TL;DR:
- Nvidia introduces NeMo Retriever, a generative AI microservice.
- NeMo Retriever connects custom large language models to enterprise data.
- It utilizes retrieval-augmented generation (RAG) for accurate AI responses.
- Part of Nvidia’s AI Enterprise software platform, available on AWS Marketplace.
- Jensen Huang, Nvidia CEO, emphasizes the potential of RAG-capable AI applications.
- NeMo Retriever enhances generative AI accuracy, stability, security, and efficiency.
- Supports connections to various data sources, enabling conversational interaction.
- Collaboration with Cadence, Dropbox, SAP, and ServiceNow to integrate RAG capabilities.
- NeMo Retriever’s RAG process reduces hallucinations with a verification step.
- Vectara’s Hallucination Evaluation Model aids in verification.
Main AI News:
In a groundbreaking move, Nvidia has introduced the NeMo Retriever, a revolutionary generative AI microservice designed to combat the issue of inaccurate responses generated by AI systems. This innovative solution is poised to transform the enterprise landscape by connecting custom large language models (LLMs) with corporate data, ensuring precise and reliable responses to user queries. At its core, the NeMo Retriever harnesses semantic retrieval, incorporating cutting-edge retrieval-augmented generation (RAG) capabilities powered by Nvidia’s advanced algorithms. This game-changing microservice is a pivotal component of Nvidia’s AI Enterprise software platform, now conveniently accessible through the AWS Marketplace.
Jensen Huang, co-founder and CEO of Nvidia, affirms, “Generative AI applications with RAG capabilities are the next frontier in enterprise technology. With Nvidia NeMo Retriever, developers gain the power to create tailored generative AI chatbots, co-pilots, and summarization tools that tap into their organization’s data reservoirs, revolutionizing productivity through precise and valuable generative AI intelligence.”
NeMo Retriever brings a host of benefits to the table, including robust support for production-ready generative AI models, API stability, security enhancements, and top-notch enterprise assistance—a marked departure from open source RAG toolkits. Embedded models within the system capture intricate word relationships, enabling LLMs (large language models, the foundation of generative AI) to efficiently process and analyze textual data.
One of the standout features of NeMo Retriever is its capability to seamlessly connect LLMs with diverse data sources, encompassing text, PDFs, images, videos, and knowledge bases. This empowers users to engage with data and receive accurate, real-time responses through simple, conversational prompts. The result is a significant boost in accuracy, reduced training requirements, expedited time-to-market, and heightened energy efficiency in the development of generative AI applications.
Leading companies in various industries, including Cadence (industrial electronics design), Dropbox, SAP, and ServiceNow, have joined forces with Nvidia to incorporate production-ready RAG capabilities into their bespoke generative AI applications and services.
A Game-Changing Approach to Mitigating LLM Hallucinations
Retrieval-augmented generation represents a revolutionary approach to mitigating the issue of hallucinatory responses from generative AI chatbots powered by LLMs. Traditional models are often trained on a broad spectrum of information, lacking the specificity of domain-specific data. The NeMo Retriever tackles this problem by translating user requests into a set of coded parameters known as vector embeddings. These embeddings are then employed to query a domain-specific database containing information relevant to the user’s organization. The RAG query scours the database for similarities between the input request vector embeddings and the database’s vector-embedded content, selecting the content that best aligns with the user’s context.
It’s worth noting that while the RAG process significantly reduces hallucinations, a verification step is crucial to ensure that the output remains contextually relevant, grounded, and accurate. To aid in this verification process, Vectara has developed an open-source Hallucination Evaluation Model, providing an added layer of confidence in the reliability of generative AI responses.
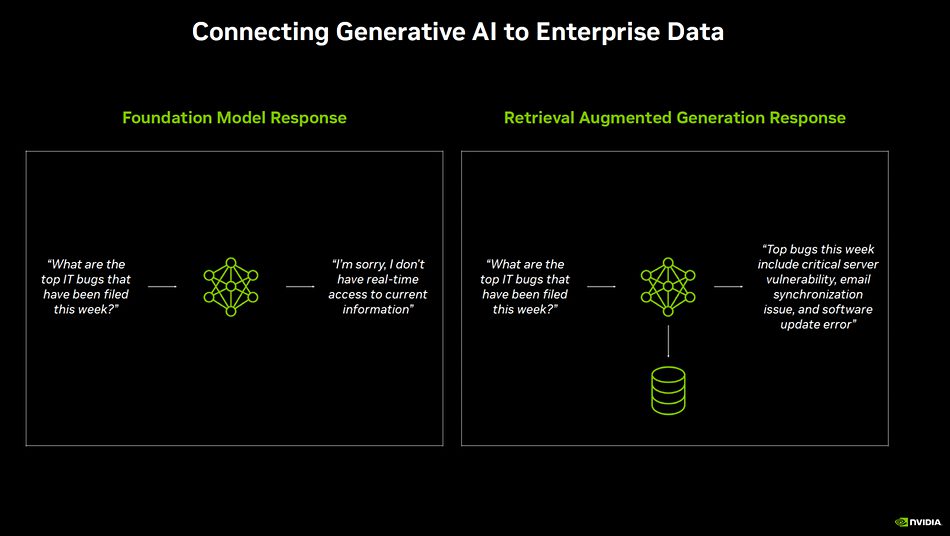
Source: Situation Publishing
Conclusion:
Nvidia’s NeMo Retriever marks a significant milestone in AI error reduction for enterprises. With its RAG capabilities, it enhances accuracy and efficiency, offering businesses a powerful tool to leverage generative AI effectively. This development reflects the growing importance of precise AI applications in the market, driving increased productivity and reliability in enterprise operations.