TL;DR:
- AI’s progress in the past decade has been remarkable, but concerns over its lack of ‘explainability’ persist.
- A joint study by the University of Chicago and York University reveals significant disparities in how neural networks process visual information compared to human cognition.
- The study demonstrates the existence of ‘Frankenstein’ images, which AI can identify but are problematic to human observers.
- Neural networks tend to take ‘shortcuts’ when handling complex recognition tasks, leading to potential failures in real-world AI applications.
- To achieve human-like configurable sensitivity, AI models must be trained to address a broader range of object tasks beyond category recognition.
Main AI News:
In the past decade, Artificial Intelligence (AI) has achieved remarkable progress, revolutionizing industries with applications like autonomous driving and medical diagnoses. However, the lack of ‘explainability’ in AI algorithms has hindered the complete trust and relinquishment of control to these systems.
A groundbreaking joint study conducted by the esteemed University of Chicago and York University delves deeper into the concerns surrounding AI, especially in mission-critical scenarios. The study has shed light on the potential justifiability of these worries. The core finding reveals that while neural networks can be trained to ‘see,’ their perception and processing of visual information differ significantly from human cognition.
Throughout the research, intriguing ‘Frankenstein’ images were generated. To the human eye, these images were immediately recognized as problematic. However, the neural network could effortlessly identify and process them. This stark discrepancy between human perception and AI’s interpretation arises due to the neural network’s inclination towards ‘shortcuts’ when tackling complex recognition tasks.
Professor James Elder, a distinguished academic at York University, elucidates the concept of ‘Frankensteins’: objects disassembled and reassembled in incorrect configurations. Consequently, they exhibit all the correct local features but in the wrong spatial arrangement. This peculiarity is what makes neural networks potentially dangerous in real-world AI applications.
Elder emphasizes that deep AI models are prone to failure under specific conditions due to these shortcuts. Collaborating with industry and government partners, the research raises concerns about the safety and reliability of AI-driven systems currently in development.
In light of the study’s findings, Elder advocates for a more holistic approach to understanding visual processing in the brain. He suggests that expanding the focus beyond mere object recognition is crucial to achieving human-like configurable sensitivity in neural networks. Consequently, training these AI models to handle a diverse range of object tasks beyond category recognition might be the key to overcoming the limitations and failures encountered in complex applications.
The study serves as a crucial reminder to the AI community and industry stakeholders alike, urging them to prioritize explainability and comprehensive training protocols to ensure the responsible and effective implementation of AI technologies in mission-critical scenarios. By addressing these challenges head-on, we pave the way for a future where AI and human collaboration can thrive harmoniously, leading to groundbreaking advancements in various fields.
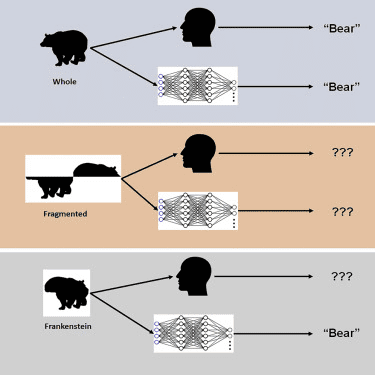
Source: ScienceDirect: article by Nicholas Baker and James H. Elder
Conclusion:
The study highlights the importance of addressing the challenges posed by neural network shortcuts in AI applications. As the market embraces AI technologies for critical tasks such as autonomous driving and medical diagnoses, it becomes imperative for businesses to prioritize explainability and comprehensive training protocols. By investing in responsible AI development and bridging the gap between AI and human cognition, companies can ensure the successful and safe implementation of AI solutions, opening doors to unprecedented opportunities and growth in various industries.