TL;DR:
- International astronomers employ machine learning to sift through 11 million images from the southern sky.
- They identify 581 strong lens candidates, shedding light on cosmic mysteries.
- The study pioneers the use of machine learning in astronomy, setting a precedent for automated data analysis.
- Challenges include the subjective nature of defining strong lenses and the reliance on simulated data for training models.
Main AI News:
In the heart of Chile, atop a lofty mountaintop 7,200 feet above sea level, the Cerro Tololo Inter-American Observatory stands as a symbol of human curiosity and exploration. Since 1976, beneath its colossal dome, scientists have been meticulously scrutinizing the cosmos one image at a time. However, as technology continues to flood us with an unprecedented influx of data, a glaring challenge persists – the sheer speed at which human eyes can process it.
This is where a global consortium of astronomers has embarked on an ingenious journey, harnessing the power of machine learning to navigate the celestial sea of data. Their mission: to uncover the hidden treasures of the universe, buried within millions of images.
Through a groundbreaking machine-learning model, researchers have accomplished the Herculean task of narrowing down a staggering 11 million images, all originating from the southern sky, to a mere 581 – each showcasing a cosmic phenomenon known as strong lensing.
The concept of strong lensing is as elegant as it is fascinating. When a colossal celestial body positions itself between a distant galaxy and our vantage point, its immense gravity acts as a cosmic magnifying glass, bending and distorting the light of the background galaxy. This transformative gravitational ballet not only magnifies the distant galaxy but also unveils its hidden intricacies.
In the annals of astronomical discovery, strongly lensed galaxies are rare gems. Only about a thousand of these enigmatic celestial arrangements have been confirmed among the countless galaxies cataloged. Graduate student Erik Zaborowski, from Ohio State University, who spearheaded this groundbreaking study published in the Astrophysical Journal on September 1st, remarked, “Strong lenses are really rare.” He envisions a future where observatories like the DECam-equipped Blanco Telescope unveil an uncharted sky rich with lenses, offering a unique opportunity to unravel the universe’s deepest mysteries.
These strongly lensed galaxies serve as invaluable tools for astronomers, enabling them to probe some of the most profound questions in the field, from the nature of dark matter to the universe’s expansion rate. However, most of the world’s significant astronomical endeavors have been centered in the Northern Hemisphere, leaving the southern sky relatively uncharted until recently.
The arrival of the Blanco Telescope’s Dark Energy Camera (DECam) in 2012 revolutionized the game. This specialized camera can capture vast swathes of the sky in a single frame, effectively covering an area equivalent to 20 full Moons as viewed from Earth.
As the sheer expanse of the southern sky opens up to exploration, it becomes evident that the volume of data to be analyzed is beyond human capacity. Greg Mosby of NASA Goddard, who utilizes machine-learning algorithms in his research, astutely observes, “The amount of data that we’re going to have to sift through is going to be impossible for a human to go by eye.” Thus, the dawn of automated data analysis, powered by machine learning, is upon us – a testament to the changing times in astronomy.
Enter machine learning, a burgeoning subfield of artificial intelligence that empowers computer algorithms to autonomously discern patterns from training data, eliminating the need for direct human intervention. This technological paradigm shift has already birthed familiar applications like language translation, targeted advertising, and image recognition, with the latter finding newfound significance in the realm of astronomy.
Zaborowski and his team were trailblazers in applying machine learning to public data from the DECam Local Volume Exploration Survey (DELVE), which commenced in 2019, amassing a staggering 520 million cosmic sources.
From this colossal dataset, the researchers meticulously selected 11 million extended sources, objects that manifest differently from point sources in images, as the starting point for their quest to identify strongly lensed galaxies. These sources were then fed into a five-layered convolutional neural network, a machine learning model specially designed for image classification.
But before this network could discern real lensed galaxies, it had to undergo training. Over 80,000 authentic galaxy images from DELVE were fed into the system, with an artificial lensing effect applied to half of them. An additional 3,200 false positives, images resembling strongly lensed galaxies but not exhibiting the phenomenon, were included to enhance the model’s precision.
