TL;DR:
- Machine learning system predicts polymer-solvent compatibility.
- Utilizes quantum chemistry data to overcome experimental limitations.
- Speedy calculations enable rapid screening of solvent candidates.
- Potential for selective plastic waste recycling and high-performance polymer blends.
- Open-source approach encourages data-driven refinement.
Main AI News:
In the dynamic landscape of materials science, the dissolution of polymers in organic solvents plays a pivotal role in numerous applications, from polymer synthesis to refining, painting, and coating. As the world grapples with the urgent need to reduce carbon emissions, particularly stemming from materials development, recycling plastic waste has emerged as a critical imperative.
The term “polymers” in this context encompasses plastics and plastic-like materials that necessitate specific solvents for effective dissolution and subsequent recycling. However, achieving this feat is far from straightforward. Leveraging the extensive quantum chemistry calculations within Mitsubishi Chemical Group’s (MCG) comprehensive databank, scientists have pioneered an innovative machine learning system designed to assess the compatibility of various polymers with potential solvents, quantified as χ (chi) parameters.
This groundbreaking system addresses the limitations arising from the scarcity of experimental data on polymer-solvent miscibility by harnessing the vast dataset generated through high-throughput quantum chemistry simulations.
The fruits of their labor have been documented in the esteemed journal Macromolecules. Through a methodological framework known as multitask learning, which adeptly integrates data from quantum chemistry calculations and real-world experiments, researchers have successfully fashioned a predictive model of extraordinary precision. This model empowers scientists to foresee the miscibility of any polymer-solvent blend with remarkable accuracy.
The implications of this innovation are far-reaching. It enables the selection and custom design of solvent molecules for the separation of specific materials within plastic waste, which often consists of diverse plastic types. These specially tailored solvents, referred to as “miscibilizers,” also facilitate the creation of high-performance polymer blends.
Ryo Yoshida, the study’s author and researcher, underscores the significance of this development, especially in the context of our evolving society: “In recent years, expectations for technological breakthroughs in recycling waste plastic resources have surged as we progress towards decarbonized societies. The development of miscibilizers for various polymer types will be indispensable in elevating waste plastic recycling rates.“
One standout feature of the predictive model is its remarkable speed. It computes χ parameters approximately 40 times faster than traditional quantum chemistry calculations. This accelerated pace enables the swift screening of millions of candidate solvent molecules, ushering in an era of ultrahigh-speed experimentation.
Indeed, the model has already proven its mettle in elucidating the key factors required to achieve homogenous mixtures of polymers and solvents, a prerequisite for effective recycling. This advancement significantly reduces the guesswork and trial-and-error traditionally associated with creating miscible substances suitable for recycling methods.
However, as with any pioneering technology, there remains room for refinement and optimization before widespread adoption. Yoshida acknowledges this reality: “To further enhance and expand the capabilities of machine learning techniques and foster open innovation and open science in materials informatics, we have made a portion of the source code and data publicly accessible.“
One limitation of the model lies in its inability to discern how a polymer’s miscibility relates to factors such as molecular weight or compositional features. Nevertheless, the decision to open-source data and source code represents a strategic move to expand the model’s dataset, enabling it to glean invaluable insights and refine its representation of polymer miscibility. Open innovation and crowd-sourced data collection hold the potential to rapidly enhance our understanding.
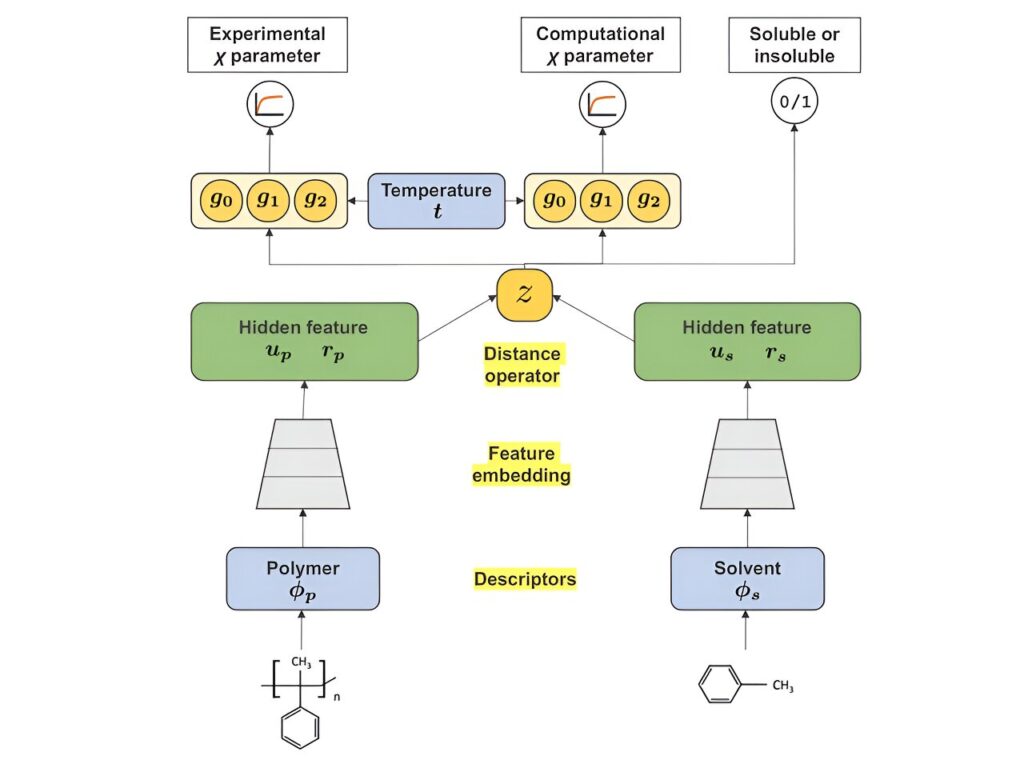
Source: Phys.org
Conclusion:
The development of a machine learning system for predicting polymer-solvent compatibility is poised to reshape the materials industry. By accelerating the selection of solvents and promoting sustainable practices through enhanced plastic recycling and high-performance polymer blends, this innovation aligns with the market’s growing demand for eco-friendly solutions. Its open-source nature also fosters collaboration and data-driven improvements, ensuring its continued impact on the market.