TL;DR:
- COLE is an innovative hierarchical generation framework for graphic design.
- It leverages Large Language Models (LLMs) and curated datasets for intent comprehension.
- COLE excels in generating diverse visual components and typographic features.
- Quality assurance and user feedback are integral to COLE’s process.
- The system empowers designers of all levels to create high-quality graphic designs efficiently.
Main AI News:
In the realm of visual communication, graphic design plays a pivotal role in conveying distinct messages to specific social audiences. It’s a discipline that demands creativity, innovation, and rapid ideation. The fusion of text and visuals, whether through digital or manual means, is the core approach to crafting visually compelling narratives. Its primary objective? To structure data, breathe life into concepts, and infuse emotions into objects that capture the human experience. The strategic use of typefaces, text layout, embellishments, and imagery often breathes life into ideas, sentiments, and perspectives that defy mere words. Achieving top-tier designs requires a profound wellspring of creativity, innovative thinking, and lateral problem-solving.
While the world witnessed substantial advancements in natural picture production, thanks to groundbreaking technologies like DALL•E3, SDXL, and Imagen, these strides are inherently linked to leveraging the power of Large Language Models (LLMs) as text encoders, expanding training datasets, augmenting model complexity, refining sampling strategies, and enhancing data quality. Amidst this transformation, it becomes evident that the field of graphic design, with its pivotal role in branding, marketing, and advertising, warrants a professional makeover.
In a significant departure from its predecessors, COLE, developed by researchers from Microsoft Research Asia and Peking University, emerges as a revolutionary hierarchical generation framework poised to streamline the intricate process of graphic design creation. At its core, COLE employs a multifaceted approach, employing specialized generation models for various sub-tasks.
The journey commences with a profound focus on imaginative design and intent comprehension. Utilizing state-of-the-art LLMs, particularly the Llama2-13B, and fine-tuning them with a meticulously curated dataset of nearly 100,000 intention-JSON pairings, COLE delves deep into understanding creative intents. This comprehensive dataset encompasses textual descriptions, item captions, backdrop captions, and even optional parameters for object positioning.
The second phase revolves around refining and enhancing visual elements, encompassing two crucial subtasks: the generation of visual components and typographic features. The creation of diverse visual attributes entails the fine-tuning of specialized cascaded diffusion models, exemplified by DeepFloyd/IF. These models are meticulously designed to ensure seamless transitions between components, ranging from layered object images to intricately adorned backdrops. Simultaneously, COLE predicts typography JSON files using a Large Multimodal Model (LMM) meticulously crafted with LLaVA-1.5-13B. This model leverages the anticipated JSON file from the Design LLM, the projected backdrop image from a diffusion model, and the expected object image from a cascaded diffusion model. A visual renderer skillfully assembles these components, adhering to the layout specified in the anticipated JSON file.
The final stage of COLE’s journey is dedicated to quality assurance and feedback, which significantly elevates the overall design quality. This phase involves meticulous adjustments of a reflection LMM and harnessing the power of GPT-4V(ision) for a comprehensive and multifaceted quality assessment. Here, the JSON file can be easily fine-tuned, allowing for adjustments to text box sizes and positions as needed.
In a comprehensive evaluation, the research team assessed COLE’s capabilities against approximately 200 professional graphic design intention prompts, spanning various categories and including around 20 creative prompts. They conducted exhaustive ablation experiments for each generation model across various sub-tasks, offering a detailed analysis of the graphic designs produced by their system. Additionally, they engaged in a discussion surrounding the limitations and potential future directions in the realm of graphic design image generation.
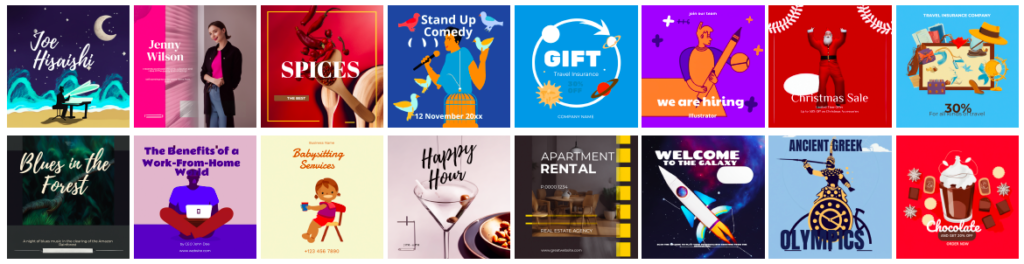
Source: Marktechpost Media Inc.
Conclusion:
COLE’s introduction into the market signifies a transformative shift in graphic design, making it more accessible and efficient. This revolutionary framework empowers designers to produce high-quality graphics effortlessly, unlocking new possibilities for branding, marketing, and advertising. It paves the way for a more democratized and innovative graphic design landscape, poised to reshape the industry.