TL;DR:
- PIXART-δ combines Latent Consistency Models (LCM) and ControlNet for superior text-to-image synthesis.
- Achieves remarkable inference speed of 0.5 seconds for 1024×1024 images on an A100 GPU.
- Novel ControlNet-Transformer architecture enhances controllability and performance.
- Latent Consistency Distillation (LCD) algorithm with Classifier-Free Guidance (CFG) improves training efficiency.
- PIXART-δ supports training on consumer-grade GPUs, broadening accessibility.
- Outperforms competitors in inference speed, maintaining efficiency with just four steps.
- Rapid convergence and superior edge quality observed in ControlNet-Transformer.
- PIXART-δ promises to revolutionize the text-to-image synthesis landscape.
Main AI News:
In today’s dynamic landscape of text-to-image models, the demand for high-quality visuals is at an all-time high. However, the road to achieving this level of excellence has often been paved with resource-intensive training and sluggish inference speeds, limiting their real-time utility. In response to these challenges, we proudly present PIXART-δ, a groundbreaking advancement that seamlessly fuses Latent Consistency Models (LCM) and a customized ControlNet module into the existing PIXART-α framework.
PIXART-α, renowned for its efficient training and superior image generation capabilities, serves as the solid foundation upon which PIXART-δ is built. What sets PIXART-δ apart is its integration of LCM, which significantly accelerates the inference process, enabling the generation of high-quality images in just 2∼4 steps on pre-trained Latent Diffusion Models (LDMs). This remarkable enhancement allows PIXART-δ to achieve an astonishing inference speed of 0.5 seconds per 1024 × 1024 image on an A100 GPU, marking a remarkable 7× improvement over its predecessor, PIXART-α.
The incorporation of ControlNet, originally designed for UNet architectures, posed a unique challenge when applied to Transformer-based models like PIXART-δ. To conquer this challenge, our dedicated team devised a pioneering ControlNet-Transformer architecture, ensuring seamless integration while preserving the efficacy of ControlNet in managing crucial control information. The proposed design strategically applies the ControlNet structure to the initial N base blocks of the Transformer, resulting in substantial improvements in controllability and overall performance.
The training process is further optimized through Latent Consistency Distillation (LCD), an evolved iteration of the original Consistency Distillation (CD) algorithm. Our algorithm, outlined in the accompanying Algorithm section, incorporates Classifier-Free Guidance (CFG), where the Teacher, Student, and EMA Model function as denoisers for the ODE solver. The innovative LCD algorithm demonstrates its effectiveness, as substantiated by rigorous evaluations using FID and CLIP scores as performance benchmarks.
One of the standout features of PIXART-δ is its exceptional training efficiency. It successfully undergoes the distillation process within the confines of a 32GB GPU memory constraint, supporting image resolutions up to 1024 × 1024. This remarkable efficiency ensures that PIXART-δ can be trained on consumer-grade GPUs, significantly broadening its accessibility to a wider audience.
When it comes to inference speed, PIXART-δ outshines comparable methods such as SDXL LCM-LoRA, PIXART-α, and the SDXL standard across various hardware platforms. With a mere four steps, PIXART-δ maintains a consistent lead in generation speed, underscoring its efficiency compared to the 14 and 25 steps required by PIXART-α and SDXL standards, respectively.
The integration of ControlNet into PIXART-δ involves replacing the original zero-convolution with a specially tailored zero linear layer designed for Transformer architectures. The ControlNet-Transformer design selectively applies ControlNet to the initial N base blocks, achieving a harmonious integration that enhances controllability and overall performance.
An in-depth ablation study on ControlNet-Transformer unequivocally demonstrates its superiority, showcasing faster convergence and improved performance across various scenarios. While satisfactory results are attained with N = 1 for most scenarios, it’s worth noting that increased performance is observed in challenging edge conditions as N is incremented.
Our analysis of the impact of training steps on ControlNet-Transformer (N = 13) reveals rapid convergence, particularly noticeable in enhancing the quality of outline edges for human faces and bodies. The efficiency and effectiveness of ControlNet-Transformer are further underscored, reinforcing its potential for real-time applications.
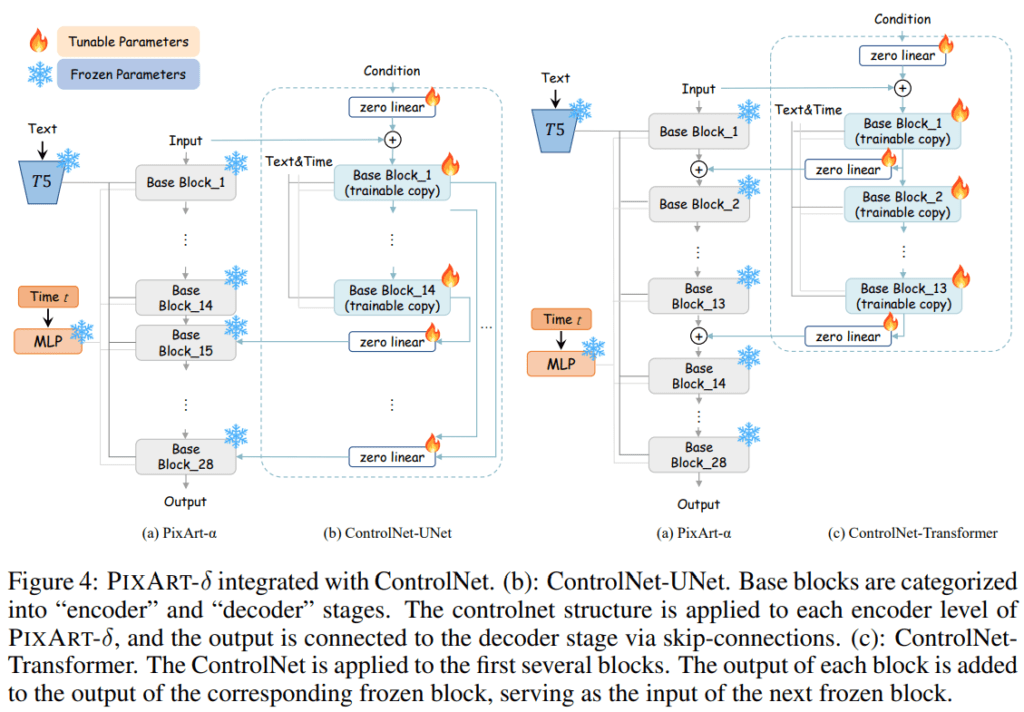
Source: Marktechpost Media Inc.
Conclusion:
PIXART-δ’s groundbreaking advancements in text-to-image synthesis have the potential to reshape the market landscape. Its unmatched efficiency and quality will empower businesses to generate high-quality visuals faster and with greater control, unlocking new possibilities for content creation, marketing, and customer engagement. This innovation positions PIXART-δ as a game-changer, offering businesses a competitive edge in the digital age.