TL;DR:
- Researchers at Hosei University study the effectiveness of cameras and AI in analyzing traffic volume.
- Challenges in accurately measuring traffic volume were identified, but three solutions were proposed.
- Published research paper in the Journal of Digital Life, a leading multidisciplinary online journal.
- Road Traffic Census comprises a general traffic volume survey and a survey of vehicle origins and destinations.
- Ministry of Land, Infrastructure, Transport and Tourism plans to replace manual observation with AI in the general traffic volume survey.
- Concerns about AI taking over part-time jobs, but the survey reveals the need for human surveyors in rural areas.
- AI system faces accuracy issues at night and during traffic congestion.
- Estimations made for traffic volume during unreliable AI hours based on past surveys.
- Efforts are underway to develop systems enhancing efficiency in traffic surveys.
- Automation of cross-sectional and intersection traffic volume measurements.
- Solutions were developed to address inaccuracies in vehicle counts and misclassification of vehicle types.
- Conversion of nighttime images to “daytime” images improves accuracy during nighttime hours.
- The research concludes that the employed technologies hold promise for traffic surveys.
Main AI News:
In a groundbreaking study led by Professor Ryuichi Imai from Hosei University, the effectiveness of cameras and artificial intelligence (AI) in analyzing traffic volume has been thoroughly explored. Specifically, the study delved into the potential of this technology in the Road Traffic Census, a crucial survey conducted by the Ministry of Land, Infrastructure, Transport and Tourism. Although the initial findings highlighted challenges in accurately measuring traffic volume, the research team unveiled three innovative solutions that paved the way for practical implementation.
The team’s insightful research paper, recently published in the esteemed Journal of Digital Life, offers a multidisciplinary perspective on this cutting-edge endeavor. As a peer-reviewed and open-access online journal based in Japan, it serves as a testament to the significance and relevance of the study’s findings.
The Road Traffic Census, conducted every five years, encompasses two key components: a comprehensive traffic volume survey that examines road usage and a survey that investigates the origins and destinations of vehicles. Notably, the Ministry’s 2021 plan to replace manual observation in the general traffic volume survey with AI has sparked a significant interest. However, concerns regarding the potential displacement of part-time surveyors by AI have surfaced.
Surprisingly, a survey conducted by the National Institute for Land and Infrastructure Management (NILIM) in 2021 revealed an unexpected need for human surveyors in rural areas. It appears that despite the advancements in AI technology, a complete takeover by AI is yet to occur. The survey highlighted the importance of human expertise, particularly in rural environments, where the distinct nuances of traffic patterns require skilled observation.
One of the key challenges discovered in the implementation of AI systems was their decreased accuracy during nighttime hours and traffic congestion. Notably, between 8 pm and 10 pm, the AI system’s accuracy plummeted to a mere 20-30% compared to visual observation. To address this limitation, the 2021 survey made estimations for traffic volume during unreliable AI hours. Leveraging past surveys conducted under similar traffic conditions, as well as locations with available nighttime monitoring, researchers sought to fill the gap in AI’s reliability.
With the decline in the available workforce posing a significant challenge, the recruitment of surveyors is expected to become increasingly arduous. Consequently, efforts are underway to develop systems that can enhance efficiency in traffic surveys. Spearheading this initiative, Professor Imai and his research group focused on automating two critical measurements: “cross-sectional traffic volume” and “intersection traffic volume.”
The first measurement, “cross-sectional traffic volume,” entails summarizing the number, speed, and type of inbound and outbound vehicles. The second, “intersection traffic volume,” scrutinizes the movement of vehicles at intersections, considering whether they turn right, left, or proceed straight ahead. Through their innovative approach, the research group successfully devised solutions to three key challenges: inaccurate vehicle counts, misrecognition of vehicle types, and diminished accuracy during nighttime hours.
Accurate vehicle counts have long been a challenge for existing AI methods. These methods often fail to detect vehicles in the proximity of the survey point, leading to inaccuracies. To address this issue, the research group harnessed the power of YOLOv3 (You Only Look Once), an AI model renowned for its object-detection capabilities in video images. YOLOv3 enabled the system to identify vehicles with similar characteristics appearing in frames before and after passing the survey point. By considering the entire trajectory of a vehicle, even if it was missed at a specific “point,” it would still be accounted for along the “line” of its path.
In their quest for improved accuracy, the researchers recognized the tendency of existing methods to misclassify vehicles with similar color and shape attributes. To mitigate this challenge, they employed VGG19, an AI technology adept at image recognition, to enhance classification precision.
To ensure the accuracy of their system, the research group put it to the test using a 15-minute video capturing two one-way lanes in Osaka City. While the number of vehicles was accurately counted, the classification of vehicle types remained an issue. Sedans and trucks were classified correctly, but misclassifications occurred for vans and small trucks, which were mistakenly identified as larger vehicles.

