- Generative AI evaluation lacks reliable metrics for assessing model performance comprehensively.
- GenAI-Arena, pioneered by researchers from the University of Waterloo, introduces a dynamic platform driven by community voting to rank generative models.
- The platform supports various tasks including text-to-image generation, image editing, and text-to-video generation, with a public voting system ensuring transparency.
- GenAI-Arena has collected over 6000 votes across multiple tasks, constructing leaderboards to identify state-of-the-art models.
- Top-ranking models include Playground V2.5 and V2 for image generation, and T2VTurbo for text-to-video tasks.
Main AI News:
The realm of Generative AI has been witnessing an unprecedented surge, particularly in domains like image and video generation, fueled by cutting-edge algorithms, innovative architectures, and vast datasets. However, this rapid expansion has underscored a crucial void: the absence of reliable evaluation metrics. Existing automated evaluation methods such as FID, CLIP, and FVD often fall short in capturing the nuanced quality and user satisfaction associated with generative outputs. Despite the rapid advancement in image generation and manipulation technologies, navigating through the plethora of available models and assessing their performance poses a daunting challenge. While traditional metrics like PSNR, SSIM, LPIPS, and FID offer valuable insights into specific aspects of visual content generation, they often lack in providing a comprehensive evaluation of overall model performance, especially concerning subjective qualities such as aesthetics and user satisfaction.
A myriad of techniques has been proposed to evaluate the efficacy of multimodal generative models across various dimensions. For image generation, methodologies like CLIPScore gauge text-alignment, while metrics like IS, FID, PSNR, SSIM, and LPIPS measure image fidelity and perceptual similarity. Recent endeavors leverage multimodal large language models (MLLMs) as adjudicators, exemplified by T2I-CompBench utilizing miniGPT4, TIFA employing visual question answering, and VIEScore highlighting MLLMs’ potential to supplant human judges. In the realm of video generation, metrics such as FVD evaluate frame coherence and quality, while CLIPSIM harnesses image-text similarity models. Nevertheless, these automated metrics still lag behind human preferences, casting doubts on their reliability.
To address this gap, Generative AI evaluation platforms have emerged with the aim of systematically ranking models. Benchmark suites like T2ICompBench, HEIM, and ImagenHub for images, and VBench and EvalCrafter for videos, endeavor to fulfill this need. However, despite their functionality, these benchmarks rely on model-based metrics that are less dependable compared to human evaluation. Consequently, model arenas have surfaced to gather direct human preferences for ranking purposes. Yet, no existing arena singularly focuses on the evaluation of generative AI models.
Enter GenAI-Arena, a groundbreaking platform introduced by researchers from the University of Waterloo. Drawing inspiration from successful implementations in other domains, GenAI-Arena presents a dynamic and interactive arena where users can generate images, juxtapose them for comparison, and cast their votes for favored models. This platform streamlines the process of model comparison and furnishes a ranking mechanism that reflects human preferences, thereby offering a more holistic evaluation of model capabilities. Distinguished as the premier evaluation platform with comprehensive assessment capabilities spanning multiple attributes, GenAI-Arena supports a diverse array of tasks including text-to-image generation, text-guided image editing, and text-to-video generation, complemented by a public voting mechanism to ensure labeling transparency. The accumulated votes are leveraged to gauge the evaluation proficiency of MLLM evaluators. GenAI-Arena stands out for its versatility and transparency, having amassed over 6000 votes across three multimodal generative tasks and constructed leaderboards for each task, thereby identifying the cutting-edge models in the field.
GenAI-Arena facilitates tasks such as text-to-image generation, image editing, and text-to-video generation, offering features like anonymous side-by-side voting, battle playground, direct generation tab, and leaderboards. The platform standardizes model inference with fixed hyper-parameters and prompts for equitable comparison. It ensures impartial voting through anonymity, enabling users to express their preferences between anonymously generated outputs, with Elo rankings calculated accordingly. This architecture fosters a democratic and precise evaluation of model performance across multiple tasks.
As the researchers divulge their leaderboard rankings, Playground V2.5 and Playground V2 models from Playground.ai clinch the top spots for image generation with 4443 votes amassed. Following the same SDXL architecture but trained on a proprietary dataset, these models significantly outperform the 7th-ranked SDXL, underscoring the pivotal role of training data. StableCascade, leveraging an efficient cascade architecture, secures the subsequent rank, surpassing SDXL despite incurring only 10% of SD-2.1’s training cost, thereby highlighting the significance of diffusion architecture. In the realm of image editing with 1083 votes, MagicBrush, InFEdit, CosXLEdit, and InstructPix2Pix, facilitating localized editing, emerge as frontrunners, whereas older methodologies like Prompt-to-Prompt, yielding disparate images, lag behind despite yielding high-quality outputs. In text-to-video tasks with 1568 votes, T2VTurbo claims the pole position with the highest Elo score as the most efficacious model, closely pursued by StableVideoDiffusion, VideoCrafter2, AnimateDiff, alongside others like LaVie, OpenSora, and ModelScope, exhibiting diminishing performance.
In this exposé, GenAI-Arena emerges as a pioneering platform propelled by community voting, aimed at ranking generative models across text-to-image, image editing, and text-to-video tasks based on user preferences, thereby fostering transparency. Leveraging over 6000 votes garnered from February to June 2024, Elo leaderboards have been compiled, unveiling the state-of-the-art models, while concurrently illuminating potential biases. The dissemination of high-quality human preference data as GenAI-Bench exposes the inadequate correlation between existing multimodal language models and human judgments concerning generated content quality and other facets.
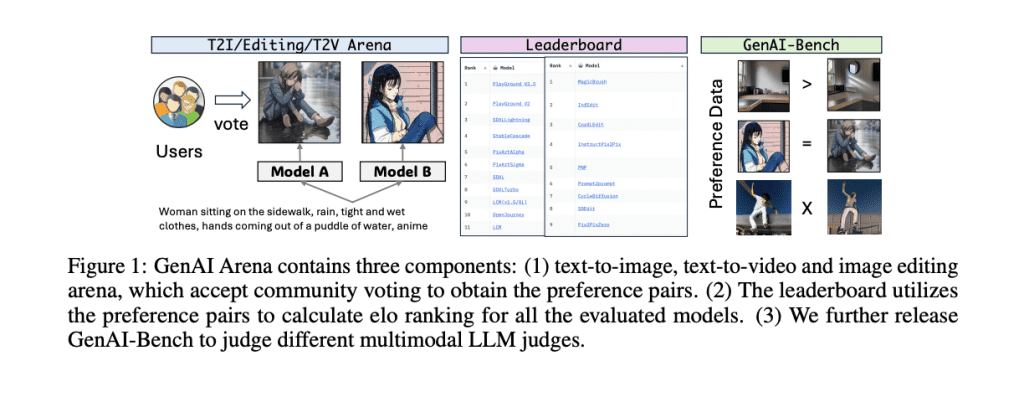
Source: Marktechpost Media Inc.
Conclusion:
GenAI-Arena’s emergence marks a significant step forward in the evaluation of Generative AI models. By leveraging community feedback and transparent ranking systems, it addresses the critical need for comprehensive assessment metrics. This platform not only identifies state-of-the-art models but also sheds light on the disparities between automated evaluations and human judgments. This highlights the growing importance of community-driven evaluation platforms in shaping the future landscape of Generative AI technologies.