TL;DR:
- RPG (Recaption, Plan, and Generate) is a pioneering Text-to-Image Generation/Editing Framework.
- Developed by researchers from Peking University, Pika, and Stanford University, it excels in handling complex text prompts.
- RPG harnesses Multimodal Large Language Models (MLLMs) for improved compositionality.
- Its three core strategies are Multimodal Recaptioning, Chain-of-Thought Planning, and Complementary Regional Diffusion.
- RPG stands out with its closed-loop editing approach, enhancing generative power.
- The framework utilizes GPT-4 as the reception and CoT planner, with SDXL as the diffusion backbone.
- Extensive experiments confirm RPG’s superiority in multi-category object composition and text-image alignment.
- RPG outperforms other models in precision, flexibility, and generative ability.
- It represents a promising advancement in the field of text-to-image synthesis.
Main AI News:
In the realm of cutting-edge AI research, a groundbreaking paper emerges, introducing RPG (Recaption, Plan, and Generate), a revolutionary Text-to-Image Generation/Editing Framework. Developed by a collaboration of brilliant minds hailing from Peking University, Pika, and Stanford University, RPG represents the pinnacle of innovation in the field. This framework is specifically designed to tackle the intricate landscape of text-to-image conversion, particularly excelling in the realm of complex text prompts that involve multiple objects with diverse attributes and intricate relationships.
While conventional models have exhibited remarkable prowess in handling simple prompts, they often falter when confronted with the challenge of accurately translating intricate prompts that necessitate the synthesis of multiple entities into a single image. Previous approaches have attempted to address these challenges by introducing additional layouts or boxes, employing prompt-aware attention guidance, or utilizing image understanding feedback to refine the generation process. However, these methods are not without limitations, particularly when dealing with overlapping objects and the escalated training costs associated with complex prompts.
Enter RPG, a pioneering training-free text-to-image generation framework that capitalizes on the multifaceted capabilities of Multimodal Large Language Models (MLLMs) to enhance the compositional prowess of text-to-image diffusion models. RPG operates on three fundamental strategies: Multimodal Recaptioning, Chain-of-Thought Planning, and Complementary Regional Diffusion, each contributing to the heightened flexibility and precision in long text-to-image generation. RPG distinguishes itself by employing a closed-loop editing approach, a distinctive feature that bolsters its generative prowess.
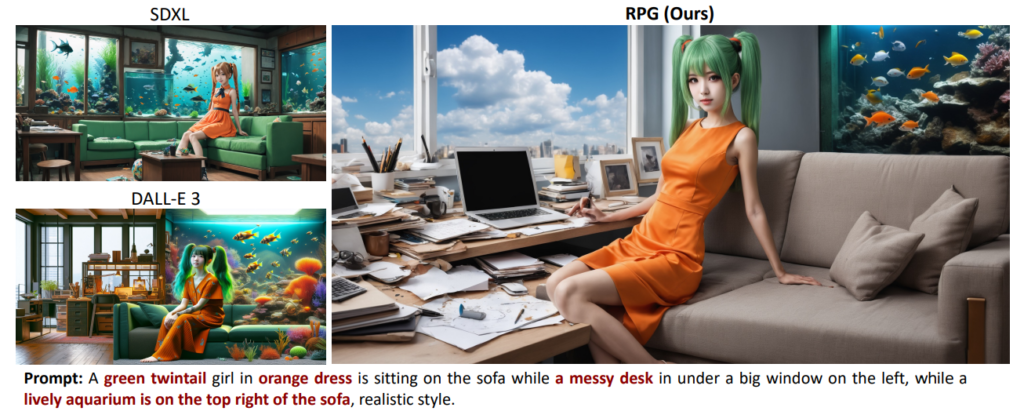
Source: Marktechpost Media Inc.
Let’s delve into the essence of each strategy:
- Multimodal Recaptioning: MLLMs play a pivotal role in this strategy by transforming text prompts into highly descriptive narratives, dissecting them into discrete subprompts. This process enriches the depth and detail of the generated images.
- Chain-of-Thought Planning: This strategy involves segmenting the image space into complementary subregions, assigning distinct subprompts to each subregion, and harnessing the power of MLLMs to facilitate efficient region division. The result is a coherent and well-structured image synthesis process.
- Complementary Regional Diffusion: This component enables region-wise compositional generation by independently generating image content guided by subprompts within designated regions and subsequently merging them spatially. It ensures the seamless integration of various elements within the generated image.
The RPG framework leverages the capabilities of GPT-4 as the reception and CoT planner, with SDXL serving as the foundational diffusion backbone. Rigorous experiments have unequivocally established RPG’s superiority over existing models, particularly in the realms of multi-category object composition and text-image semantic alignment. This methodology exhibits remarkable adaptability, extending its excellence across various MLLM architectures and diffusion backbones.
In a series of exhaustive evaluations, the RPG framework has consistently outperformed its counterparts, showcasing exceptional performance in attribute binding, recognizing object relationships, and conquering the complexities of intricate prompts. The images generated by RPG are not mere approximations; they are meticulously detailed, successfully encapsulating all the elements articulated within the input text. RPG stands tall among diffusion models, distinguished by its precision, flexibility, and unparalleled generative capabilities.




Source: Marktechpost Media Inc.
Conclusion:
RPG presents a promising frontier in the advancement of text-to-image synthesis. Its innovative strategies and impressive results position it as a trailblazer in the field, setting a new standard for excellence in AI-driven image generation. As the AI landscape continues to evolve, RPG represents a beacon of progress, ushering in a new era of possibilities in the realm of text-to-image transformation.