Source: Japan Forward
Addressing this classification challenge, the research group conducted interviews with ten seasoned surveyors who possessed years, or even decades, of experience in traffic volume surveys. Through these interviews, the researchers discovered that vehicle classification relied not only on shape but also on additional features such as the front-to-side ratio, the proportion of the windshield and other parts, and the color of the license plate. Armed with this valuable insight, the researchers devised a method that utilized specific vehicle parts as unique identifiers.
To further refine the classification system, computer-generated graphics of various vehicles were created. By assigning distinct colors to different parts of the vehicles, such as red for the front, green for the top side, and blue for the windshield, the researchers trained the AI system to recognize the characteristics of each vehicle type. After rigorous testing, the AI system successfully classified small sedans, vans, buses, and trucks while maintaining accurate counts within a “10% error margin,” a crucial benchmark for practical application.
Addressing the challenges encountered during nighttime hours, the research group explored the transformative potential of AI technology in converting nighttime images into visually comparable “daytime” images. In conjunction with the previously mentioned vehicle classification technique that employed vehicle parts as identifiers, this method proved to be effective. The results demonstrated that the accuracy of vehicle detection in the converted nighttime images, spanning from 7 pm to 7 am, was comparable to that achieved during daytime surveys at the same survey point.
Nighttime conditions presented unique challenges due to low luminous intensity, measuring at approximately 10 lux, akin to the level of illumination experienced during a movie theater intermission. Without image conversion, the accuracy of the AI system was significantly reduced, particularly for larger vehicles. However, the conversion technology triumphantly addressed this limitation, markedly improving accuracy in such scenarios. Nevertheless, the team noted that the classification of vehicle types posed challenges when vehicles were black, leading to occasional misclassifications.
Drawing from the wealth of insights gained through their experiments, the research team confidently concluded that the three technologies employed in their study hold tremendous promise for traffic surveys. Undoubtedly, their findings will drive further exploration and refinement of vehicle classification accuracy while inspiring the pursuit of diverse applications for this groundbreaking technology. As the research group continues to build upon existing studies, they remain steadfast in their commitment to enhancing the accuracy of vehicle classification and revolutionizing the world of traffic analysis.
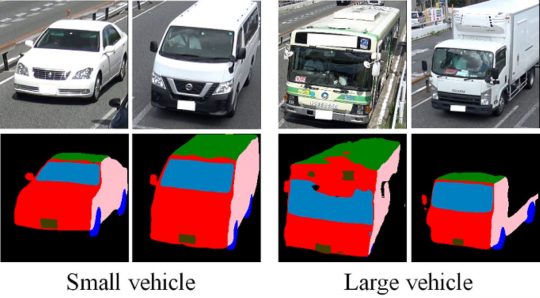
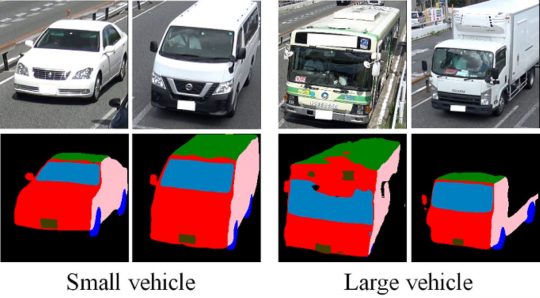
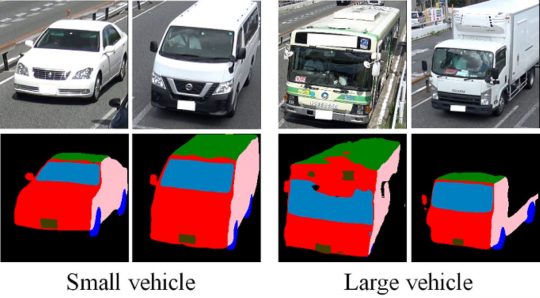
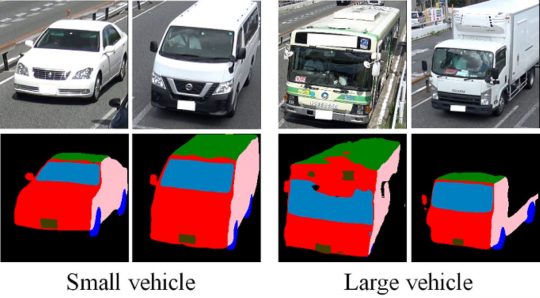
Source: Japan Forward
Conclusion:
The integration of cameras and AI in traffic volume analysis presents a paradigm shift in the market. Despite challenges in accurately measuring traffic volume, the proposed solutions offer potential for practical implementation. The recognition of the continued need for human surveyors in rural areas ensures a balance between AI and human expertise. As this technology advances, businesses operating in the traffic analysis market should consider investing in AI-based solutions to enhance efficiency and accuracy, capitalizing on the transformative potential it brings to the industry.