- Vision-language models (VLMs) are pivotal for processing visual and textual inputs in AI.
- Challenges persist in integrating and interpreting visual and linguistic data effectively.
- ALLaVA introduces a novel approach by leveraging synthetic data from GPT-4V to enhance VLM efficiency.
- The method focuses on meticulous captioning and question generation, yielding two expansive synthetic datasets: ALLaVA-Caption and ALLaVA-Instruct.
- Empirical evaluations demonstrate competitive performance across 12 benchmarks, showcasing efficiency and efficacy.
- The success of ALLaVA highlights the potential of high-quality synthetic data in training more efficient VLMs.
Main AI News:
In the realm of artificial intelligence, vision-language models (VLMs) stand out for their ability to comprehend and process both visual and textual inputs, mirroring the human capacity to perceive and understand the world. This convergence of vision and language is pivotal for a multitude of applications, spanning from automated image captioning to intricate scene analysis and interaction.
Yet, a significant challenge persists: the development of models capable of seamlessly integrating and deciphering visual and linguistic data, a task that remains inherently complex. This complexity is exacerbated by the necessity for models to discern individual components within an image or text and grasp the subtle interplay between them.
Traditional methods for aligning image-text data in language models often rely on caption data. However, these captions frequently lack depth and granularity, resulting in noisy signals that impede alignment. Moreover, the current volume of aligned data is limited, posing difficulties in learning extensive visual knowledge across diverse domains. Expanding the breadth of aligned data from various sources is imperative for fostering a nuanced understanding of less mainstream visual concepts.
Addressing this challenge head-on, a collaborative team from the Shenzhen Research Institute of Big Data and the Chinese University of Hong Kong introduces a groundbreaking approach to enhancing vision-language models. Their innovation, known as A Lite Language and Vision Assistant (ALLaVA), harnesses synthetic data generated by GPT-4V to train a streamlined version of large vision-language models (LVLMs). This methodology aims to deliver a resource-efficient solution without sacrificing performance quality.
By leveraging the capabilities of GPT-4V, ALLaVA synthesizes data using a captioning-then-QA technique, with a focus on images sourced from Vision-FLAN and LAION platforms. This meticulous process entails detailed captioning, the generation of intricate questions to facilitate rigorous reasoning and the provision of comprehensive answers. Upholding ethical standards, the generation process avoids biased or inappropriate content. The resultant output comprises two expansive synthetic datasets: ALLaVA-Caption and ALLaVA-Instruct, encompassing captions, visual questions and answers (VQAs), and meticulously crafted instructions. The architectural framework adopts CLIP-ViT-L/14@336 for vision encoding and Phi2 2.7B as the language model backbone, ensuring robust performance across a spectrum of benchmarks.
In empirical evaluations, the model demonstrates competitive performance across 12 benchmarks, even when compared to LVLMs with significantly larger architectures, underscoring its efficiency and efficacy. Ablation analysis further validates the significance of training the model with ALLaVA-Caption-4V and ALLaVA-Instruct-4V datasets, which markedly enhance performance on benchmarks. Notably, the Vision-FLAN questions, while relatively straightforward, prioritize the enhancement of fundamental abilities over complex reasoning.
The success achieved by ALLaVA serves as a testament to the potential of leveraging high-quality synthetic data to train more efficient and effective vision-language models, thereby democratizing access to advanced AI technologies.
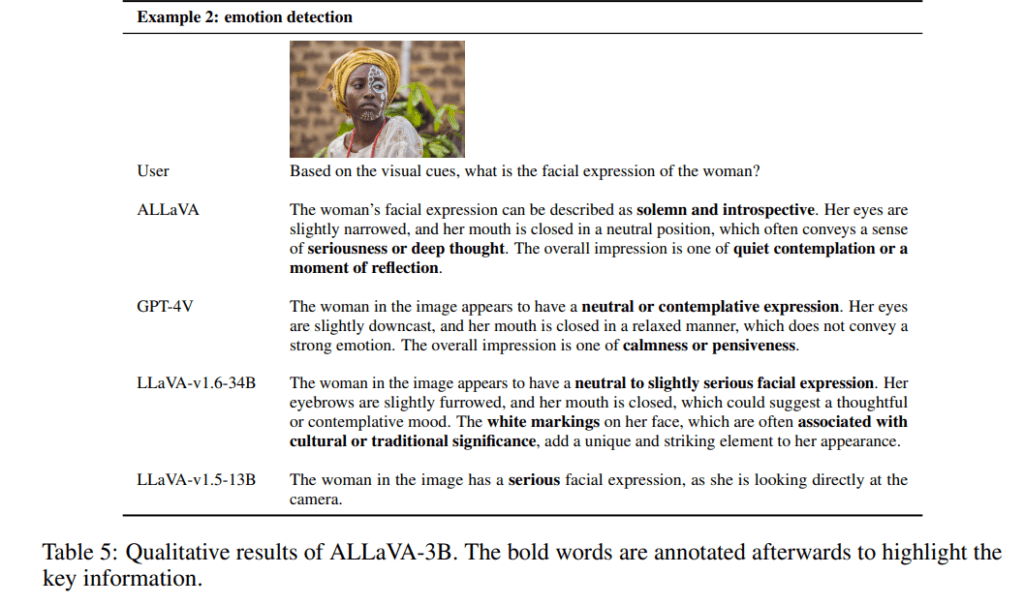
Source: Marktechpost Media Inc.
Conclusion:
The emergence of ALLaVA signifies a significant advancement in the realm of vision-language models. By harnessing synthetic data, it offers a promising avenue for improving efficiency and effectiveness in AI technologies. This innovation is poised to reshape the market landscape, making advanced vision-language capabilities more accessible and impactful across various industries. Businesses should take note of this development and consider its implications for future AI initiatives and investments.