- A study by S-Lab and Nanyang Technological University explores the denoising process.
- Insights from the Fourier domain reveal modulation of low and high-frequency components during denoising.
- The U-Net architecture plays a crucial role in denoising, but high-frequency features can pose challenges.
- “FreeU” is a novel approach enhancing generated sample quality without additional training.
- It introduces specialized modulation factors to balance denoising contributions.
- FreeU seamlessly integrates with existing diffusion models, improving various applications.
- Empirical evaluations demonstrate significant quality enhancements in generated outputs.
Main AI News:
Probabilistic diffusion models, a prominent category within the realm of generative models, have emerged as a pivotal focal point in contemporary research, particularly within the sphere of computer vision. Unlike their generative counterparts, such as Variational Autoencoders (VAEs), Generative Adversarial Networks (GANs), and vector-quantized methods, diffusion models present an inventive approach to generative capabilities. These models harness a steadfast Markov chain to navigate the latent space, facilitating intricate mappings that elegantly capture latent structural complexities nestled within datasets. Their impressive generative prowess, spanning from remarkable detail to diverse exemplars, has heralded groundbreaking advancements across myriad computer vision applications, including but not limited to image synthesis, image manipulation, image-to-image translation, and text-to-video synthesis.
The architectural essence of diffusion models comprises two cardinal components: the diffusion process and the denoising process. Throughout the diffusion process, Gaussian noise gradually infiltrates the input data, metamorphosing it into an almost pristine state of Gaussian noise. Conversely, the denoising process endeavors to resurrect the original input data from its noisy counterpart through a sequence of learned inverse diffusion operations. Traditionally, a U-Net framework is harnessed to iteratively predict noise removal at each denoising juncture. While existing research predominantly revolves around employing pre-trained diffusion U-Nets for downstream tasks, the inner workings of the diffusion U-Net have remained relatively unexplored.
A collaborative study conducted by the esteemed S-Lab and Nanyang Technological University takes an unconventional path by investigating the efficacy of the diffusion U-Net within the denoising process. In a quest for a deeper comprehension of this process, the researchers embark on a paradigm shift towards the Fourier domain to unravel the inner workings of diffusion models—a hitherto underexplored territory.
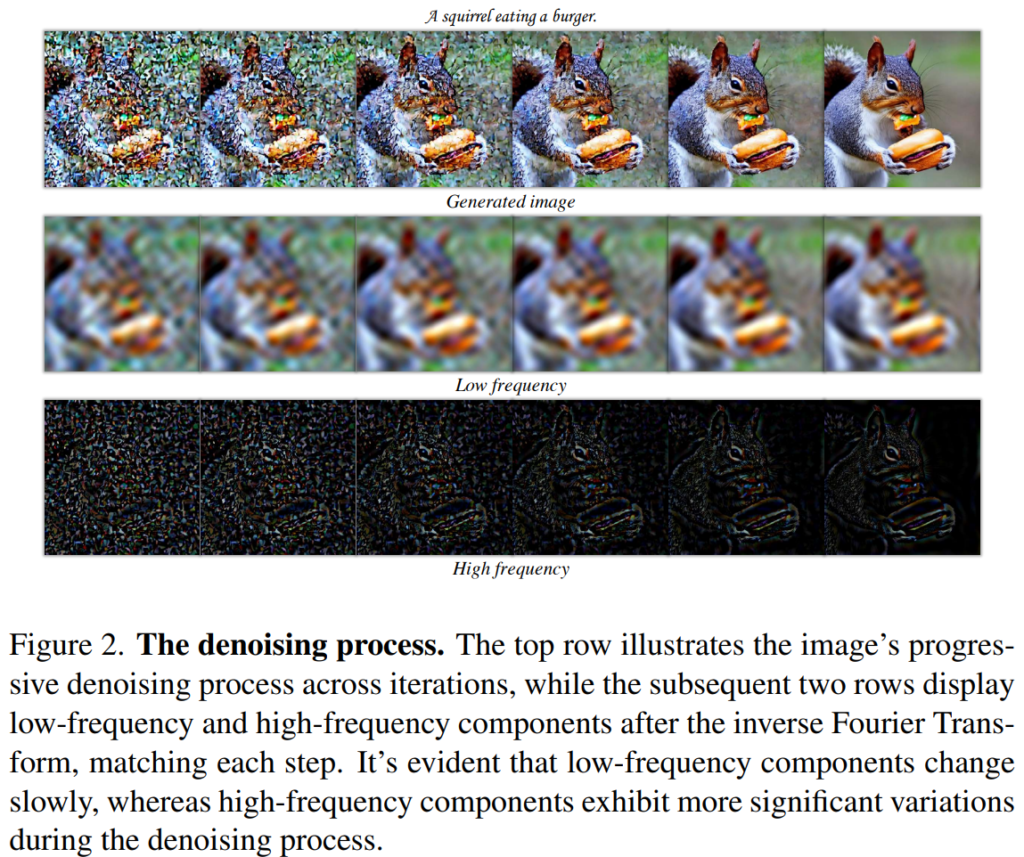
Source: Marktechpost Media Inc.
The diagram above elegantly portrays the gradual denoising process in the top row, showcasing the evolution of images through successive iterations. In contrast, the subsequent two rows unveil the corresponding low-frequency and high-frequency spatial domain information post-inverse Fourier Transform, corresponding to each respective stage. This graphic revelation unveils a gradual modulation of low-frequency components, signifying a subdued rate of change, while high-frequency elements exhibit