TL;DR:
- Researchers at UNC-Chapel Hill have developed CREMA, a cutting-edge framework for multimodal video reasoning.
- CREMA efficiently integrates diverse data types such as visual frames, audio, and 3D point clouds to enhance AI’s understanding of complex scenarios.
- Traditional approaches to multimodal learning often face challenges in adaptability and computational intensity, which CREMA aims to address.
- CREMA employs a modular and efficient system, leveraging parameter-efficient modules and a query transformer architecture.
- Rigorous validation shows CREMA’s superior performance compared to existing models, with fewer trainable parameters.
Main AI News:
In the realm of artificial intelligence, the integration of multimodal inputs for video reasoning poses both a challenge and an opportunity. Researchers are increasingly directing their efforts toward harnessing a spectrum of data types – spanning visual frames, audio segments, and intricate 3D point clouds – to enhance AI’s comprehension of the world. This pursuit seeks to replicate and transcend human sensory fusion, empowering machines to decipher intricate environments and scenarios with unparalleled clarity.
Central to this endeavor lies the task of efficiently amalgamating these diverse modalities. Traditional methodologies have often faltered, either due to a lack of adaptability to new data types or the demand for prohibitively extensive computational resources. Thus, the quest persists for a solution that not only embraces the diversity of sensory inputs but also does so with agility and scalability.
While current multimodal learning methodologies exhibit promise, they are hindered by their computational intensity and rigidity. These systems typically mandate substantial parameter adjustments or bespoke modules for each new modality, rendering the incorporation of fresh data types cumbersome and resource-intensive. Such constraints impede the adaptability and scalability of AI systems in navigating the complexity of real-world inputs.
Enter a pioneering framework conceived by researchers at UNC-Chapel Hill, poised to redefine how AI systems tackle multimodal inputs for video reasoning. This groundbreaking approach introduces a modular and efficient system for fusing disparate modalities, including optical flow, 3D point clouds, and audio, devoid of extensive parameter alterations or custom modules for individual data types. At its nucleus, CREMA employs a query transformer architecture to seamlessly integrate diverse sensory inputs, ushering in a more nuanced and comprehensive understanding of complex scenarios by AI.
Noteworthy for its efficiency and adaptability, CREMA leverages a suite of parameter-efficient modules to map diverse modality features onto a unified embedding space, facilitating seamless integration sans the need for an overhaul of the underlying model architecture. This strategy conserves computational resources while ensuring the framework’s readiness to accommodate emerging modalities.
Rigorous validation of CREMA’s performance across various benchmarks underscores its superiority or parity with existing multimodal learning models, achieved with a fraction of the trainable parameters. This efficiency does not compromise effectiveness; CREMA adeptly balances the inclusion of novel modalities, ensuring each contributes meaningfully to the reasoning process, devoid of redundant or extraneous information.
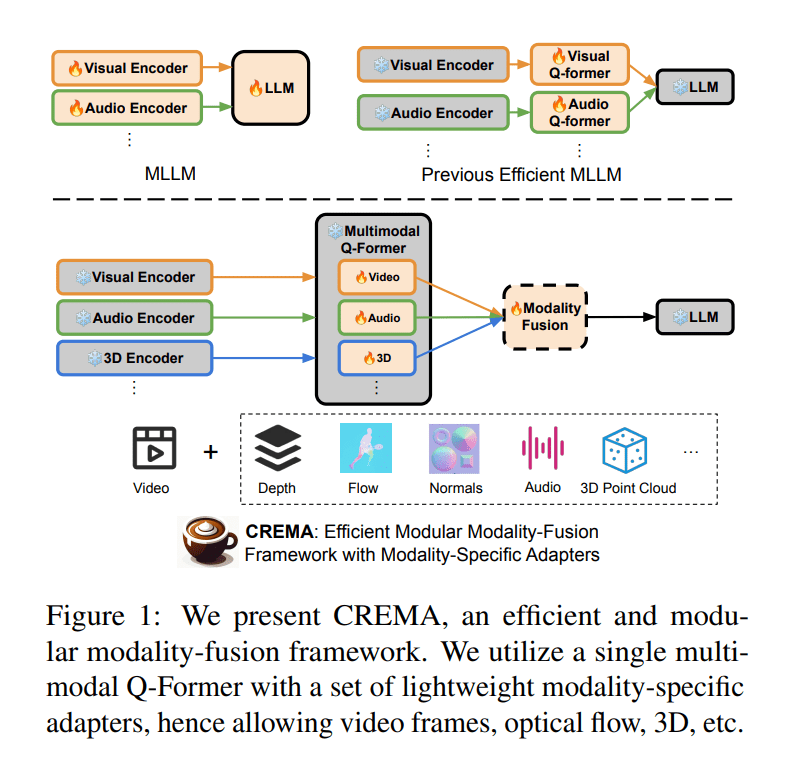
Source: Marktechpost Media Inc.
Conclusion:
The introduction of the CREMA framework by UNC-Chapel Hill signifies a significant leap forward in the field of multimodal video reasoning. By efficiently integrating diverse sensory inputs through a modular approach, CREMA not only addresses existing challenges but also sets a new standard for efficiency and adaptability. Its proven superior performance with fewer parameters underscores its potential to reshape the market landscape, offering businesses an opportunity to leverage advanced AI capabilities for enhanced video understanding and interpretation.