TL;DR:
- Qualcomm AI Research introduces LRR, a multi-modal LM paradigm.
- LRR focuses on improving low-level visual skills and complex visual reasoning.
- The model employs a two-stream video encoder and a “Look, Remember, Reason” approach.
- It outperforms task-specific methods and leads the STAR challenge leaderboard.
- LRR adapts effectively across datasets like ACRE, CATER, and Something-Else.
Main AI News:
In the realm of multi-modal language models (LMs), the pursuit of mastering intricate visual reasoning tasks has been a persistent challenge. Tasks such as the nuanced recognition of compositional actions within videos demand a fusion of low-level object motion analysis and high-level spatiotemporal reasoning. While multi-modal LMs have achieved excellence in various domains, their prowess in tasks necessitating meticulous attention to fine-grained details, coupled with advanced rationale, remains relatively uncharted. This discrepancy highlights a significant opportunity for growth and innovation in their capabilities.
Innovations in the field of multi-modal LMs are steadily advancing, with the emergence of auto-regressive models and specialized adapters for visual processing. Prominent image-based models such as Pix2seq, ViperGPT, VisProg, Chameleon, PaLM-E, LLaMA-Adapter, FROMAGe, InstructBLIP, Qwen-VL, and Kosmos-2 are pushing the boundaries of what’s possible. Simultaneously, video-based models like Video-ChatGPT, VideoChat, Valley, and Flamingo have captured the industry’s attention. One of the recent focal points is spatiotemporal video grounding, a novel approach to object localization in media through the interpretation of linguistic cues. Attention-based models are at the forefront of this research, employing advanced techniques like multi-hop feature modulation and cascaded networks to elevate their visual reasoning capabilities.
Enter Qualcomm AI Research, with a pioneering multi-modal LM designed to enhance low-level visual skills. This innovative model is trained comprehensively on tasks encompassing object detection and tracking, delivering a powerful two-stream video encoder equipped with spatiotemporal attention mechanisms. Following a disciplined “Look, Remember, Reason” process, this model represents a leap forward in visual reasoning capabilities.
The research undertaken by Qualcomm AI Research is centered on the enhancement of multi-modal LMs and is grounded in real-world datasets such as ACRE, CATER, and STAR. During the training phase, surrogate tasks involving object recognition, re-identification, and the identification of the state of the blicket machine are introduced with a 30% probability after each contextual trial or query. Remarkably, this model achieves its remarkable performance with fewer parameters, utilizing the OPT-125M and OPT-1.3B architectures. The training process continues until convergence, employing a batch size of 4 and harnessing the power of the AdamW optimizer.
As of January 2024, the LRR framework proudly leads the STAR challenge leaderboard, underscoring its unparalleled prowess in video reasoning. Its adaptability and proficiency in processing low-level visual cues shine through as it excels across a diverse array of datasets including ACRE, CATER, and Something-Else. The LRR model’s ability to be trained end-to-end and outperform task-specific methodologies further solidifies its position as a game-changer in the realm of video reasoning.
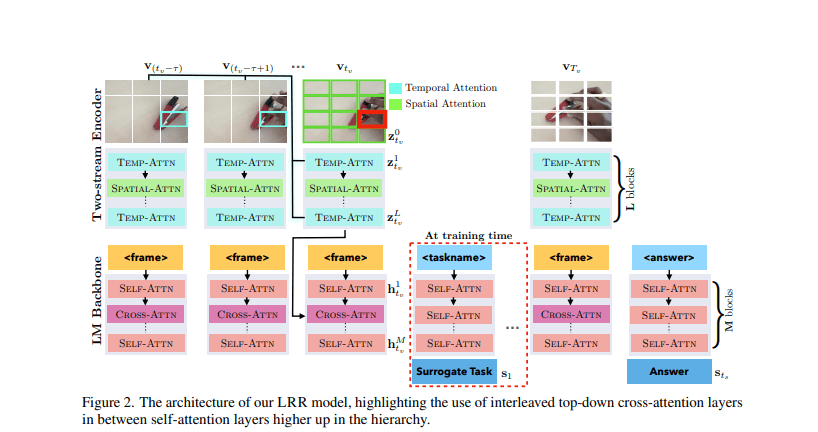
Source: Marktechpost Media Inc.
Conclusion:
Qualcomm’s LRR paradigm represents a significant leap in multi-modal language models’ ability to excel in complex visual reasoning tasks. With a focus on enhanced visual processing and a leading position in the STAR challenge, Qualcomm’s innovation is poised to disrupt and elevate the market for multi-modal LMs, catering to the growing demand for advanced visual reasoning capabilities.