TL;DR:
- Researchers address the challenge of data deletion in image-to-image (I2I) generative models.
- They develop a machine unlearning framework for I2I generative models.
- The framework efficiently removes unwanted data while preserving data quality.
- An algorithm ensures minimal impact on retained data, complying with privacy regulations.
- Empirical studies on ImageNet1K and Places-365 datasets validate the algorithm’s efficacy.
- This research marks a significant advancement in machine unlearning for generative models.
Main AI News:
In an age where safeguarding digital privacy takes center stage, the demand for artificial intelligence (AI) systems to seamlessly erase specific data when required has evolved into a societal necessity. Researchers have embarked on an innovative journey to confront this challenge, particularly within the domain of image-to-image (I2I) generative models. Renowned for their ability to craft intricate images from given inputs, these models pose a unique obstacle to data deletion due to their deep learning architecture, which inherently retains training data.
The core of this research endeavor centers on the development of a machine unlearning framework, specifically tailored for I2I generative models. In contrast to previous endeavors primarily focused on classification tasks, this framework is geared towards efficiently eliminating unwanted data – aptly termed “forget samples” – while upholding the quality and integrity of the desired data, or “retaining samples.” Achieving this balance is no simple feat; generative models are inherently skilled at memorizing and reproducing input data, making selective forgetting a complex undertaking.
Researchers from The University of Texas at Austin and JPMorgan have proposed an algorithm grounded in a unique optimization problem to tackle this challenge. Through meticulous theoretical analysis, they have devised a solution that effectively expunges forgotten samples with minimal impact on the retained samples. Striking this equilibrium is crucial for complying with privacy regulations without compromising the overall performance of the model. The algorithm’s efficacy has been substantiated through rigorous empirical studies conducted on two substantial datasets, namely ImageNet1K and Places-365, illustrating its ability to align with data retention policies without necessitating direct access to the retained samples.
This pioneering research marks a significant stride in the realm of machine unlearning for generative models. It offers a tangible solution to a challenge that transcends technology, encompassing ethical and legal dimensions. The framework’s capacity to seamlessly erase specific data from memory without the need for a complete model retraining represents a leap forward in the development of privacy-compliant AI systems. By ensuring the integrity of retained data remains intact while eradicating information pertaining to forgotten samples, this research lays a robust foundation for the responsible utilization and management of AI technologies.
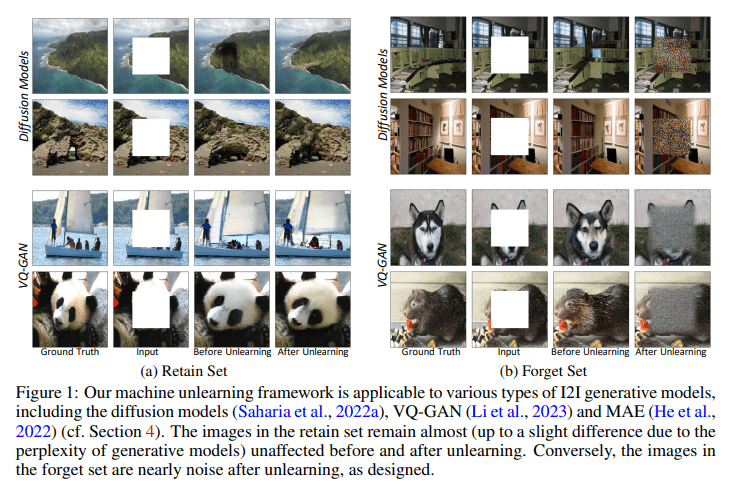
Source: Marktechpost Media Inc.
Conclusion:
This breakthrough in unlearning data within generative models has far-reaching implications for the market. It paves the way for the development of privacy-compliant AI systems, providing businesses with the tools to manage data responsibly and adhere to evolving privacy regulations. This advancement enhances the market’s ability to deploy AI technologies while safeguarding data integrity and privacy, strengthening consumer trust and expanding AI adoption across industries.