- NREL introduces Sup3rCC, a generative machine learning model for simulating future energy-climate impacts.
- Sup3rCC offers high-resolution climate datasets to understand climate change effects on renewable energy sources.
- Developed through collaboration between energy analysts and computational scientists, Sup3rCC bridges the gap between energy and climate research.
- Powered by generative adversarial networks (GANs), Sup3rCC enhances data resolution and accelerates modeling processes.
- Sup3rCC’s datasets complement NREL’s modeling tools, aiding in comprehensive studies on renewable energy potential.
Main AI News:
In an era where global transitions to renewable energy sources like wind and solar are reshaping energy landscapes, understanding the intricate relationship between energy systems and climate dynamics becomes paramount. With the increasing frequency of extreme weather events due to climate change, there’s a pressing need for accurate projections of future energy variables to inform planning and operational strategies.
Grant Buster, a data scientist at the U.S. Department of Energy’s National Renewable Energy Laboratory (NREL), emphasizes the necessity of anticipating how climate shifts will affect renewable resources like wind and solar. He underscores the importance of bridging the gap between energy needs and climate realities.
Enter Sup3rCC (pronounced “super-c-c”), a groundbreaking generative machine learning model developed by a team at NREL. Recently featured in Nature Energy journal, Sup3rCC offers open-source, high-resolution climate datasets that are vital for assessing the impacts of climate change on energy systems.
According to Dan Bilello, director of the Strategic Energy Analysis Center at NREL, Sup3rCC heralds a new era in energy system planning by providing essential climate data for informed decision-making. This tool, he notes, fills a crucial void in understanding the energy-climate nexus.
Sup3rCC tackles the longstanding disconnect between energy and climate research by integrating multi-decadal climate changes into energy system models. Through collaboration between energy analysts and computational scientists, this model promises to advance the emerging field of energy-climate research.
Powered by generative adversarial networks (GANs), Sup3rCC leverages artificial intelligence to enhance spatial and temporal resolutions of climate data. Ryan King, a computational researcher at NREL and co-developer of Sup3rCC, emphasizes its ability to generate detailed climate variables crucial for assessing renewable energy potential and system operations.
This innovative approach not only boosts data resolution but also accelerates the modeling process, enabling swift analysis of multiple climate scenarios. King highlights Sup3rCC’s contribution to understanding future renewable energy generation and its implications for power system operations.
Sup3rCC’s datasets complement NREL’s suite of modeling tools, facilitating comprehensive studies on renewable energy potential. Accessible via Amazon Web Services, these datasets empower researchers to explore various climate scenarios and their impact on renewable energy deployment.
The success of Sup3rCC underscores NREL’s commitment to collaborative research and data-driven innovation. By synergizing analysis and computational expertise, NREL aims to advance research across diverse energy domains and pave the way for a sustainable energy future.
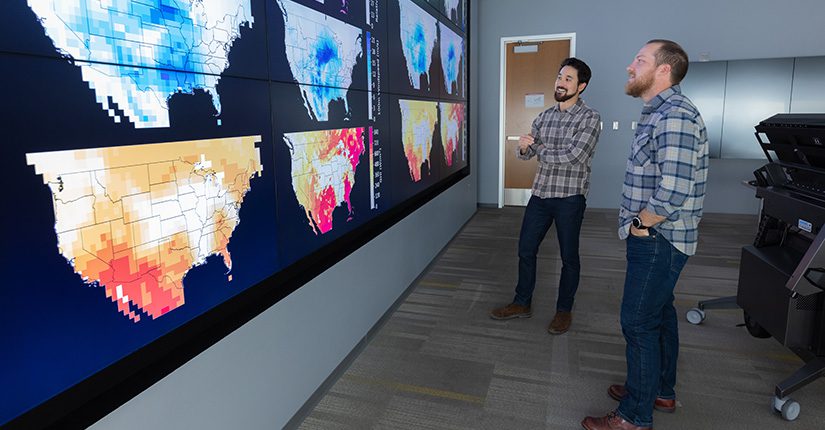
Sup3rCC is changing the way we conduct integrated energy system planning. Source: photo by Joe DelNero, NREL
Conclusion:
NREL’s Sup3rCC model marks a significant advancement in understanding the complex interplay between climate change and renewable energy systems. Its ability to provide high-resolution climate data and streamline modeling processes empowers decision-makers to plan and operate energy systems more effectively in a changing climate landscape. This innovation underscores the growing importance of data-driven approaches in navigating the transition towards a sustainable energy future.