However, human expertise still played a pivotal role in the process. Out of the 11 million DELVE sources, the model generated a list of 50,000 candidates most likely to be lenses. Subsequently, scientists reverted to traditional methods, meticulously examining each candidate by eye. Upon completing this arduous task, they emerged with 581 extended sources strongly indicative of being lensed galaxies, out of which a remarkable 562 had never been previously reported. Additionally, the team discovered eight potentially lensed quasars, highly luminous galactic cores, hinting at a substantial expansion of the known population of strongly lensed galaxies.
Yet, as we rejoice in this monumental discovery of new candidate strong lenses, it prompts astronomers to contemplate the challenges of navigating this new era characterized by vast databases and machine-learning models. One prominent concern is the inherent reliance of machine learning on training data. Given the scarcity of authentic strong lens images, scientists are compelled to train models on simulated data. As Ben Metcalf of the University of Bologna notes, “I do have some reservations. If there’s something in the real data that you haven’t put in the simulated data, you just don’t know how [the model] reacts to that at all.”
Furthermore, the definition of what visually constitutes a strong lens is subjective, demanding a consensus among scientists to guide machine learning models in their initial selection process.
Although machine learning may not be infallible, its paramount strength lies in its remarkable speed. Tedious image classifications that would typically consume months of human effort can now be executed within mere hours by these models. As we find ourselves drowning in an ever-expanding sea of data, this study underscores machine learning’s capacity to propel us forward, equipping us with the insights strong lenses offer into the mysteries of the universe.
Erik Zaborowski encapsulates the essence of this transformative technology when he asserts, “There are a lot of kinds of analyses that you can really only do with a lot of these [lenses]. With that data, you can start answering the science questions.” Indeed, this is the dawn of a new era in astronomy, one where the cosmos itself invites us to unravel its deepest enigmas through the lens of machine learning.
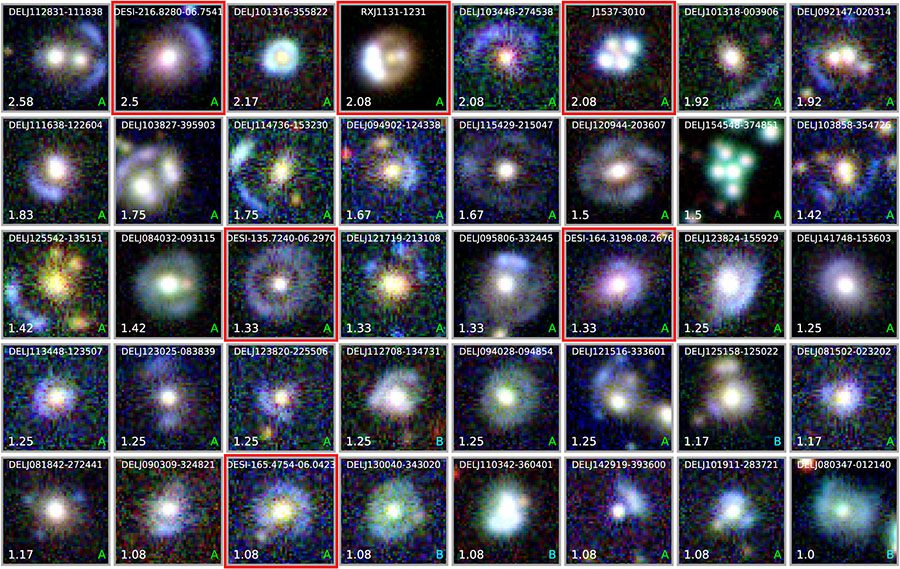
Source: AAS Sky Publishing LLC.
Conclusion:
The utilization of machine learning to discover 562 new strong lens candidates marks a significant advancement in the field of astronomy. This breakthrough showcases the potential of automation in analyzing vast astronomical datasets, offering a glimpse into the market’s future, where AI-driven tools could revolutionize data processing and accelerate scientific discoveries.