TL;DR:
- Researchers from Tohoku University and Shanghai Jiao Tong University introduced a machine learning method for predicting carbon nanostructure growth on metal surfaces.
- This innovation promises to simplify the design and synthesis of carbon nanostructures, unlocking their vast potential in electronics and energy processing.
- The method’s success was validated through simulations of graphene growth on various metallic surfaces, closely matching real-world experiments.
- The machine learning approach analyzes atomic-level factors, electron distributions, and material properties, providing efficient control over carbon crystalline growth.
- Future research will explore applications in advanced catalysts and energy storage materials.
Main AI News:
A groundbreaking development in the world of carbon nanotechnology promises to simplify the design and synthesis of carbon nanostructures. Researchers from Tohoku University in Japan and Shanghai Jiao Tong University in China have unveiled an innovative machine learning method that predicts the growth of carbon nanostructures on metal surfaces. This advancement is set to unlock the vast potential of carbon nanotechnology’s unique chemical versatility, with their findings published in the esteemed journal Nature Communications.
While the growth of carbon nanostructures on various surfaces, including atomically thin films, has been the subject of extensive research, understanding the dynamics and atomic-level factors that influence the quality of resulting materials has remained elusive. “Our work confronts a critical challenge in harnessing the potential of carbon nanostructures in electronics and energy processing devices,” explains Hao Li of Tohoku University.
The complexity of experimenting with a wide array of surfaces, combined with the sensitivity of the process to numerous variables, has made direct experimental investigation a formidable task. In response, the researchers turned to machine learning simulations as a more efficient means of exploring these intricate systems.
Through machine learning, a fusion of theoretical models and data from chemistry experiments enables the prediction of carbon crystalline growth dynamics and the control necessary to achieve precise outcomes. The simulation program autonomously explores strategies, discerning which ones prove effective and which do not, eliminating the need for continuous human intervention.
The initial test case involved simulating the growth of graphene, a carbon derivative, on a copper surface. Once the foundational framework was established, the researchers successfully extended their approach to encompass various metallic surfaces, including titanium, chromium, and copper contaminated with oxygen.
The distribution of electrons around atomic nuclei varies across different forms of graphene crystals, leading to subtle differences in atomic structure and electron arrangement that impact the overall chemical and electrochemical properties of the material. The machine learning approach enables rigorous testing of how these differences affect the diffusion of individual atoms, bonded atoms, and the formation of carbon chains, arches, and ring structures.
The results of these simulations were rigorously validated through experiments, confirming a remarkable alignment between theoretical predictions and real-world outcomes. Hao Li concludes, “In summary, our work presents a practical and efficient methodology for designing metallic or alloy substrates to achieve desired carbon nanostructures and explore a wide range of future possibilities.”
He also notes that forthcoming research will delve into topics such as the interfaces between solids and liquids in advanced catalysts, as well as the chemical properties of materials crucial for processing and storing energy. This groundbreaking development marks a significant stride toward realizing the full potential of carbon nanotechnology in various industrial applications.
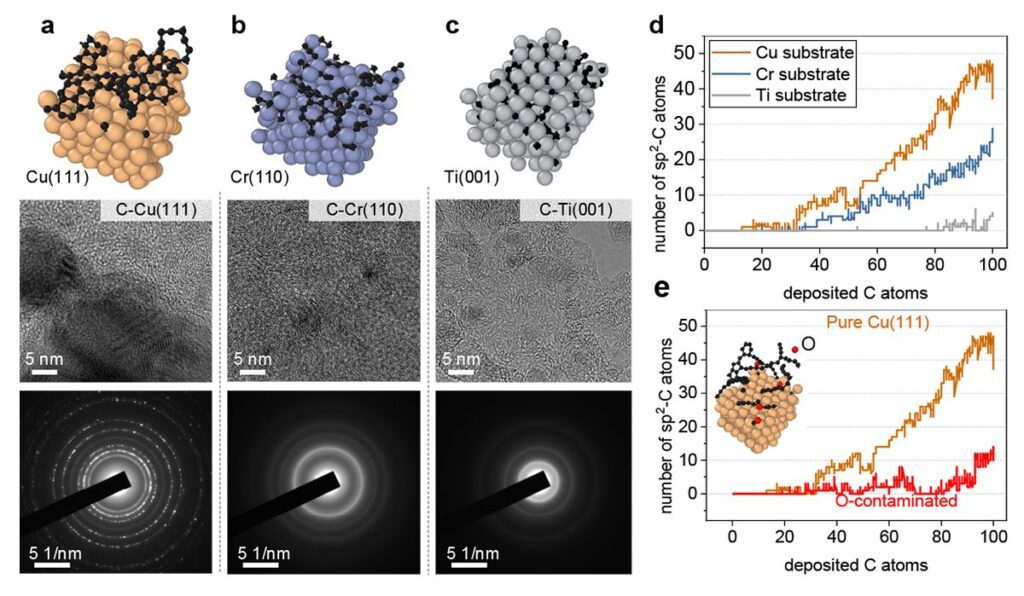
Source: Mirage.News real-time media portal
Conclusion:
The integration of machine learning into carbon nanotechnology not only simplifies the design and synthesis of carbon nanostructures but also offers practical and efficient methods for achieving desired outcomes. This development holds significant promise for the market by enabling the optimization of carbon nanotechnology for a wide range of applications, from electronics to energy processing devices, and even advanced catalyst interfaces and energy storage materials.