TL;DR:
- Self-Rewarding Language Models introduced by Meta and NYU.
- Overcoming limitations of traditional reward models derived from human preferences.
- Direct Preference Optimization (DPO) and Traditional Reinforcement Learning from Human Feedback (RLHF) challenges.
- Self-improving reward model continuously updated during LLM alignment.
- Integration of instruction-following and reward modeling for self-assessment.
- The outperformance of existing models on AlpacaEval 2.0 leaderboard.
- A promising avenue for self-improvement in language models.
Main AI News:
In a groundbreaking paper jointly authored by Meta and New York University, a revolutionary concept is introduced: Self-Rewarding Language Models. These models represent a quantum leap in the realm of artificial intelligence and language processing. They possess the extraordinary ability to align themselves through the process of self-judgment and self-training, setting a new standard for the development of superhuman agents.
The traditional approach to training large language models (LLMs) has been reliant on deriving reward models from human preferences. However, this method is fraught with limitations, as it is bound by the constraints of human performance. Fixed reward models hinder the potential for enhancing learning during LLM training, thus impeding progress toward creating agents that surpass human capabilities.
Recent studies have demonstrated the importance of leveraging human preference data to improve the effectiveness of LLMs. Traditional Reinforcement Learning from Human Feedback (RLHF) has been the go-to method, involving the creation of a fixed reward model from human preferences. An emerging alternative, Direct Preference Optimization (DPO), seeks to skip the reward model training step and directly employ human preferences for LLM training. Yet, both approaches face challenges related to the quantity and quality of available human preference data.
Enter Self-Rewarding Language Models, a game-changing approach that promises to overcome these hurdles. Unlike the static nature of frozen reward models, this innovative method involves training a self-improving reward model that evolves continuously during LLM alignment. By seamlessly integrating instruction-following and reward modeling into a single system, the model generates and assesses its own examples, refining its instruction-following and reward modeling abilities.
The process begins with a pretrained language model and a limited set of human-annotated data. These models excel in two pivotal skills: instruction following and self-instruction creation. Through the LLM-as-a-Judge mechanism, the model self-evaluates its generated responses, eliminating the need for external reward models. The iterative self-alignment process encompasses the development of new prompts, evaluation of responses, and model updates through AI Feedback Training. This approach deviates from the conventional fixed reward models, ushering in a new era of adaptability and self-improvement.
The results speak for themselves. Self-Rewarding Language Models have exhibited substantial improvements in instruction following and reward modeling through iterative training iterations. They have even surpassed existing models on the AlpacaEval 2.0 leaderboard, including Claude 2, Gemini Pro, and GPT4, by leveraging proprietary alignment data. This groundbreaking approach offers a promising path for language models to continually enhance their performance, surpassing the limitations of relying solely on positive examples.
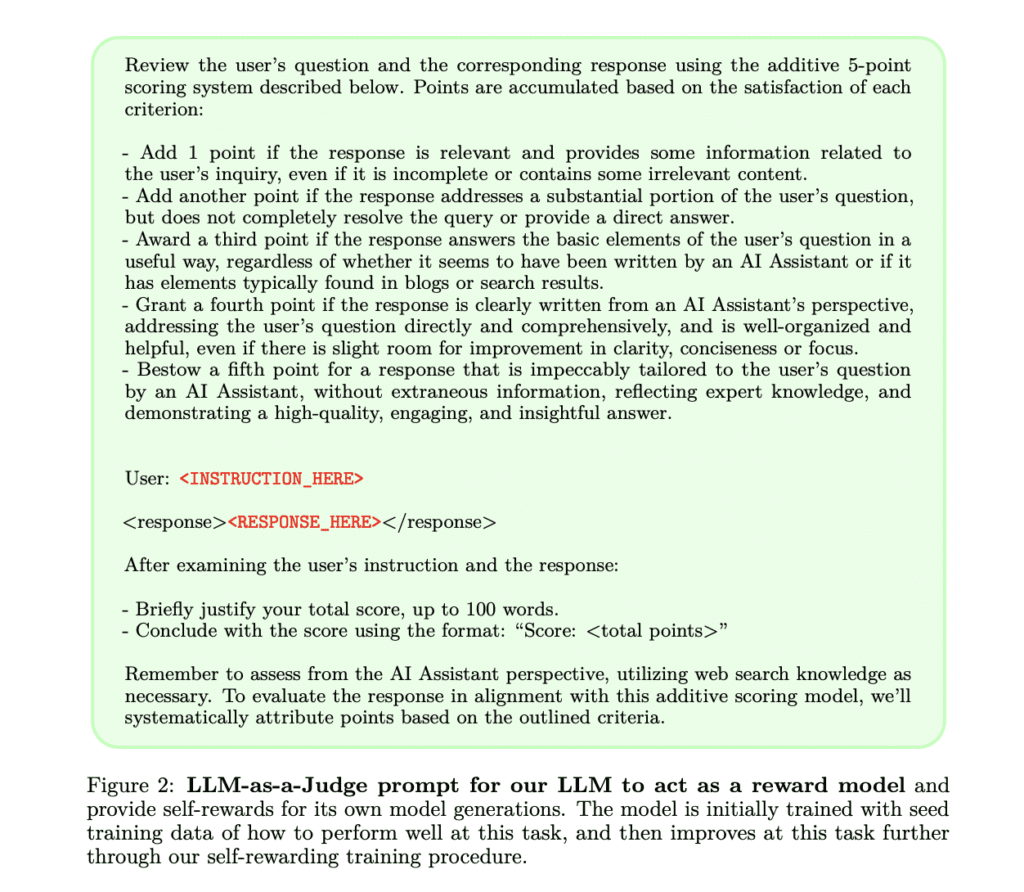
Source: Marktechpost Media Inc.
Conclusion:
The introduction of Self-Rewarding Language Models represents a significant advancement in the AI market. These models have the potential to outperform existing methods by continuously enhancing their performance, setting a new standard for language processing and artificial intelligence. Businesses and researchers in the AI sector should closely monitor this development as it could shape the future landscape of AI technologies and applications.