TL;DR:
- Bayesian inference and machine learning techniques revolutionize the study of astrochemistry.
- Conflicting values of binding energies pose challenges in understanding grain-surface chemistry.
- The MOPED compression algorithm aids in prioritizing species for future detection.
- Interpretable machine learning reveals non-linear relationships between binding energies and species abundances.
Main AI News:
Astrochemistry, the captivating realm that unravels the chemical composition of the universe, holds an abundance of secrets yet to be fully deciphered. To embark on a profound exploration of this domain, a comprehensive comprehension of the fundamental parameters governing grain-surface chemistry is indispensable. Among these paramount parameters, the binding energies of various species play a pivotal role.
Ironically, the scientific community finds itself entangled in a web of conflicting values for these critical binding energies, leading to perplexity and uncertainty. Resolving this enigma requires a fresh perspective and innovative methodologies. In this groundbreaking endeavor, a statistical and machine learning approach emerges as a guiding light, offering a novel pathway to estimate these elusive values.
Admittedly, the scarcity of available data poses a substantial challenge in accurately determining these binding energies. To overcome this hurdle, a visionary solution comes in the form of the Massive Optimized Parameter Estimation and Data (MOPED) compression algorithm. By leveraging this cutting-edge tool, the research community gains invaluable assistance in identifying which species should be given precedence for future detections. This strategic prioritization enables more precise confinement of the binding energy values, elevating the accuracy of astrochemical investigations.
However, the quest for understanding the intricate relationship between binding energies and the ultimate abundances of specific species of interest demands an even deeper comprehension. Enter an interpretable machine learning approach, a breakthrough methodology that unravels the complex, non-linear interplay between these vital elements. Through the lens of this advanced technique, scientists can unlock new insights and unravel hidden patterns, fostering a deeper understanding of astrochemical processes.
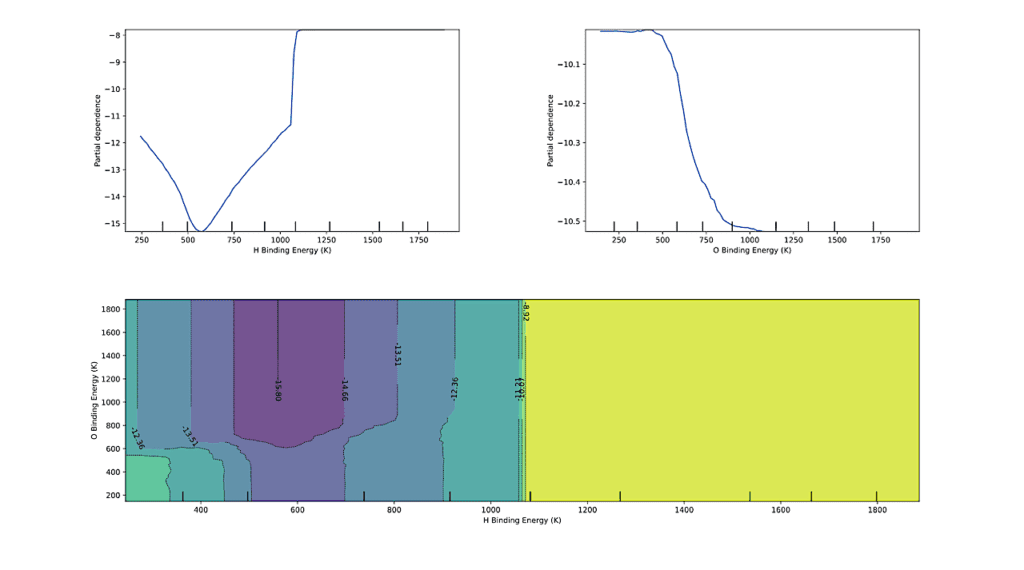
Top: A plot of the 1-D partial dependence plots of the binding energies of H and O for CO. The partial dependence represents the expected value of the log-abundance of CO as a function of the variable in features, marginalised over all other features. Bottom: A 2-D partial dependence plot for the binding energies of H and O. Yellow represents the region with the highest abundance of CO. Source: astro-ph.GA
Conclusion:
The application of statistical and machine learning methodologies in astrochemistry brings about significant advancements and opportunities in the market. By employing Bayesian inference and machine learning techniques, researchers can enhance their understanding of grain-surface chemistry, leading to breakthrough discoveries and insights. The development of the MOPED compression algorithm provides valuable guidance for future detections, enabling efficient allocation of resources and prioritization of species.
Moreover, the utilization of interpretable machine learning approaches allows for a deeper comprehension of the complex relationships between binding energies and species abundances. This newfound knowledge opens doors to innovation and the potential development of novel technologies, materials, and processes with applications in various industries, including pharmaceuticals, materials science, and environmental sciences. Businesses operating in these sectors can leverage these advancements to gain a competitive edge, optimize their processes, and drive innovation in their respective markets.