more pronounced dynamics during the denoising journey. The rationale behind these findings is intuitive: low-frequency components inherently encapsulate an image’s global structure and characteristics, encompassing overarching layouts and seamless color gradients. Alarming transformations to these elements during denoising are often unwelcome, as they can fundamentally alter an image’s essence. Conversely, high-frequency elements capture swift transitions in images, including edges and textures, and remain highly sensitive to noise. Ergo, the denoising endeavor must expunge noise while preserving these intricate facets.
Armed with these insights into the low-frequency and high-frequency components during denoising, the investigation naturally extends to delineate the specific contributions of the U-Net architecture within the diffusion framework. At each stage of the U-Net decoder, a fusion occurs between skip features from the skip connections and backbone features. The study’s findings underscore the pivotal role played by the primary U-Net backbone in denoising, while the skip connections introduce high-frequency features into the decoder module, thereby aiding in the recovery of finely detailed semantic information. Nevertheless, this infusion of high-frequency features can inadvertently dilute the intrinsic denoising capabilities of the backbone during the inference phase, potentially leading to the generation of aberrant image details.
In light of this revelatory insight, the researchers proffer an innovative solution christened “FreeU.” This cutting-edge approach elevates the quality of generated samples without necessitating additional computational overhead in the form of training or fine-tuning. An overview of the FreeU framework is elucidated below.
During the inference phase, FreeU introduces two specialized modulation factors to calibrate the contributions of features emanating from the primary backbone and skip connections within the U-Net architecture. The first factor, denominated “backbone feature factors,” serves to amplify the feature maps of the primary backbone, thereby bolstering the denoising process. However, it is discerned that the inclusion of these backbone feature scaling factors, while conferring substantial improvements, can at times inadvertently result in an undesired over-smoothing of textures. To ameliorate this concern, the second factor, dubbed “skip feature scaling factors,” is introduced to mitigate the risk of over-smoothing textures.
The FreeU framework showcases remarkable adaptability when integrated seamlessly with existing diffusion models, extending its transformative influence to applications encompassing text-to-image and text-to-video synthesis. A comprehensive empirical assessment of this pioneering approach is meticulously undertaken, with foundational models like Stable Diffusion, DreamBooth, ReVersion, ModelScope, and Rerender serving as benchmarks for comparisons. When FreeU assumes its role during the inference phase, these models resoundingly exhibit a discernible augmentation in the quality of the generated outputs. The visual representation depicted in the accompanying illustration unequivocally attests to FreeU’s prowess in substantially enhancing both intricate details and the overall visual fidelity of the generated imagery.
