TL;DR:
- MoE-LLaVA integrates visual and linguistic data for enhanced business insights.
- It strategically balances performance metrics with resource efficiency.
- The framework leverages a Mixture of Experts (MoE) approach.
- MoE-LLaVA’s architecture ensures efficient processing of image and text tokens.
- It achieves comparable performance metrics with fewer resources.
- MoE-LLaVA excels in object hallucination benchmarks, showcasing superior visual understanding capabilities.
Main AI News:
In the ever-evolving landscape of enterprise technology, the integration of cutting-edge solutions such as MoE-LLaVA marks a significant stride forward. MoE-LLaVA represents a groundbreaking advancement in the realm of large vision-language models (LVLMs), seamlessly bridging the gap between visual and linguistic data for enhanced business insights. This innovative framework, developed collaboratively by leading researchers from esteemed academic and corporate institutions, promises to revolutionize how businesses leverage AI-driven insights for strategic decision-making.
Central to the success of MoE-LLaVA is its unique approach to balancing performance metrics with resource efficiency. Traditional LVLMs often face challenges related to escalating computational demands as model complexity increases. However, MoE-LLaVA introduces a paradigm shift by strategically activating only a fraction of its parameters at any given time. This novel methodology ensures optimal performance while mitigating resource consumption, making it an ideal choice for businesses operating in resource-constrained environments.
At its core, MoE-LLaVA leverages a Mixture of Experts (MoE) approach, fine-tuned through a meticulously designed training strategy. This strategy encompasses a multi-stage process, starting with the adaptation of visual tokens to fit the language model framework. As the training progresses, MoE-LLaVA transitions into a sparse mixture of experts, strategically activating specific parameters to optimize performance. The architecture of MoE-LLaVA, comprising a vision encoder, a visual projection layer, and stacked language model blocks interspersed with MoE layers, ensures efficient processing of image and text tokens, resulting in a streamlined workflow.
One of the most remarkable aspects of MoE-LLaVA is its ability to achieve performance metrics comparable to those of larger models while utilizing significantly fewer resources. With only 3 billion sparsely activated parameters, MoE-LLaVA demonstrates exceptional efficiency without compromising on performance. Furthermore, it showcases superior capabilities in object hallucination benchmarks, surpassing larger models in visual understanding tasks. This not only highlights its efficacy but also underscores its potential to enhance the quality and reliability of AI-driven insights for businesses across various industries.
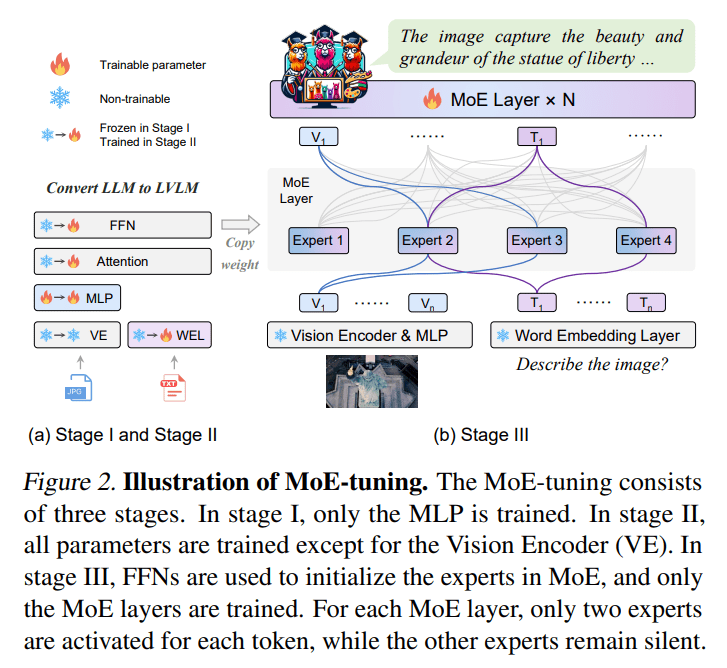
Source: Marktechpost Media Inc.
Conclusion:
The emergence of MoE-LLaVA signifies a pivotal moment in the market for AI-driven business intelligence solutions. Its ability to achieve comparable performance metrics with significantly fewer resources heralds a new era of efficiency and effectiveness in leveraging artificial intelligence for strategic decision-making. By streamlining the processing of visual and textual data while maintaining superior performance, MoE-LLaVA sets a new standard for LVLMs, offering businesses a transformative tool to gain actionable insights and maintain a competitive edge in today’s dynamic landscape.