TL;DR:
- Cornell and Tel Aviv researchers introduce “Doppelgangers” dataset for visual disambiguation.
- The dataset aids in distinguishing identical and visually similar 3D surfaces in images.
- Feature-matching methods and binary masks refine image pairs for analysis.
- A specialized network architecture enhances visual disambiguation accuracy.
- Impressive performance was demonstrated, surpassing baseline approaches.
- Potential for practical application in scene graph computations and 3D reconstruction.
- Promises improved reliability and precision in computer vision systems.
Main AI News:
In the ever-evolving realm of computer vision, distinguishing between similar structures in images can be akin to identifying subtle differences between identical twins. This challenge becomes particularly critical in geometric vision tasks like 3D reconstruction, where the accuracy of discerning whether two images depict the same 3D surface or two strikingly similar yet distinct surfaces can make or break the resulting 3D models. This intricate task is aptly termed “visual disambiguation.”
Addressing this challenge head-on, a team of researchers from Cornell University has introduced a groundbreaking solution—meet “Doppelgangers.” This innovative dataset comprises pairs of images, carefully curated to include those that portray either identical 3D surfaces (the positives) or two separate 3D surfaces bearing a remarkable resemblance (the negatives). Constructing the Doppelgangers dataset proved to be no small feat, as even human observers can struggle with this differentiation. Leveraging the power of existing image annotations from the Wikimedia Commons image database, the team harnessed automation to generate a substantial collection of labeled image pairs.
The journey to visual disambiguation begins with the presentation of a pair of images. Employing feature-matching methods, key points and matches are meticulously extracted. Notably, within this context, the images under scrutiny represent a negative pair, showcasing opposing facets of the iconic Arc de Triomphe. Interestingly, the feature matches predominantly converge in the upper portion of the structure, characterized by recurring elements, in stark contrast to the lower section adorned with sculptures.
To further refine the process, binary masks for key points and matches are meticulously created. Subsequently, both the image pair and their corresponding masks undergo alignment through the application of an affine transformation, a procedure determined by the identified matches.
Crucially, the classifier at play here takes the amalgamation of images and binary masks as input, producing an output probability that serves as an indicator of the likelihood that the given pair constitutes a positive match.
However, it was observed that training a deep network model directly on these raw image pairs yielded less-than-satisfactory results. In response, a dedicated network architecture was meticulously crafted, one that incorporates invaluable information in the form of local features and 2D correspondences, thus amplifying the performance of the visual disambiguation task.
In the rigorous evaluation conducted using the Doppelgangers test set, this pioneering method proved its mettle by delivering impressive results in tackling intricate disambiguation tasks. It outperformed not only baseline approaches but also alternative network designs by a substantial margin. Beyond this, the study delves into the practicality of the learned classifier, demonstrating its potential as a straightforward pre-processing filter within structure-from-motion pipelines, such as COLMAP.
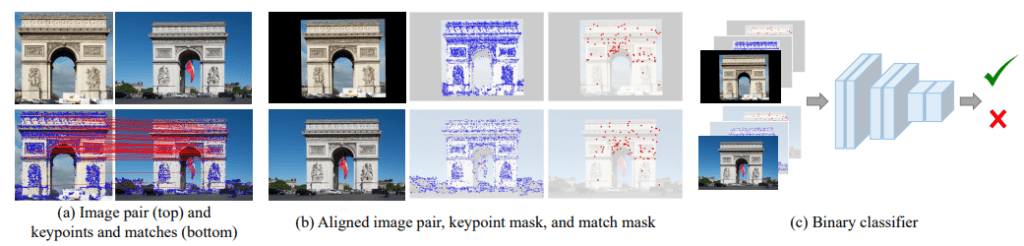
Source: Marktechpost Media Inc.
Conclusion:
The introduction of Doppelgangers by Cornell and Tel Aviv University represents a significant advancement in the field of computer vision. This innovative dataset and improved network architecture have the potential to greatly enhance the reliability and precision of computer vision systems, particularly in applications related to 3D reconstruction. This could open up new opportunities and markets for enhanced visual recognition and reconstruction technologies, benefitting industries ranging from augmented reality to autonomous vehicles.