TL;DR:
- MM-Grounding-DINO, developed by Shanghai AI Lab and SenseTime Research, is an open-source pipeline designed for unified object grounding and detection.
- It excels in handling Open-Vocabulary Detection (OVD), Phrase Grounding (PG), and Referring Expression Comprehension (REC).
- MM-Grounding-DINO leverages diverse vision datasets for pre-training and fine-tuning on various detection and grounding datasets.
- The model’s transparency and reproducibility are underscored by a comprehensive analysis of reported results and detailed settings.
- Extensive benchmark experiments reveal that MM-Grounding-DINO-Tiny surpasses the performance of the Grounding-DINO-Tiny baseline.
- The model achieves state-of-the-art performance in zero-shot settings on COCO with a mean average precision (mAP) of 52.5.
- It outperforms fine-tuned models in diverse domains, setting new benchmarks for mAP.
Main AI News:
In the realm of multi-modal understanding systems, the importance of object detection cannot be overstated. This critical process involves feeding images into models to generate proposals that align seamlessly with textual descriptions. It serves as the backbone for cutting-edge models designed to handle Open-Vocabulary Detection (OVD), Phrase Grounding (PG), and Referring Expression Comprehension (REC). OVD models, for instance, are equipped to handle base categories in zero-shot scenarios while venturing into the uncharted territory of predicting both base and novel categories within a vast vocabulary. PG steps in to provide a descriptive phrase for candidate categories and then conjures up the corresponding bounding boxes. REC, on the other hand, takes precision to another level by accurately identifying a target mentioned in the text and outlining its exact position with a bounding box. The revolutionary Grounding-DINO addresses these complex tasks, gaining widespread acclaim and adoption across diverse applications.
Enter MM-Grounding-DINO, a groundbreaking solution crafted by the collaborative efforts of researchers from Shanghai AI Lab and SenseTime Research. This user-friendly and open-source pipeline, meticulously hewn using the MMDetection toolbox, stands as a testament to innovation. Its journey begins with pre-training on a plethora of diverse vision datasets, followed by fine-tuning on an array of detection and grounding datasets. What sets MM-Grounding-DINO apart is its commitment to transparency and reproducibility. This is exemplified by a comprehensive analysis of reported results and an exhaustive breakdown of settings, all aimed at empowering fellow researchers to replicate and build upon its achievements.
Through an extensive series of experiments on benchmark datasets, MM-Grounding-DINO-Tiny emerges as a force to be reckoned with, surpassing the performance of the Grounding-DINO-Tiny baseline. Its prowess lies in its ability to seamlessly bridge the gap between textual descriptions and image content. This is made possible through a carefully orchestrated ensemble of components, each contributing to the model’s remarkable success.
At the core of MM-Grounding-DINO lies a text backbone, entrusted with the responsibility of extracting rich features from textual descriptions. Simultaneously, an image backbone diligently extracts features from images, regardless of their shapes and sizes. The magic truly happens when these extracted features converge in the feature enhancer module, fostering a harmonious fusion of image and text features. This module, a true testament to the power of cross-modality collaboration, employs a Bi-Attention Block, which orchestrates a delicate dance between text-to-image and image-to-text cross-attention layers. As if that weren’t enough, the fused features undergo further refinement through the judicious use of vanilla self-attention and deformable self-attention layers, culminating in a Feedforward Network (FFN) layer.
When presented with the intricate task of processing an image-text pair, MM-Grounding-DINO springs into action. Its image backbone diligently extracts features from the image at multiple scales. In parallel, the text backbone extracts features from the accompanying text, setting the stage for a symphony of fusion. This fusion is brought to life through the Bi-Attention Block, orchestrating a delicate interplay of text and image features. The ensuing transformation is nothing short of remarkable, thanks to the judicious application of self-attention layers and a Feedforward Network (FFN) layer.
In a world where unified object grounding and detection are paramount, MM-Grounding-DINO stands as a beacon of hope. It offers a comprehensive pipeline that addresses the intricacies of OVD, PG, and REC tasks. The model’s performance is put to the test through a visualization-based analysis, revealing the imperfections in the ground-truth annotations of the evaluation dataset. In the realm of zero-shot settings on COCO, MM-Grounding-DINO shines bright with a mean average precision (mAP) of 52.5. But its brilliance doesn’t end there. The model outperforms fine-tuned counterparts in diverse domains, from marine objects to brain tumor detection, urban street scenes to people in paintings, establishing new benchmarks for mAP. In the world of unified object grounding and detection, MM-Grounding-DINO reigns supreme, poised to shape the future of multi-modal understanding systems.
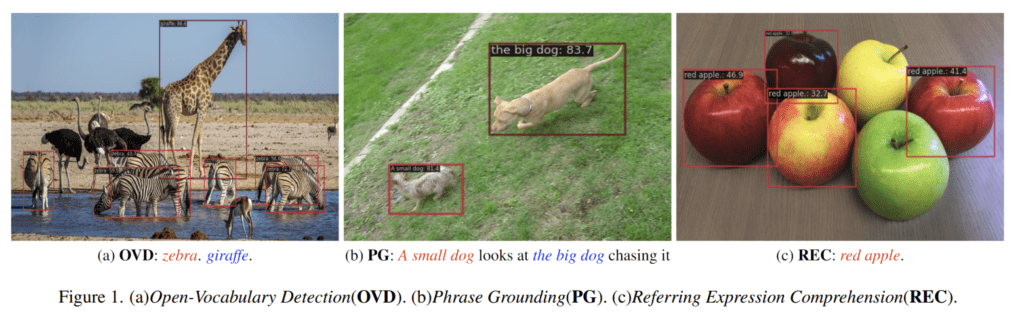
Source: Marktechpost Media Inc.
Conclusion:
MM-Grounding-DINO’s innovation and remarkable performance in unified object grounding and detection represent a significant advancement in the market. Its transparency and reproducibility make it a valuable resource for researchers, while its state-of-the-art capabilities open doors to new possibilities in multi-modal understanding systems. This breakthrough technology is poised to shape the future of object detection and grounding applications across various domains, creating exciting opportunities for businesses and industries to leverage its capabilities for improved accuracy and efficiency.