TL;DR:
- Movies are powerful mediums for conveying emotions and stories.
- AI needs to understand character emotions for narrative comprehension.
- EmoTX utilizes MovieGraphs annotations to predict emotional states.
- Historical and modern explorations of emotions contribute to this field.
- EmoTX introduces 26 emotion clusters and continuous dimensions.
- Contextual modalities like dialogues and scenes enhance emotion prediction.
- EmoTX employs a Transformer-based approach for accurate emotion identification.
Main AI News:
Cinematic creations stand as some of the most profound conduits for stories and sentiments. Take, for instance, “The Pursuit of Happyness,” where the main character traverses a labyrinth of emotions—plummeting through heart-wrenching lows like heartbreak and homelessness, yet soaring amidst euphoric highs upon seizing a coveted career milestone. These potent sentiments captivate the audience, establishing an empathetic connection with the character’s expedition. In the realm of artificial intelligence (AI), unraveling such intricate narratives calls for machines to meticulously track the evolution of characters’ emotions and psychological states across the storyline. This ambitious goal materializes by harnessing insights from MovieGraphs, harnessing training paradigms to scrutinize scenes, dissect dialogues, and prognosticate the ebb and flow of characters’ emotional and mental realms.
The domain of emotions has been an inexhaustible muse across history: from Cicero’s ancient Roman taxonomy to modern-day neuroscientific inquiries, the essence of emotions has perennially enthralled humanity’s curiosity. Psychologists have enriched this terrain by introducing frameworks like Plutchik’s emotive wheel and Ekman’s notion of universally recognizable facial expressions, diversifying the theoretical landscape. Moreover, affective emotions gracefully cascade into cognitive, behavioral, and bodily states, encapsulating a holistic experiential spectrum.
In a recent endeavor, the Emotic initiative unfurled a panorama of 26 distinct clusters of emotion labels tailored for processing visual content. This avant-garde project champions a multi-label framework, acknowledging that an image can eloquently convey an ensemble of emotions concurrently—harboring tranquility and engagement in the same visual breath. Departing from the conventional pigeonholing approach, the study ushers in three continuous dimensions—valence, arousal, and dominance—infusing dynamic dynamism into emotional appraisal.
The expedition to achieve pinpoint emotional prognostication must traverse a landscape of contextual modalities, ensuring an exhaustive palette of emotions. Pioneering avenues in multimodal emotion discernment encompass Emotion Recognition in Conversations (ERC), an enterprise that entails categorizing emotions stitched within each exchange of dialogue. Conversely, an alternative path involves predicting a singular valence-activity score, succinctly encapsulating the emotional tenor within compact fragments of movie clips.
Delving into the granularity of a cinematic scene demands orchestrating a symphony of shots, collectively weaving a sub-narrative within a specific backdrop, housing a delineated cast, and unfolding over the fleeting span of 30 to 60 seconds. These vignettes bestow a temporal canvas more expansive than solitary dialogues or film snippets. The aim unfurls as a tapestry of forecasting characters’ emotions and psychological realms within the scene’s crucible, amalgamating labels at the scene level. Given the extended chronology, this pursuit naturally converges towards a multi-label classification methodology—characters might eloquently channel diverse emotions concurrently (picture curiosity intertwining with confusion) or navigate transitions as they intertwine in interpersonal exchanges (for instance, the metamorphosis from distress to tranquility).
Intriguingly, while emotions stand as prominent constituents of cognitive landscapes, this inquiry accents a distinction between overtly expressed emotions, manifesting through a character’s countenance (e.g., astonishment, melancholy, ire), and latent mental states, clandestinely surfacing solely through interactions or dialogues (e.g., graciousness, resolve, self-assuredness, benevolence). The authors cogitate that effectively categorizing within an expansive emotional lexicon necessitates a comprehensive mosaic of contextual cues. Hence emerges EmoTx—a conceptual jewel—a model that seamlessly interlaces video frames, dialogic utterances, and character apparitions into its cognitive tapestry.
EmoTx pivots upon the sinews of a Transformer-based architecture, casting a discerning gaze upon emotions at both the individual character and cinematic scene strata. The overture entails an inaugural dance of video pre-processing and feature extraction, an orchestration that summons forth salient representations from the raw data expanse. These facets span video sequences, countenances of characters, and textual attributes. Tokens, nuanced by modality, character enumeration, and temporal context, befittingly encode the gestalt. Furthermore, these tokens metamorphose into classifiers, anchoring emotions to specific characters or scenes. The synthesized tokens, once poised, elegantly converge through linear strata, cascading into a Transformer encoder—a sanctum fostering the confluence of diverse modalities. The classification facet of this opus draws inspiration from precedent studies on transformative multi-label categorization.
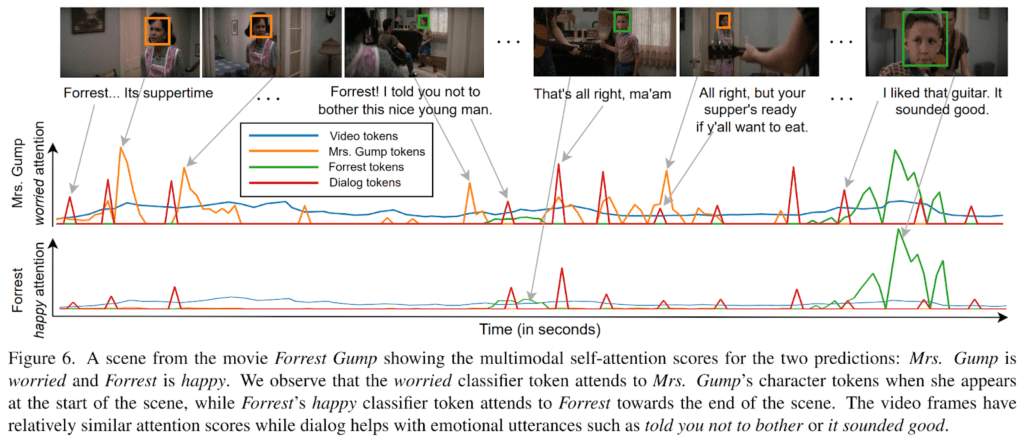
Source: Marktechpost Media Inc.
Conclusion:
EmoTX’s innovative AI framework presents a breakthrough in understanding emotions within cinematic narratives. This advancement has far-reaching implications for the market, potentially revolutionizing areas such as content recommendation systems, personalized advertising, and audience engagement strategies. Businesses in the entertainment industry can leverage EmoTX to enhance user experiences and tailor content delivery, leading to increased customer satisfaction and retention. Additionally, EmoTX’s multimodal approach sets the stage for advancements in sentiment analysis across diverse sectors, from market research to customer feedback analysis, enabling companies to gain deeper insights into consumer preferences and sentiments.