TL;DR:
- ViGoR, a novel AI framework, enhances visual grounding for LVLMs through fine-grained reward modeling.
- Developed by researchers from UT Austin and AWS AI, ViGoR integrates human evaluations and automated methods for optimization.
- The framework strategically refines pre-trained LVLMs like LLaVA, significantly improving their visual grounding efficiency.
- ViGoR’s innovative use of automated techniques reduces the need for additional human labor, enhancing LVLM performance.
- Superior to existing baseline models, ViGoR’s efficacy is validated across multiple benchmarks, including a challenging dataset.
- The team plans to release a human annotation dataset to support further research.
Main AI News:
In a groundbreaking collaboration between UT Austin and AWS AI, a pioneering AI framework dubbed ViGoR (Visual Grounding Through Fine-Grained Reward Modeling) has emerged, heralding a new era in the fusion of natural language understanding and image perception. LVLMs (Large Vision Language Models) have long captivated researchers with their extraordinary reasoning capabilities. However, the challenge of precisely aligning textual output with visual inputs has persisted, resulting in inaccuracies like phantom scene elements and misinterpretations of object attributes.
Enter ViGoR, a visionary solution crafted by researchers from The University of Texas at Austin and AWS AI. This innovative framework propels the visual grounding of LVLMs beyond conventional baselines through fine-grained reward modeling, leveraging both human evaluations and automated techniques for optimization. ViGoR’s methodology stands out for its strategic refinement of pre-trained LVLMs, notably exemplified by its enhancement of LLaVA. Through a meticulous process involving human annotators and automated mechanisms, ViGoR refines LVLMs’ visual grounding capabilities with remarkable efficiency, requiring only a modest dataset of 16,000 samples.
What sets ViGoR apart is its ingenious integration of automated methods to construct the reward model, eliminating the need for additional human labor while significantly bolstering the efficacy of LVLMs in visual grounding. The harmonious interplay between human-evaluated and automated reward models forms the cornerstone of ViGoR’s holistic approach, resulting in a substantial improvement in LVLM performance.
ViGoR’s superiority is evident in its outperforming of existing baseline models across various benchmarks, including a specially curated, challenging dataset designed to rigorously test LVLMs’ visual grounding capabilities. To facilitate further research, the team behind ViGoR plans to release their meticulously annotated human dataset, comprising approximately 16,000 image-text pairs with nuanced evaluations. With ViGoR paving the way for enhanced visual grounding in LVLMs, the possibilities for AI-driven applications in diverse domains are boundless.
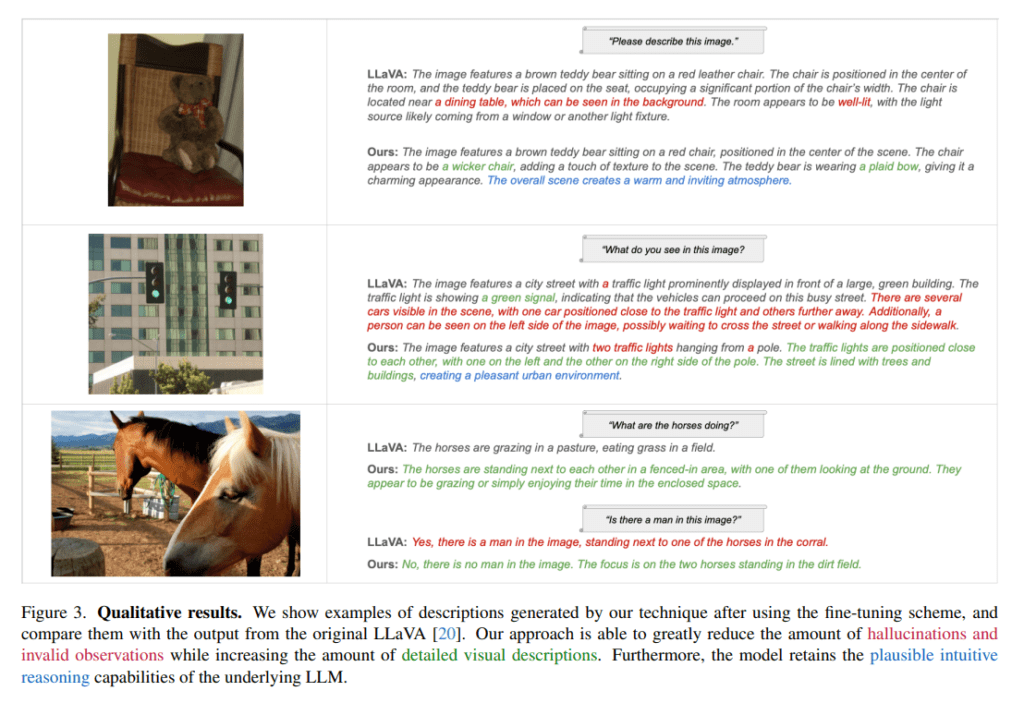
Source: Marktechpost Media Inc.
Conclusion:
ViGoR’s introduction marks a significant advancement in the fusion of natural language understanding and image perception. Its ability to enhance the visual grounding capabilities of LVLMs has profound implications for various industries, unlocking new possibilities for AI-driven applications in fields such as computer vision, robotics, and virtual reality. As ViGoR continues to evolve, businesses leveraging AI technologies stand to gain a competitive edge by harnessing more accurate and efficient visual understanding systems.