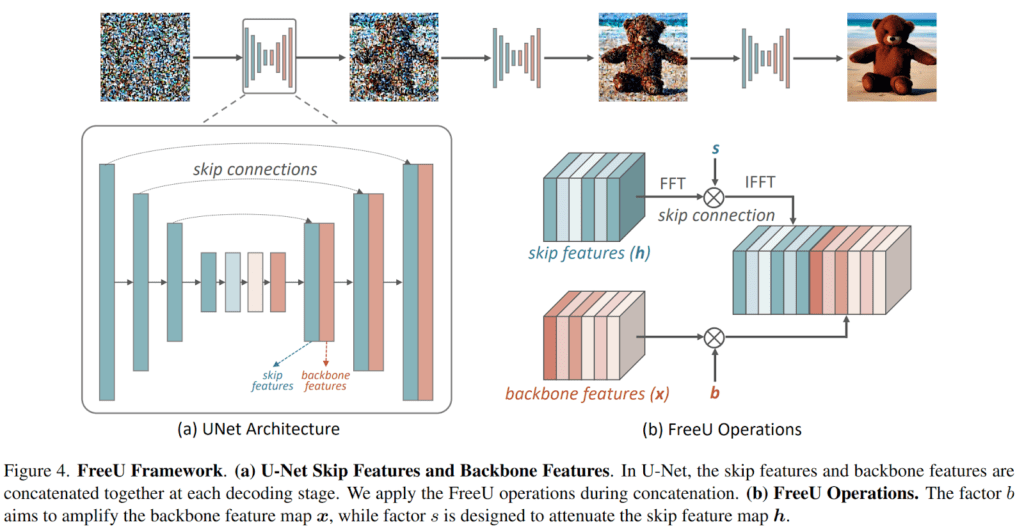
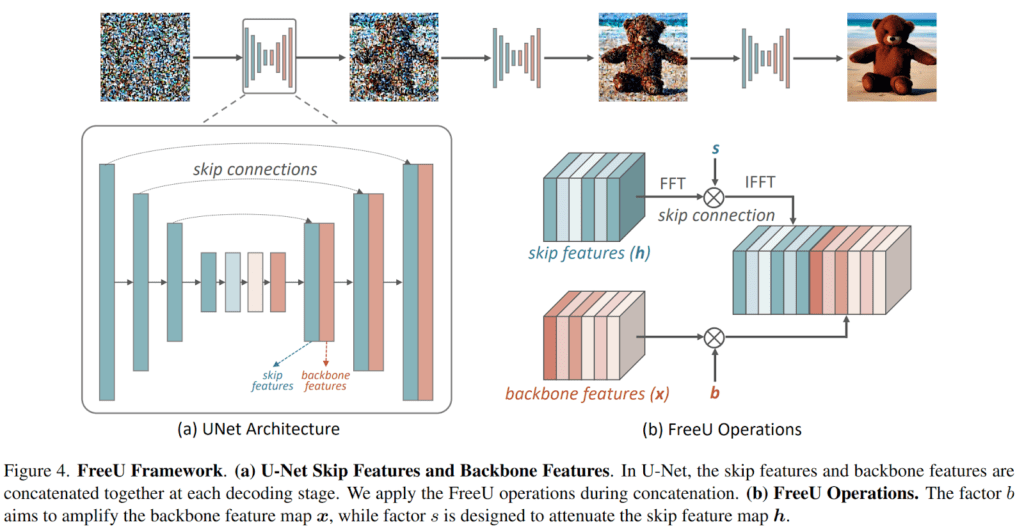
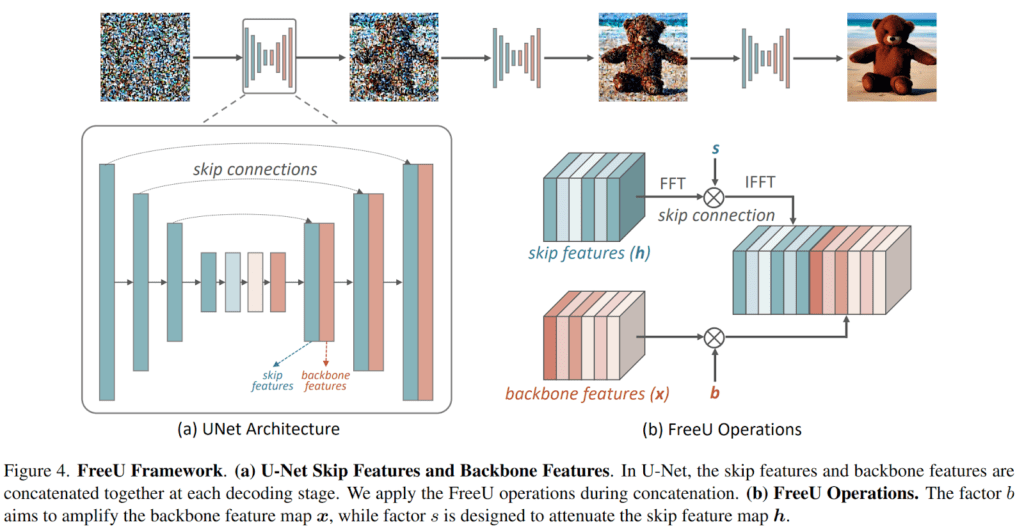
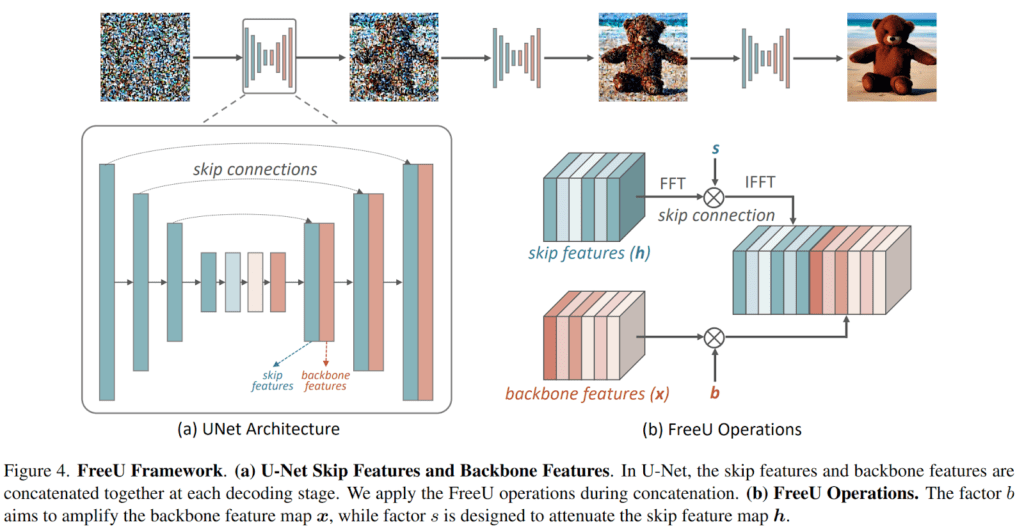
Source: Marktechpost Media Inc.
Conclusion:
FreeU’s innovative approach to enhancing generative models holds substantial promise for the market. It addresses critical challenges in maintaining image quality during denoising, which is of paramount importance in industries like computer vision, image synthesis, and more. By seamlessly integrating with existing diffusion models, FreeU offers a practical and efficient solution to elevate the quality of generated content, potentially revolutionizing the way businesses approach image and video generation tasks. This advancement could lead to improved product quality and user experiences across a wide range of applications.