TL;DR:
- Tel Aviv and University of Copenhagen unveil a groundbreaking ‘Plug-and-Play’ approach for fine-tuning Text-to-Image Diffusion Models.
- Current models struggle with lexical ambiguity and intricate details in input text descriptions, often misrepresenting intended image content.
- Traditional methods involve pre-trained classifiers, but they require classifiers capable of handling real and noisy data.
- This new method refines token representation without model tuning on labeled images, guided by a pre-trained classifier through an iterative process.
- It introduces ‘gradient skipping’ for optimization, significantly improving image generation speed.
- Market implications: This innovation streamlines AI image generation, making it more accessible and efficient for various industries, from e-commerce to content creation.
Main AI News:
In the realm of AI research, a groundbreaking innovation has emerged from the collaborative efforts of Tel Aviv and the University of Copenhagen. Their cutting-edge solution introduces a ‘Plug-and-Play’ approach, revolutionizing the fine-tuning of Text-to-Image Diffusion Models, all while utilizing a discriminative signal.
Text-to-image diffusion models have long dazzled us with their ability to craft a diverse array of high-quality images, driven by textual descriptions. Yet, these models grapple with challenges when the input text is riddled with lexical ambiguity or intricate details. This predicament often results in a misrepresentation of the intended image content, where an innocuous “iron” for clothing might be misconstrued as the “elemental” metal.
To address these limitations, previous approaches have harnessed the power of pre-trained classifiers to shepherd the denoising process. One such method involves harmonizing the score estimate of a diffusion model with the gradient of a pre-trained classifier’s log probability. In simpler terms, this technique amalgamates insights from both the diffusion model and the pre-trained classifier, ensuring that the generated images align with the classifier’s judgment of the image’s intended representation. However, a crucial caveat persists: this method hinges on a classifier’s ability to navigate real, noisy data.
Alternatively, other strategies have conditioned the diffusion process using class labels derived from specific datasets. While undeniably effective, this approach still falls short of the full expressive potential inherent in models trained on extensive collections of image-text pairs culled from the vast expanse of the internet.
A different path forward involves the fine-tuning of a diffusion model or select input tokens, leveraging a small dataset linked to a particular concept or label. Yet, this approach brings its own set of limitations, including the sluggish training process for new concepts, potential shifts in image distribution, and a rather limited diversity gleaned from a small pool of images.
Enter a pioneering solution that adeptly circumvents these issues, delivering a more precise representation of desired classes, resolving lexical quandaries, and enhancing the portrayal of intricate details. All of this is achieved without sacrificing the expressive prowess of the original pretrained diffusion model or being hamstrung by the aforementioned drawbacks. The method’s essence is beautifully encapsulated in the accompanying figure.
This novel approach eschews the traditional routes of guiding the diffusion process or overhauling the entire model. Instead, it concentrates its efforts on updating the representation of a solitary token dedicated to each class of interest. Crucially, this update process does not involve a full-blown model tuning exercise on labeled images.
The method achieves its goal by honing the token representation for a specific target class through an iterative dance of creating new images with a higher class probability, all under the discerning eye of a pre-trained classifier. Feedback from this classifier serves as the guiding star in shaping the evolution of the designated class token with each iteration. An ingenious optimization technique, aptly named ‘gradient skipping,’ comes into play, with the gradient being selectively propagated solely through the final stage of the diffusion process. The optimized token then seamlessly integrates into the conditioning text input, breathing life into images using the original diffusion model.
The architects of this method tout several distinct advantages. It demands nothing more than a pre-trained classifier, obviating the need for a classifier specially trained on noisy data, setting it apart from other class conditional techniques. Furthermore, it excels in speed, ushering in immediate improvements to generated images once a class token has undergone its transformative training journey, a stark departure from more time-consuming methods.
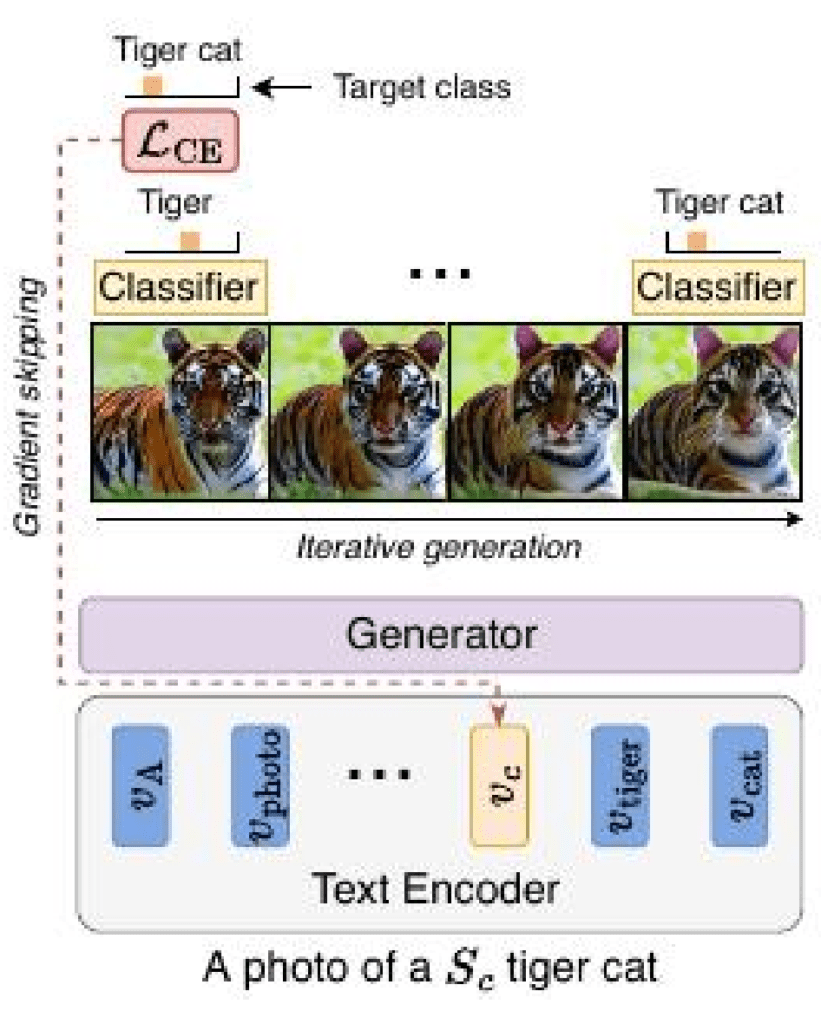
Source: Marktechpost Media Inc.
Conclusion:
This groundbreaking ‘Plug-and-Play’ approach for Text-to-Image Diffusion Models represents a significant leap forward in AI research. It addresses longstanding challenges in generating accurate images from text descriptions, offering an efficient and effective solution. For the market, this innovation promises improved image generation capabilities, which can revolutionize industries relying on AI-generated visuals, from e-commerce to entertainment and beyond.